Multiple Mask and Boundary Scoring R-CNN with Cgan Data Augmentation for Bladder Tumor Segmentation in WLC Videos
Artificial intelligence in medicine(2024)
摘要
Automatic diagnosis systems capable of handling multiple pathologies are essential in clinical practice. This study focuses on enhancing precise lesion localization, classification and delineation in transurethral resection of bladder tumor (TURBT) to reduce cancer recurrence. Despite deep learning models success, medical applications face challenges like small and limited datasets and poor image characterization, including the absence lack of color/texture modeling. To address these issues, three solutions are proposed: (1) an improved textureconstrained version of the pix2pixHD cGAN for data augmentation, addressing the tradeoff of generating high-quality images with enough stochasticity using the Fre ' chet Inception Distance (FID) measure. (2) Introducing the Multiple Mask and Boundary Scoring R-CNN (MM&BS R-CNN), a new mask sub-net scheme where multiple masks are generated from the different levels of the mask sub-net pipeline, improving segmentation accuracy by including a new scoring module to refine object boundaries. (3) A novel accelerated training strategy based on the SGD optimizer with the second momentum. Experimental results show significant mAP improvements: the data generation scheme improves by more than 12 %; MM&BS R-CNN proposed architecture is responsible for an improvement of about 1.25 %, and the training algorithm based on the second-order momentum increases mAP by 2-3 %. The simultaneous use of all three proposals improved the state-of-the-art mAP by 17.44 %.
更多查看译文
关键词
Multi-pathology detection,Lesion localization,Texture-constrained GAN
AI 理解论文
溯源树
样例
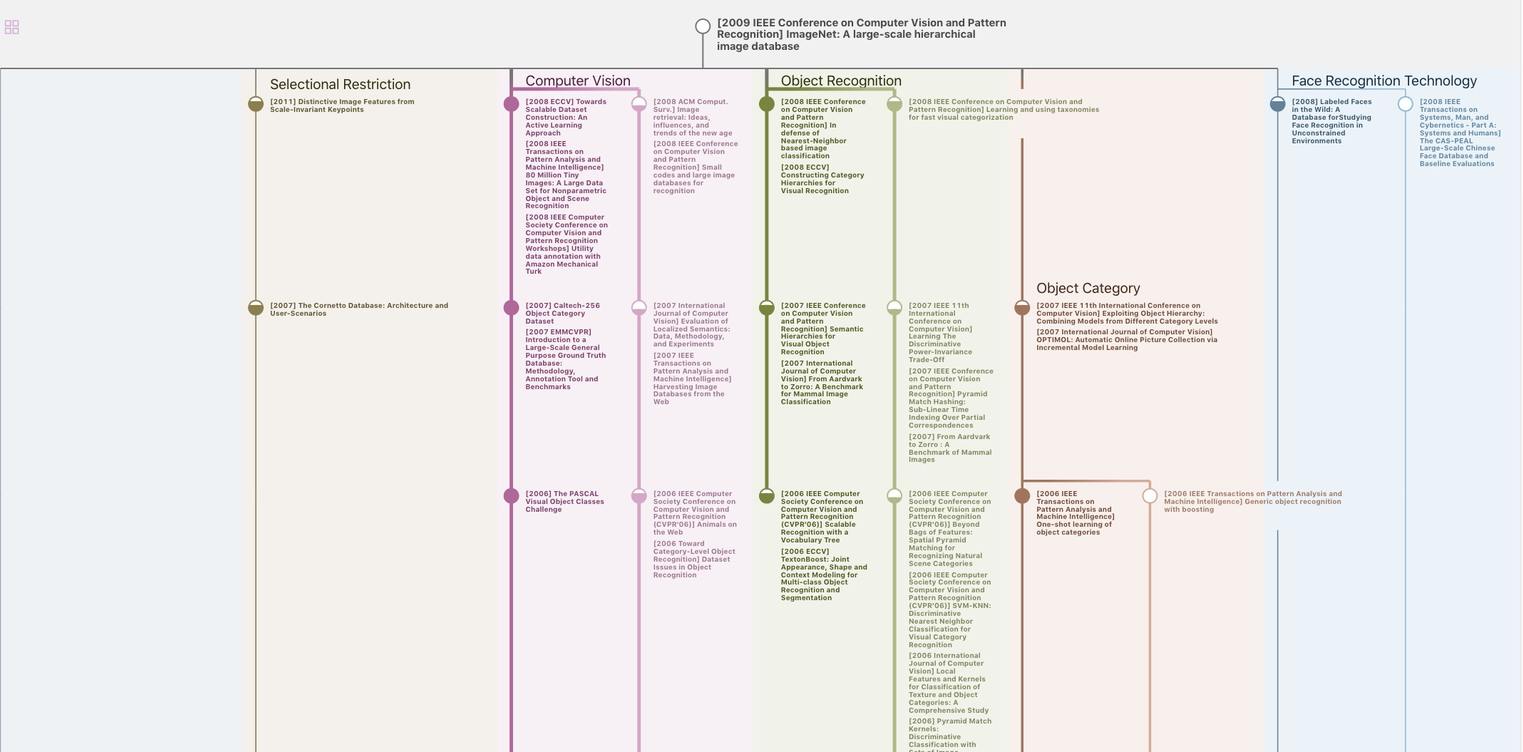
生成溯源树,研究论文发展脉络
Chat Paper
正在生成论文摘要