A Knowledge-Based Data-Driven (KBDD) Framework for All-Day Identification of Cloud Types Using Satellite Remote Sensing
Remote sensing of environment(2024)
摘要
Cloud types, as a type of meteorological data, are of particular significance for evaluating changes in rainfall, heatwaves, water resources, floods and droughts, food security and vegetation cover, as well as land use. In order to effectively utilize high-resolution geostationary observations, a knowledge-based data-driven (KBDD) framework for all-day identification of cloud types based on spectral information from Himawari-8/9 satellite sensors is designed. And a novel, simple and efficient network, named CldNet, is proposed. The accuracy of the proposed model CldNet reaches 80.89 ± 2.18% in cloud type classification, which marks an improvement of 32%, 46%, 22%, 2%, and 39% compared to other widely used semantic segmentation networks such as SegNet, PSPNet, DeepLabV3+, UNet, and ResUnet, respectively. With the assistance of auxiliary information (e.g., satellite zenith/azimuth angle, solar zenith/azimuth angle), the accuracy of CldNet-W using visible and near-infrared bands and CldNet-O not using visible and near-infrared bands on the test dataset is 82.23 ± 2.14% and 72.88 ± 1.42%, respectively. Meanwhile, the total parameters of CldNet are only 0.46M, making it easy for edge deployment. More importantly, the trained CldNet without any fine-tuning can predict cloud types with higher spatial resolution using satellite spectral data with spatial resolution 0.02°×0.02°, which indicates that CldNet possesses a strong generalization ability. In aggregate, the KBDD framework using CldNet is a highly effective cloud-type identification system capable of providing a high-fidelity, all-day, spatiotemporal cloud-type database for many climate assessment fields.
更多查看译文
关键词
Cloud types,All-day identification,Knowledge-based data-driven framework,Edge deployment,Generalization ability
AI 理解论文
溯源树
样例
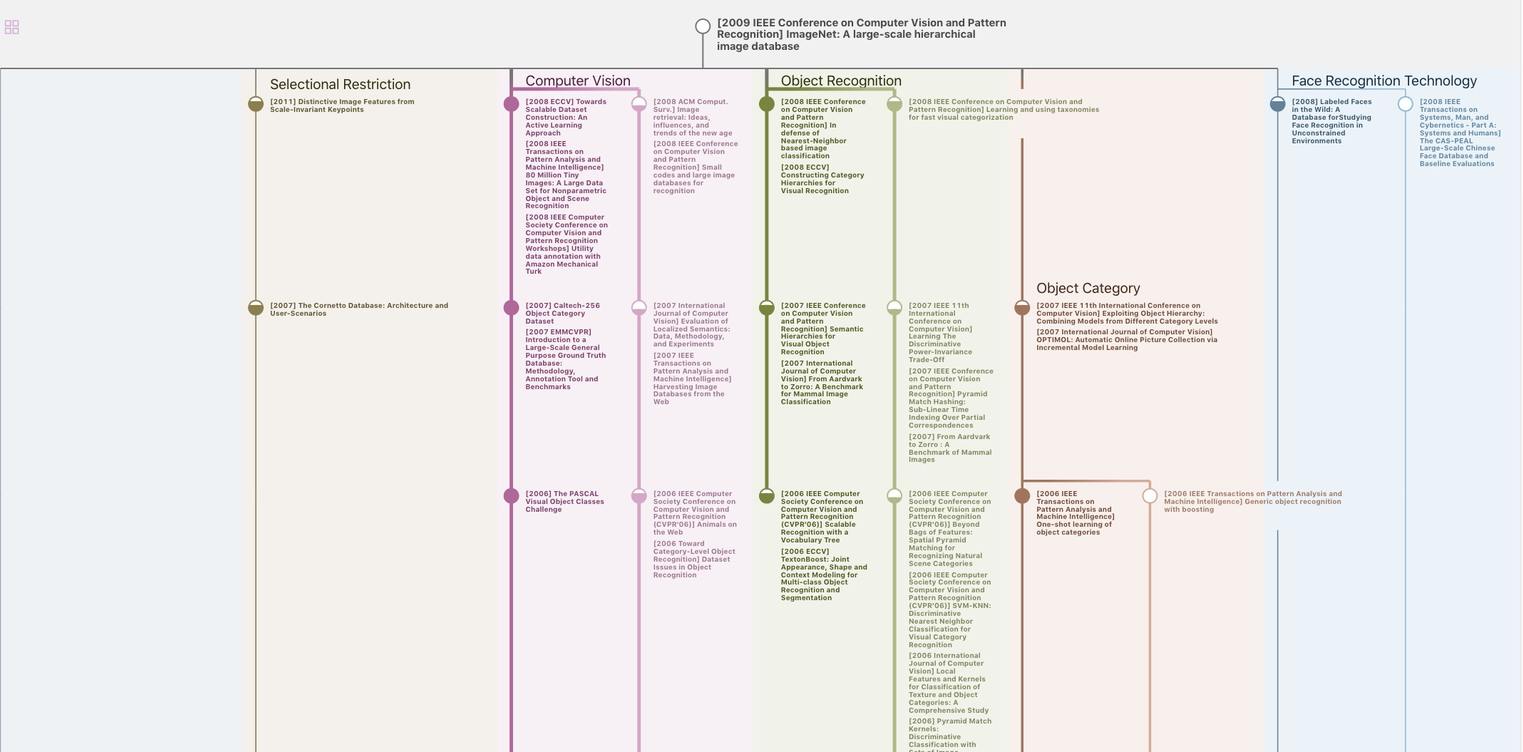
生成溯源树,研究论文发展脉络
Chat Paper
正在生成论文摘要