Malware classification using static analysis based features
2017 IEEE Symposium Series on Computational Intelligence (SSCI)(2017)
摘要
Anti-virus vendors receive hundreds of thousands of malware to be analysed each day. Some are new malware while others are variations or evolutions of existing malware. Because analyzing each malware sample by hand is impossible, automated techniques to analyse and categorize incoming samples are needed. In this work, we explore various machine learning features extracted from malware samples through static analysis for classification of malware binaries into already known malware families. We present a new feature based on control statement shingling that has a comparable accuracy to ordinary opcode n-gram based features while requiring smaller dimensions. This, in turn, results in a shorter training time.
更多查看译文
关键词
malware classification,static analysis,anti-virus vendors,malware binaries,ordinary opcode n-gram based features,machine learning features,control statement shingling
AI 理解论文
溯源树
样例
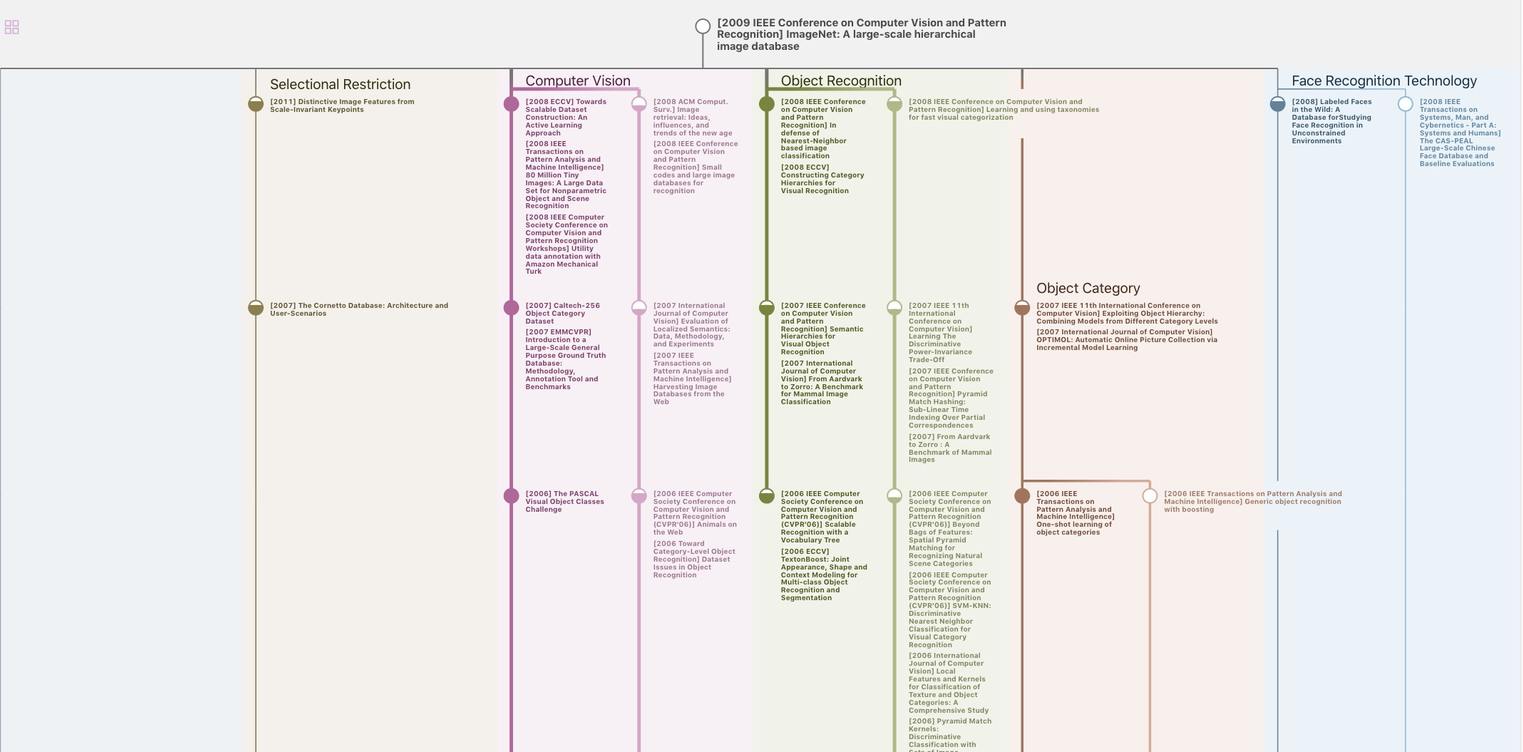
生成溯源树,研究论文发展脉络
Chat Paper
正在生成论文摘要