A Structural Consensus Representation Learning Framework for Multi-View Clustering
KNOWLEDGE-BASED SYSTEMS(2024)
Abstract
Learning a structural consensus representation is crucial for various multi-view tasks, such as multi-view clustering, multi-view classification, etc. However, this task is challenging due to the inconsistent structure problem caused by the view-level bias of multi-view data. To address this issue, we propose a structural consensus representation learning (SCRL) framework, which contains two cascading representation training processes, to learn and refine structural consensus representation. A Consensual Joint Multi-AutoEncoder is developed which estimates a consensual structure shared among all views and learns each view representation guided by the consensual structure in a unique process. By applying EM (Expectation–Maximization)-style optimization, the view representations and the consensual structure are optimized iteratively. Then, we devise a Hybrid Contrastive Refining Net, which contains two contrastive refining components to fine-tune the learnt representations by further eliminating inconsistent view representations within the same view and from different views. The proposed SCRL framework is able to debias the learning of view representations and provide structural consensus representations for multi-view clustering. Extensive experiments and analysis on several real datasets show the effectiveness of our proposed SCRL framework.
MoreTranslated text
Key words
Multi-view data,Structural consensus representation,Consensual joint learning,Hybrid contrastive refining
求助PDF
上传PDF
View via Publisher
AI Read Science
Must-Reading Tree
Example
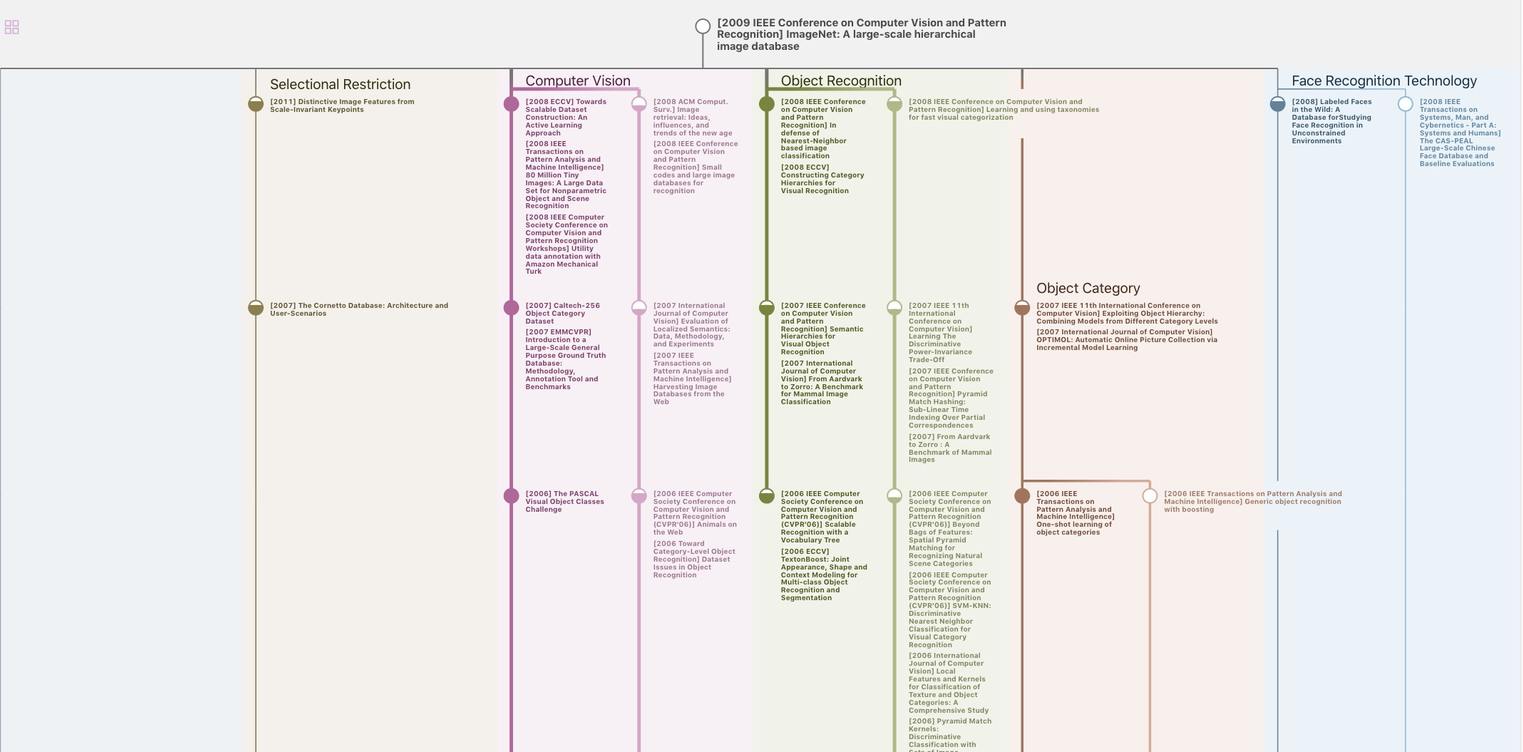
Generate MRT to find the research sequence of this paper
Data Disclaimer
The page data are from open Internet sources, cooperative publishers and automatic analysis results through AI technology. We do not make any commitments and guarantees for the validity, accuracy, correctness, reliability, completeness and timeliness of the page data. If you have any questions, please contact us by email: report@aminer.cn
Chat Paper