RingMo-SAM: A Foundation Model for Segment Anything in Multimodal Remote-Sensing Images
IEEE Transactions on Geoscience and Remote Sensing(2023)
摘要
The proposal of the segment anything model (SAM) has created a new paradigm for the deep-learning-based semantic segmentation field and has shown amazing generalization performance. However, we find it may fail or perform poorly on multimodal remote-sensing scenarios, especially synthetic aperture radar (SAR) images. Besides, SAM does not provide category information for objects. In this article, we propose a foundation model for multimodal remote-sensing image segmentation called RingMo-SAM, which can not only segment anything in optical and SAR remote-sensing data, but also identify object categories. First, a large-scale dataset containing millions of segmentation instances is constructed by collecting multiple open-source datasets in this field to train the model. Then, by constructing an instance-type and terrain-type category-decoupling mask decoder (CDMDecoder), the categorywise segmentation of various objects is achieved. In addition, a prompt encoder embedded with the characteristics of multimodal remote-sensing data is designed. It not only supports multibox prompts to improve the segmentation accuracy of multiobjects in complicated remote-sensing scenes, but also supports SAR characteristics prompts to improve the segmentation performance on SAR images. Extensive experimental results on several datasets including iSAID, ISPRS Vaihingen, ISPRS Potsdam, AIR-PolSAR-Seg, and so on have demonstrated the effectiveness of our method.
更多查看译文
关键词
Remote sensing,Task analysis,Semantic segmentation,Feature extraction,Radar polarimetry,Training,Adaptation models,Multimodal remote-sensing images,prompt learning,segment anything model (SAM),semantic segmentation
AI 理解论文
溯源树
样例
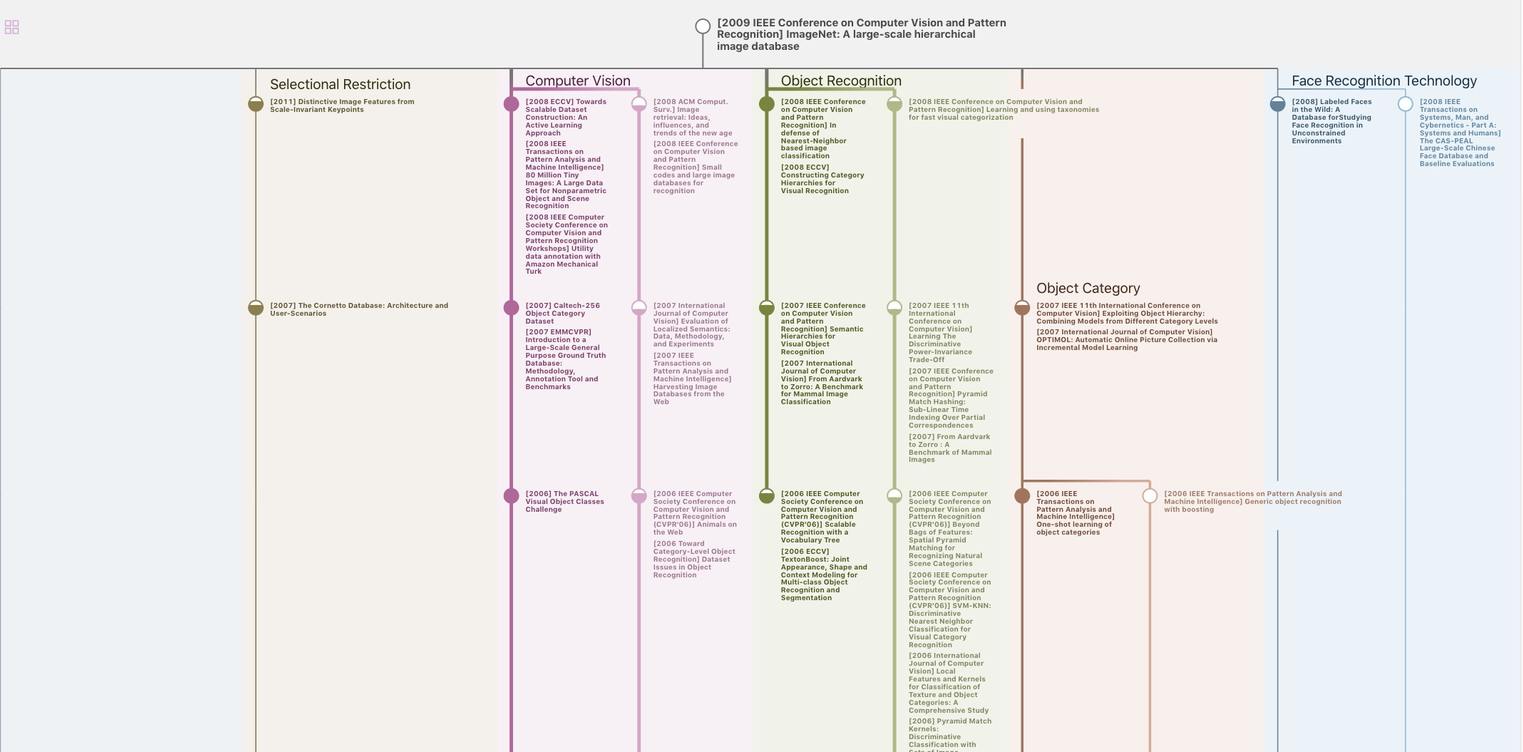
生成溯源树,研究论文发展脉络
Chat Paper
正在生成论文摘要