Linearized Alternating Direction Method of Multipliers for Elastic-Net Support Vector Machines
Pattern Recognition(2024)
摘要
In many high-dimensional datasets, the phenomenon that features are relevant often occurs. Elastic-net regularization is widely used in support vector machines (SVMs) because it can automatically perform feature selection and encourage highly correlated features to be selected or removed together. Recently, some effective algorithms have been proposed to solve the elastic-net SVMs with different convex loss functions, such as hinge, squared hinge, huberized hinge, pinball and huberized pinball. In this paper, we develop a linearized alternating direction method of multipliers (LADMM) algorithm to solve above elastic-net SVMs. In addition, our algorithm can be applied to solve some new elastic-net SVMs such as elastic-net least squares SVM. Compared with some existing algorithms, our algorithm has comparable or better performances in terms of computational cost and accuracy. Under mild conditions, we prove the convergence and derive convergence rate of our algorithm. Furthermore, numerical experiments on synthetic and real datasets demonstrate the feasibility and validity of the proposed algorithm.
更多查看译文
关键词
Convex optimization,Linearized ADMM,Elastic-net,Support vector machines
AI 理解论文
溯源树
样例
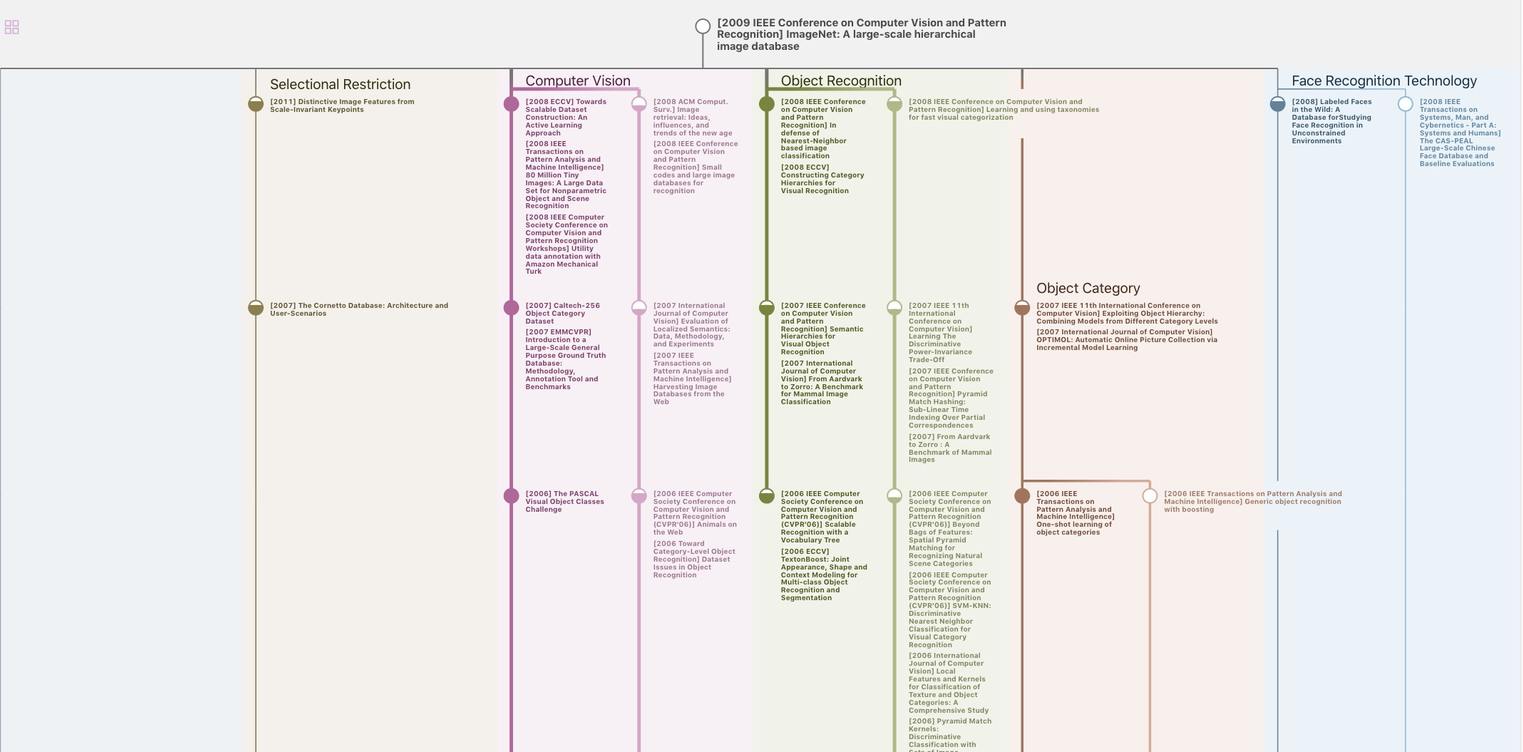
生成溯源树,研究论文发展脉络
Chat Paper
正在生成论文摘要