Distributed Tracing for Troubleshooting of Native Cloud Applications Via Rule-Induction Systems
Journal of universal computer science(2023)
摘要
Diagnosing IT issues is a challenging problem for large-scale distributed cloud environments due to complex and non-deterministic interrelations between the system components. Modern monitoring tools rely on AI-empowered data analytics for detection, root cause analysis, and rapid resolution of performance degradation. However, the successful adoption of AI solutions is anchored on trust. System administrators will not unthinkingly follow the recommendations without sufficient interpretability of solutions. Explainable AI is gaining popularity by enabling improved confidence and trust in intelligent solutions. For many industrial applications, explainable models with moderate accuracy are preferable to highly precise black-box ones. This paper shows the benefits of rule-induction classification methods, particularly RIPPER, for the root cause analysis of performance degradations. RIPPER reveals the causes of problems in a set of rules system administrators can use in remediation processes. Native cloud applications are based on the microservices architecture to consume the benefits of distributed computing. Monitoring such applications can be accomplished via distributed tracing, which inspects the passage of requests through different microservices. We discuss the application of rule-learning approaches to trace traffic passing through a malfunctioning microservice for the explanations of the problem. Experiments performed on datasets from cloud environments proved the applicability of such approaches and unveiled the benefits.
更多查看译文
关键词
cloud-native applications,application troubleshooting,distributed tracing,RED metrics,root cause analysis,explainable AI,rule-induction systems,RIPPER
AI 理解论文
溯源树
样例
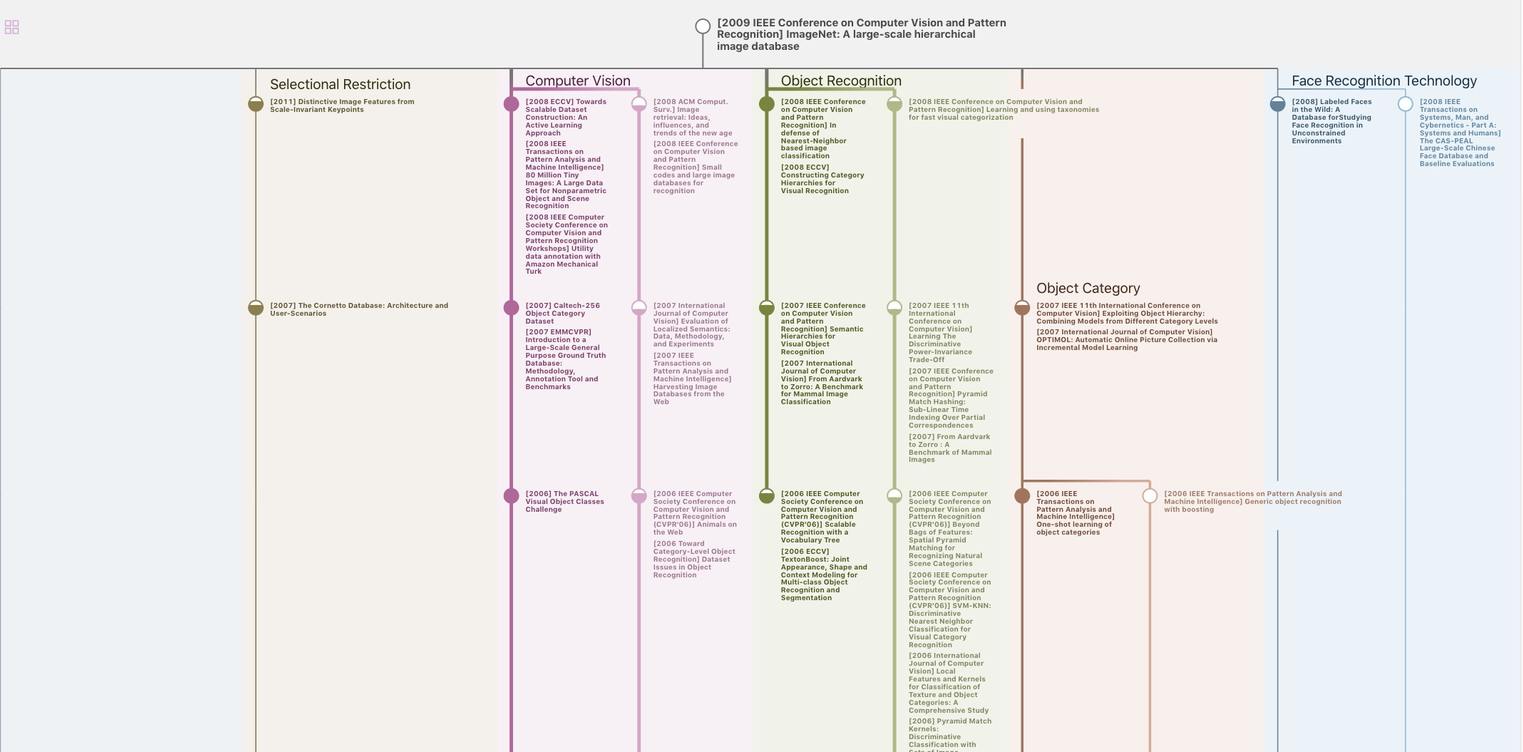
生成溯源树,研究论文发展脉络
Chat Paper
正在生成论文摘要