Classification and Ensemble Machine Learning Algorithms to Predict Memory Requirements for Compute Farm Jobs
Applications of Modelling and Simulation(2023)
摘要
Tasks ranging from synthesis to regression are executed within a computational farm environment during chip design. This process is managed by a compute farm scheduler, which handles job scheduling based on the availability of such computational resources as central processing units, memory, and storage. The increasing complexity of chip design over the years, combined with a growing number of cores per chip, has resulted in memory-intensive applications often being executed as compute jobs. Jobs submitted with inaccurate resource-related requests, especially those concerning memory, can overload a compute farm and lead to wasted resources. This study addresses this issue by using a data science-driven, machine learning-based approach to predict the memory required for a compute job at the time of its submission. Improving the accuracy of such predictions can significantly reduce the overall wait times of jobs and enable efficient use of the compute farm to reduce the overall cost because fewer machines are required to complete a set of jobs. We explored the use of the K-nearest neighbor, random forest, and ensemble methods to this end. The proposed approach yielded an accuracy of 80% in experiments, where this demonstrates the success of predicting the memory-related requirements of compute jobs across a diverse suite of applications used in the process of chip design.
更多查看译文
关键词
chip design,compute farm scheduler,machine learning algorithms,memory prediction,resource management
AI 理解论文
溯源树
样例
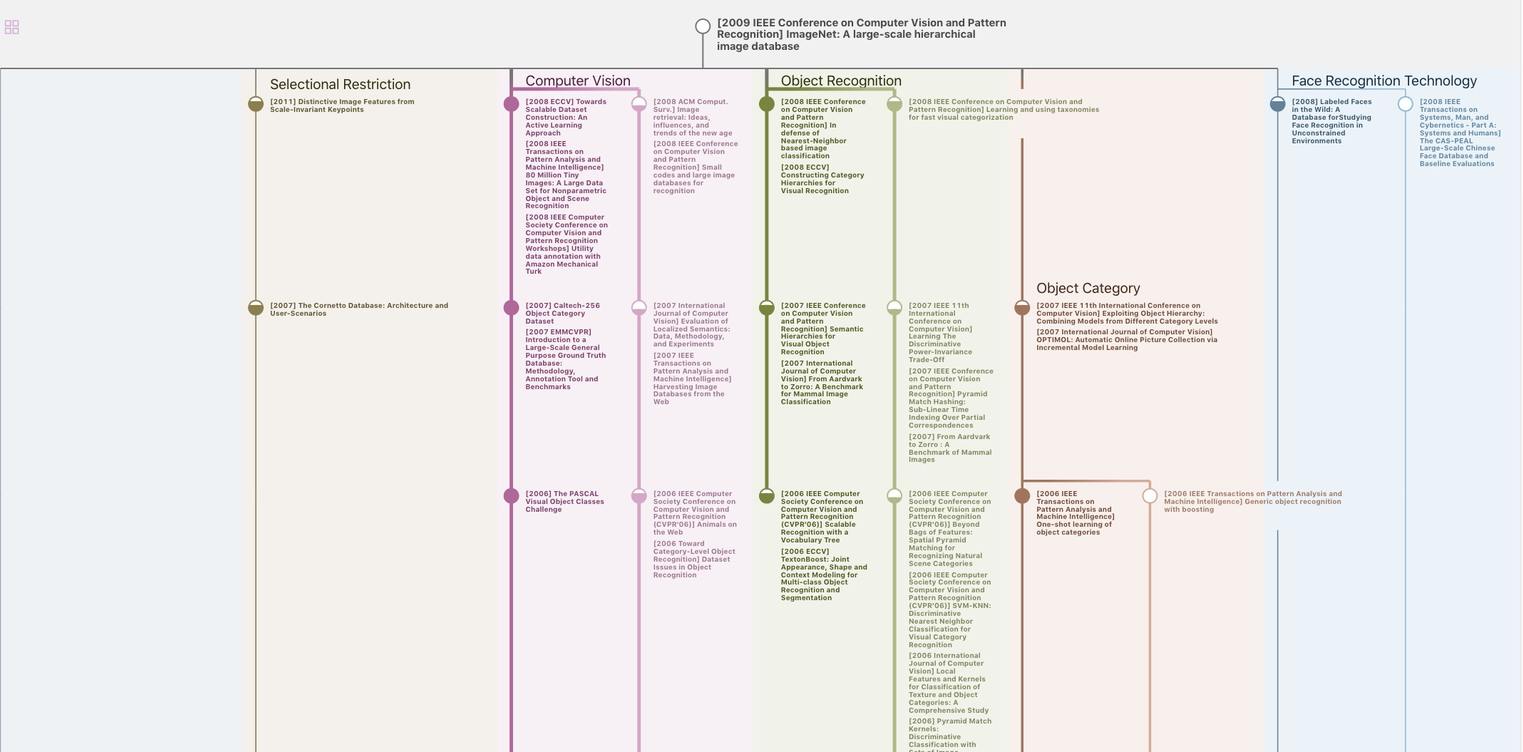
生成溯源树,研究论文发展脉络
Chat Paper
正在生成论文摘要