Enhancing Individual Tree Mortality Mapping: the Impact of Models, Data Modalities, and Classification Taxonomy
Remote sensing of environment(2024)
摘要
Tree mortality is rapidly increasing as a result of more frequent and extensive droughts and forest fires across the globe. The increasing pace and scale of disturbance and resulting mortality necessitates the study of tree mor-tality at the scale at which it occurs (individual trees) to develop effective management strategies. Achieving this objective requires high-resolution aerial or satellite remote sensing data supported by field validation and effi-cient modeling. However, the optimal remote sensing data modalities and models for mapping overstory tree mortality remain uncertain and there is a lack of benchmark datasets for reproducible research, comparison, and model improvement. In this research, we propose a framework to generate tree mortality maps at the scale of individual trees and a labeled benchmark dataset using open-source high-resolution remote sensing data. Our benchmark dataset consists of over 15,000 image crops that have been manually labeled via multi-annotator majority for live, dead, mixed, and other classes in National Ecological Observatory Network (NEON) RGB, NEON hyperspectral, and National Agriculture Imagery Program (NAIP) RGBNIR imagery. Specifically, we present a multi-class classification framework that categorizes lidar-derived tree segments as live, dead, mixed, or other. We compared the performance of different machine learning models, remote sensing data modalities and their associated spatial resolution, spectral depth, and orthorectification using our benchmark dataset. Among various models, we found Convolutional Neural Network (CNN) models outperformed all other models with the highest accuracies and F1 scores across all data modalities. Similarly, among remote sensing data modalities, NEON RGB data at 0.1 m resolution performed best due to its high spatial resolution and high quality orthorectification. The overall accuracy of 87.27% with a macro F1 score of 0.76 was achieved using the RGB data with the CNN model. Our framework can be applied to any forest ecosystem in which lidar and raster imagery are available. We applied the framework using the best performing model and data modality to produce an individual tree mortality map over the Teakettle Experimental Forest in the southern Sierra Nevada. The framework and high-resolution mortality map can be valuable resources for forest managers and provide an invaluable basis for studying potential mortality drivers of dominant trees, forest inventory, selective logging, fire disturbance, and succession modeling.
更多查看译文
关键词
Deep learning,Drought mortality,Hyperspectral imagery,Lidar,Convolutional Neural Network
AI 理解论文
溯源树
样例
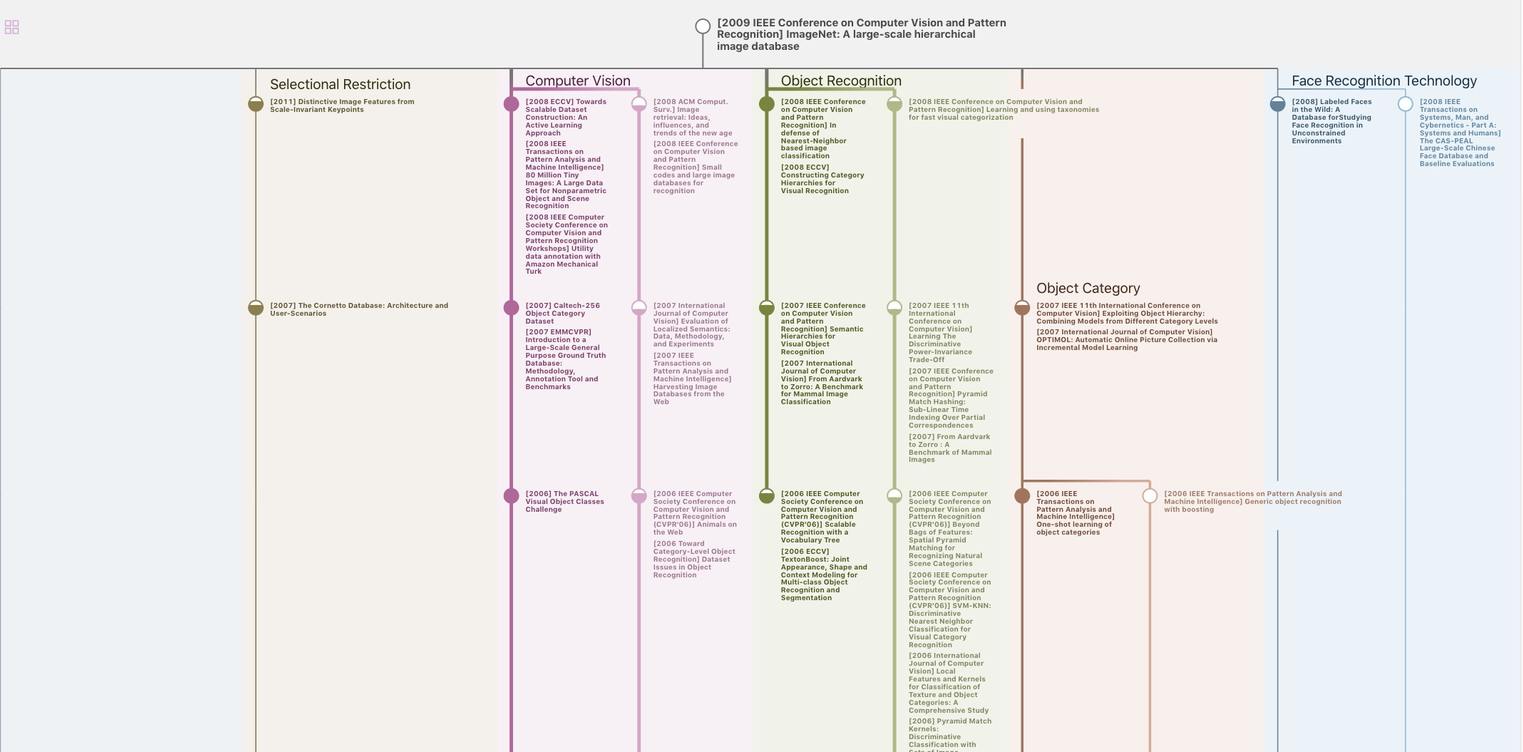
生成溯源树,研究论文发展脉络
Chat Paper
正在生成论文摘要