A Two-Stage Generative Model with CycleGAN and Joint Diffusion for MRI-based Brain Tumor Detection.
IEEE journal of biomedical and health informatics(2024)
摘要
Accuratedetection and segmentation of brain tumors is critical for medical diagnosis. However, current supervised learning methods require extensively annotated images and the state-of-the-art generative models used in unsupervised methods often have limitations in covering the whole data distribution. In this paper, we propose a novel framework Two-Stage Generative Model (TSGM) that combines Cycle Generative Adversarial Network (CycleGAN) and Variance Exploding stochastic differential equation using joint probability (VE-JP) to improve brain tumor detection and segmentation. The CycleGAN is trained on unpaired data to generate abnormal images from healthy images as data prior. Then VE-JP is implemented to reconstruct healthy images using synthetic paired abnormal images as a guide, which alters only pathological regions but not regions of healthy. Notably, our method directly learned the joint probability distribution for conditional generation. The residual between input and reconstructed images suggests the abnormalities and a thresholding method is subsequently applied to obtain segmentation results. Furthermore, the multimodal results are weighted with different weights to improve the segmentation accuracy further. We validated our method on three datasets, and compared with other unsupervised methods for anomaly detection and segmentation. The DSC score of 0.8590 in BraTs2020 dataset, 0.6226 in ITCS dataset and 0.7403 in In-house dataset show that our method achieves better segmentation performance and has better generalization.
更多查看译文
关键词
Tumors,Brain modeling,Diseases,Image segmentation,Biological system modeling,Magnetic resonance imaging,Image reconstruction,Joint diffusion model,CycleGAN synthesize,unsupervised anomaly detection,brain tumor
AI 理解论文
溯源树
样例
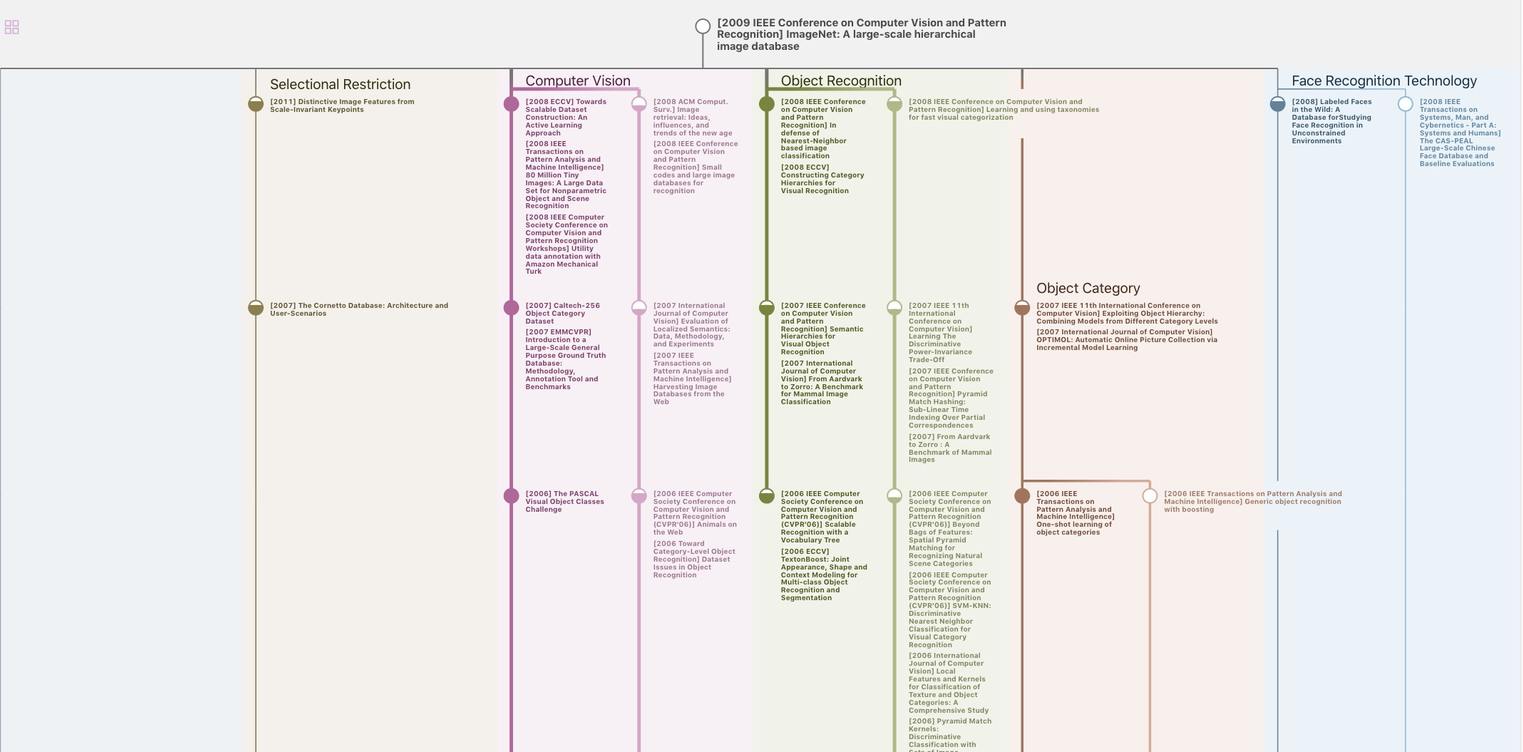
生成溯源树,研究论文发展脉络
Chat Paper
正在生成论文摘要