Neural Multi-hop Logical Query Answering with Concept-Level Answers
SEMANTIC WEB, ISWC 2023, PART I(2023)
摘要
Neural multi-hop logical query answering (LQA) is a fundamental task to explore relational data such as knowledge graphs, which aims at answering multi-hop queries with logical operations based on distributed representations of queries and answers. Although previous LQA methods can give specific instance-level answers, they are not able to provide descriptive concept-level answers, where each concept is a description of a set of instances. Concept-level answers are more comprehensible to users and are of great usefulness in the field of applied ontology. In this work, we formulate the problem of LQA with concept-level answers (LQAC), solving which needs to address challenges in incorporating, representing, and operating on concepts. We propose an original solution for LQAC. Firstly, we incorporate description logic-based ontological axioms to provide the source of concepts. Then, we represent concepts and queries as fuzzy sets, i.e., sets whose elements have degrees of membership, to bridge concepts and queries with instances. Moreover, we design operators involving concepts on top of fuzzy set representation of concepts and queries for optimization and inference. Extensive experimental results on three real-world datasets demonstrate the effectiveness of our method for LQAC. In particular, we show that our method is promising in discovering complex logical biomedical facts.
更多查看译文
关键词
Knowledge Representation Learning,Multi-hop Logical Query Answering,Fuzzy Logic,Neuro-symbolic Reasoning
AI 理解论文
溯源树
样例
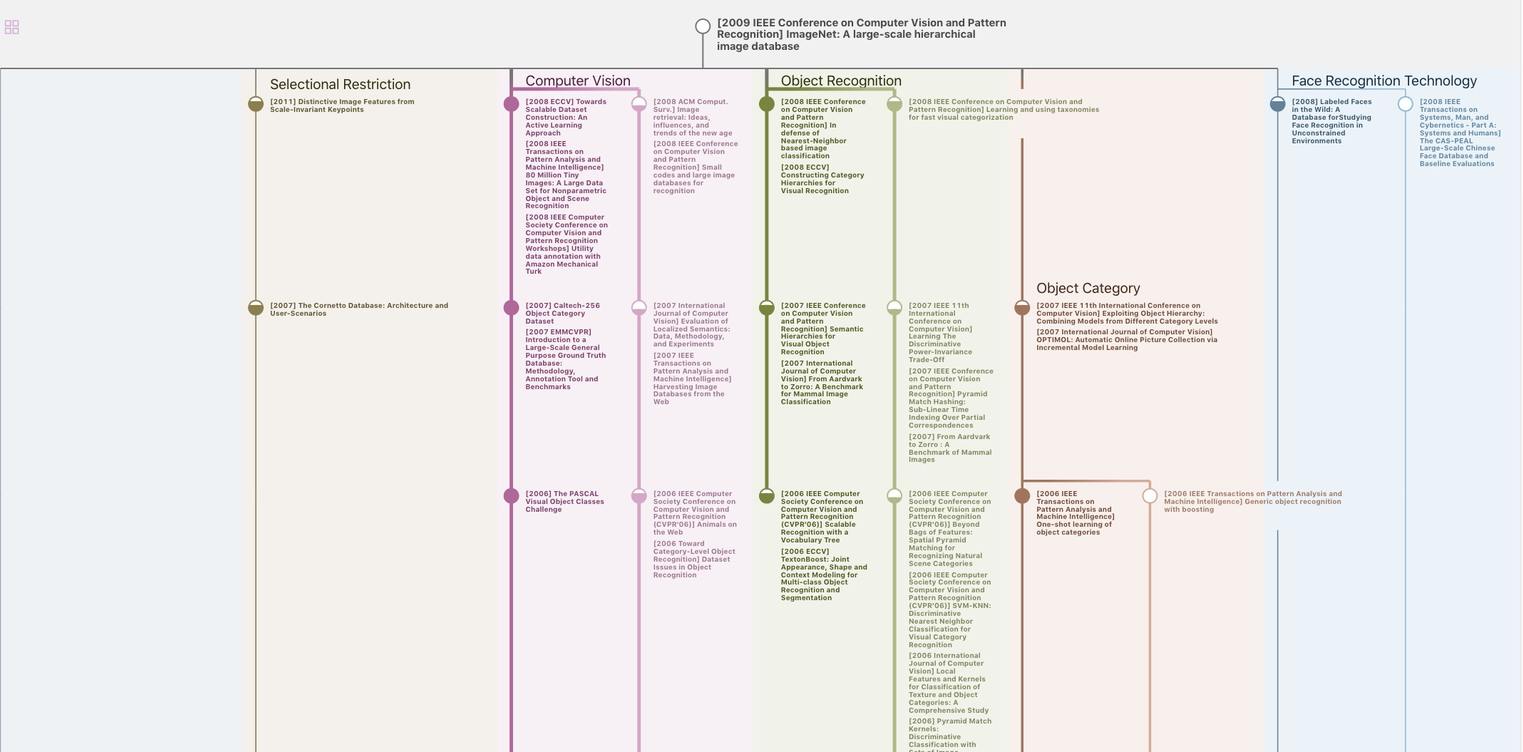
生成溯源树,研究论文发展脉络
Chat Paper
正在生成论文摘要