FedBnR: Mitigating Federated Learning Non-IID Problem by Breaking the Skewed Task and Reconstructing Representation
FUTURE GENERATION COMPUTER SYSTEMS-THE INTERNATIONAL JOURNAL OF ESCIENCE(2024)
Abstract
Federated Learning (FL), as a novel distributed machine learning paradigm, offers infinite possibilities for collaborative use of decentralized data among distributed entities. However, the potential data heterogeneity in distributed entities poses a great challenge to deploying FL for real-world practical applications. Inspired by the observed phenomenon of data heterogeneity simulation, we propose a FL framework to mitigate the non-iid problem by breaking the skewed task and reconstructing representation, called FedBnR. The basic idea of FedBnR is to break up the original skewed (unbalanced) task into multiple unskewed (balanced) subtasks and then reconstruct the representation of the original task using the unskewed subtask feature extractors. We design the FedBnR-SUR algorithm within the framework to verify its feasibility. We simulate the heterogeneous setup using the Dirichlet distribution. We conduct comparative experiments on MNIST, Cifar10, Cifar100, Cinic10, and Tiny-Imagenet. FedBnR-SUR outperforms the best baseline algorithms by about 0.96%, 1.49%, 3.53%, 1.77%, and 1.24%, respectively.
MoreTranslated text
Key words
Distributed machine learning,Federated learning,Data heterogeneity,Non-IID data,Unbalanced data,Personalized federated learning
AI Read Science
Must-Reading Tree
Example
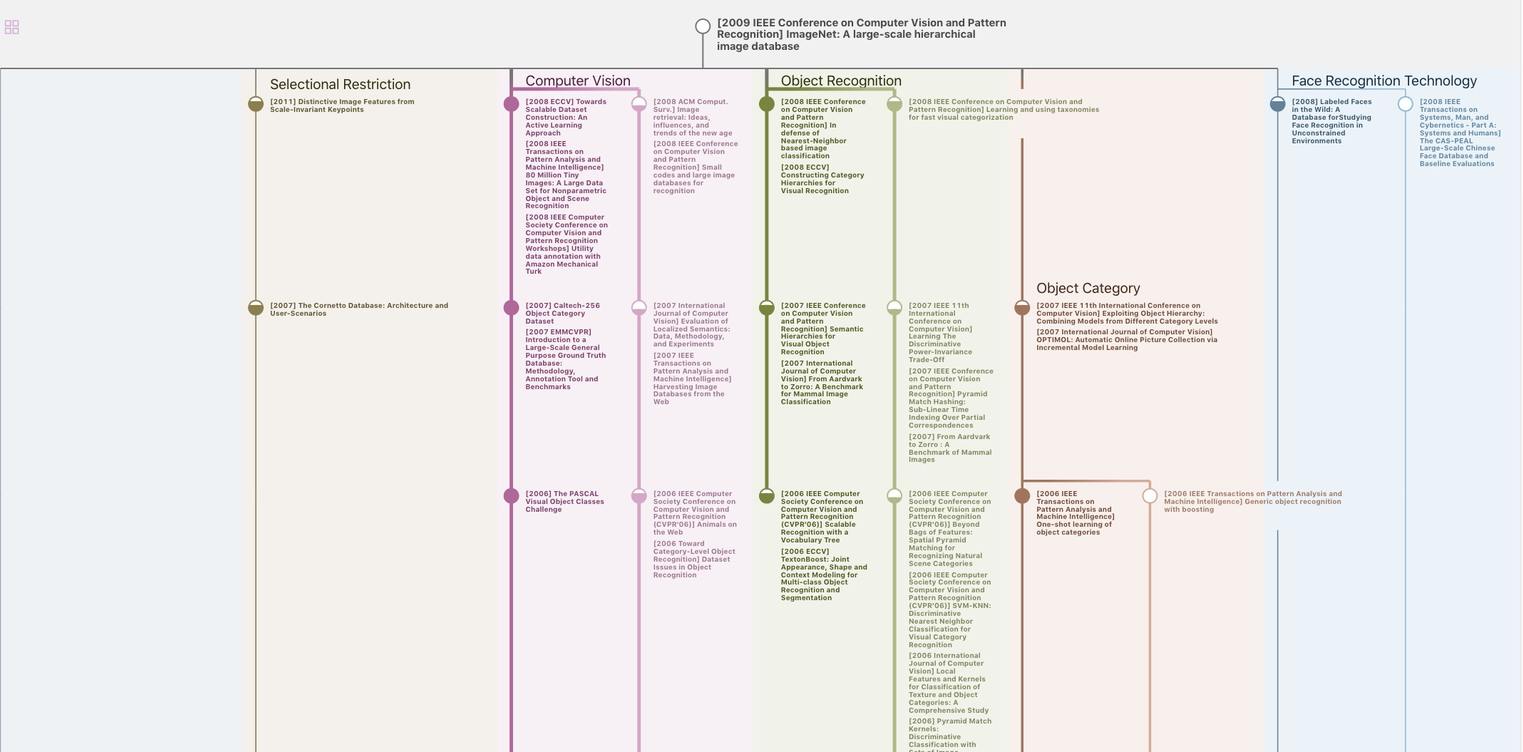
Generate MRT to find the research sequence of this paper
Chat Paper
Summary is being generated by the instructions you defined