Improved YOLOv5 Detection Algorithm of Road Disease Image
2023 IEEE 6th International Conference on Pattern Recognition and Artificial Intelligence (PRAI)(2023)
摘要
With the rapid development of China's urban roads, the traffic road network is increasingly improved, and the road mileage has reached a new height. Faced with such a large-scale traffic road network, fast and efficient pavement disease detection becomes an urgent problem to be solved. The existing models are weak in detecting defects related to the proximal and distal ends of the actual roads, and the detection performance is insufficient. Therefore, the YOLOv5 network model containing RepLKDeXt module, SOCA module, and Mixup hybrid data enhancement is modified for the YOLOv5 model, which is designed to use RepLKDeXt module to replace some of the C3 layers of the trunk and neck to enrich the feature extraction capability and improve the feature expression capability of the network, use Mixup data enhancement at the input side technique to improve the learning ability of the network to extract features from complex images and further enhance the detection accuracy of the network model. Experiments were conducted on the Japanese road disease dataset, and the experimental results showed that the improved YOLOv5 model reduced the number of parameters by about 10% and improved the detection accuracy by 3.1%.
更多查看译文
AI 理解论文
溯源树
样例
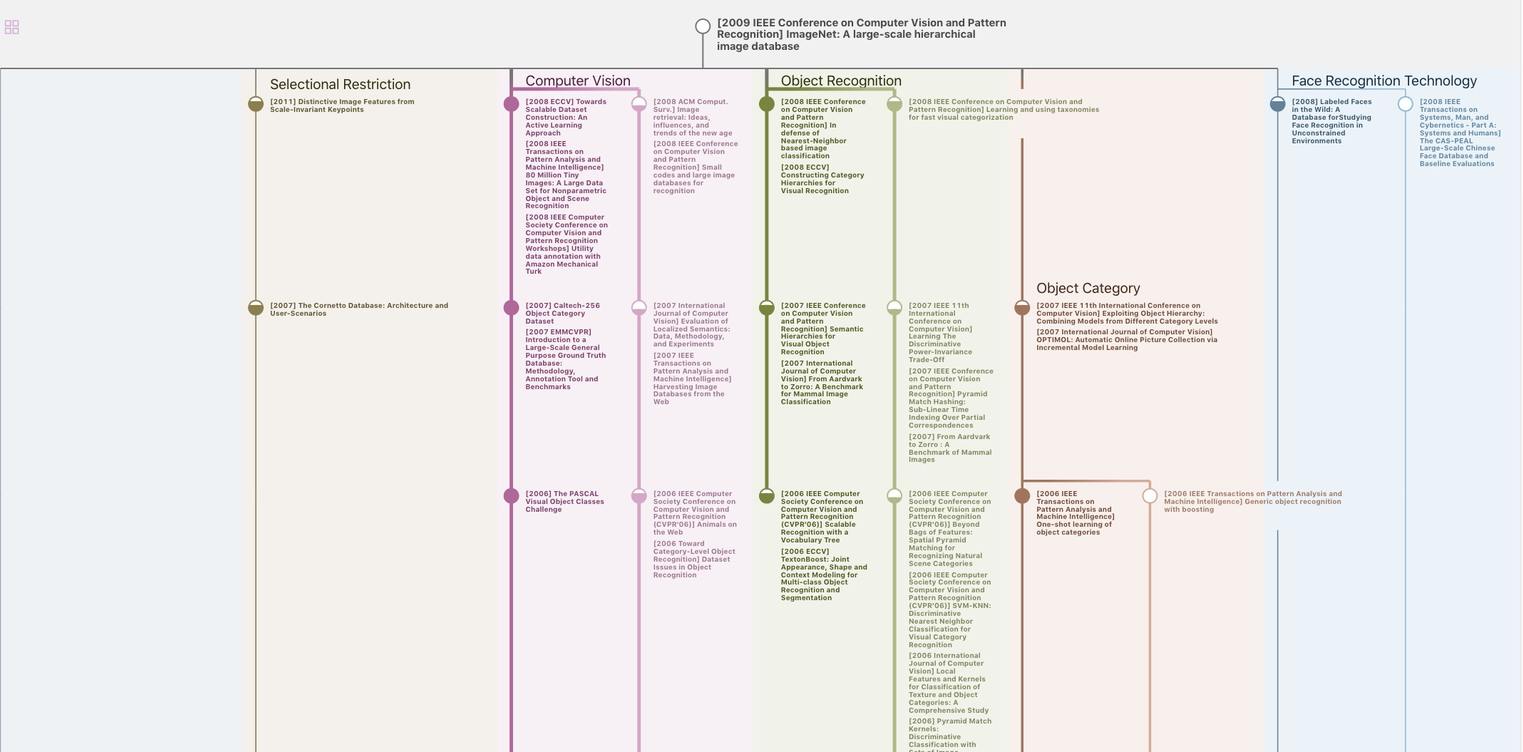
生成溯源树,研究论文发展脉络
Chat Paper
正在生成论文摘要