Text2Time: Transformer-based Article Time Period Prediction
2023 IEEE 6th International Conference on Pattern Recognition and Artificial Intelligence (PRAI)(2023)
摘要
The prediction of the publication period of textual documents, such as news articles, represents a significant and relatively understudied problem within the realm of natural language processing. Determining the year in which a news article was published holds relevance in various domains, including historical research, sentiment analysis, and media monitoring. In this research, our focus is on investigating the prediction of publication periods specifically for news articles, leveraging their textual content. To tackle this challenge, we curated an extensive labeled dataset consisting of over 350,000 news articles published by The New York Times over a span of six decades. This dataset forms the foundation of our investigation. Our approach involves utilizing a pretrained BERT model that has been fine-tuned for the task of text classification, specifically tailored for time period prediction. The performance of our model surpasses our initial expectations, demonstrating impressive results in accurately classifying news articles into their respective publication decades. Through rigorous evaluation, our model outperforms the baseline model for this relatively unexplored task of predicting time periods based on textual content. This research sheds light on the potential for effectively predicting the publication periods of news articles and presents promising outcomes achieved by leveraging a pretrained BERT model fine-tuned for time period classification. The results obtained contribute to the advancement of this underexplored task, demonstrating the viability and accuracy of time prediction from textual data.
更多查看译文
关键词
LLM,Bert,NLP,article time,NYTimes
AI 理解论文
溯源树
样例
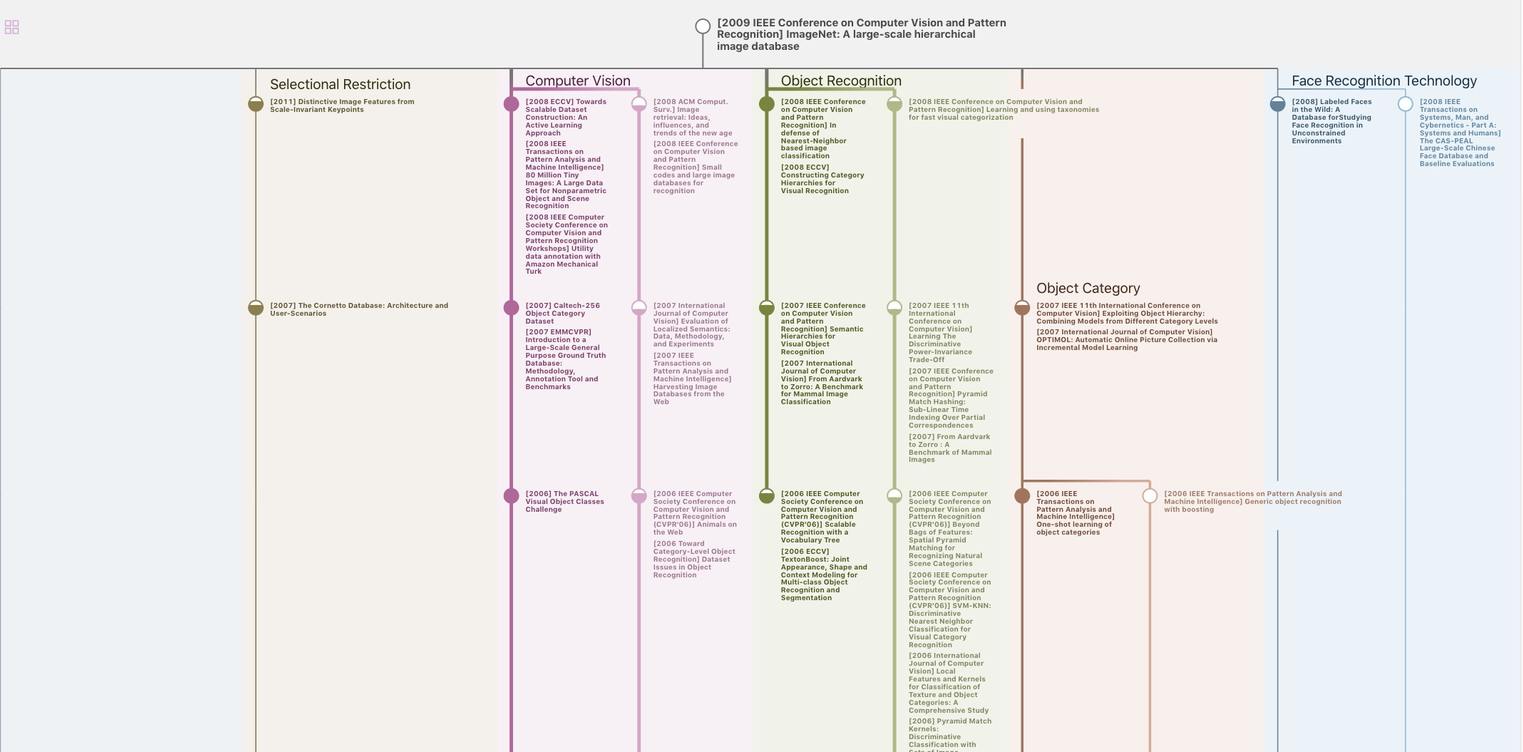
生成溯源树,研究论文发展脉络
Chat Paper
正在生成论文摘要