Multi-Hypothesis Task Planning: integrating temporal AI planning and semantic world modeling for AUV inspections in unknown environments
OCEANS 2023 - Limerick(2023)
摘要
Autonomous Underwater Vehicles (AUVs) have become fundamental tools for marine scientists and industries to explore and monitor underwater areas. Nonetheless, the collected data quality is not guaranteed: AUVs passively store the sensor acquisitions that are then analyzed offline after their recovery by human operators in charge of identifying and localizing the so-called Objects of Potential Interest (OPIs). As a consequence of these statements, the marine community has sought robots able to meaningfully perceive and model the surroundings while autonomously selecting and performing the requested tasks, which are the foundations of fully autonomous vehicles. Motivated by such considerations, the novel Multi-Hypothesis Task Planning (MH-TP) architecture has been designed to integrate temporal Artificial Intelligence (AI) based planning with semantic world modeling techniques toward fully autonomous AUV inspections in unknown environments. The proposed architecture extends the standard AI planning methodologies relying on the Problem Domain Definition Language (PDDL) by dynamically varying the PDDL problem with the probabilistic, semantically enriched objects that the World Modeling (WM) itself evaluates. The developed methodology has been validated with realistic simulations made by means of the UUV Simulator, where a dynamic model of FeelHippo AUV was implemented alongside the requested perception and payload devices.
更多查看译文
关键词
AUVs,Underwater Robotics,Task Planning,Underwater World Modeling,Automatic Target Recognition
AI 理解论文
溯源树
样例
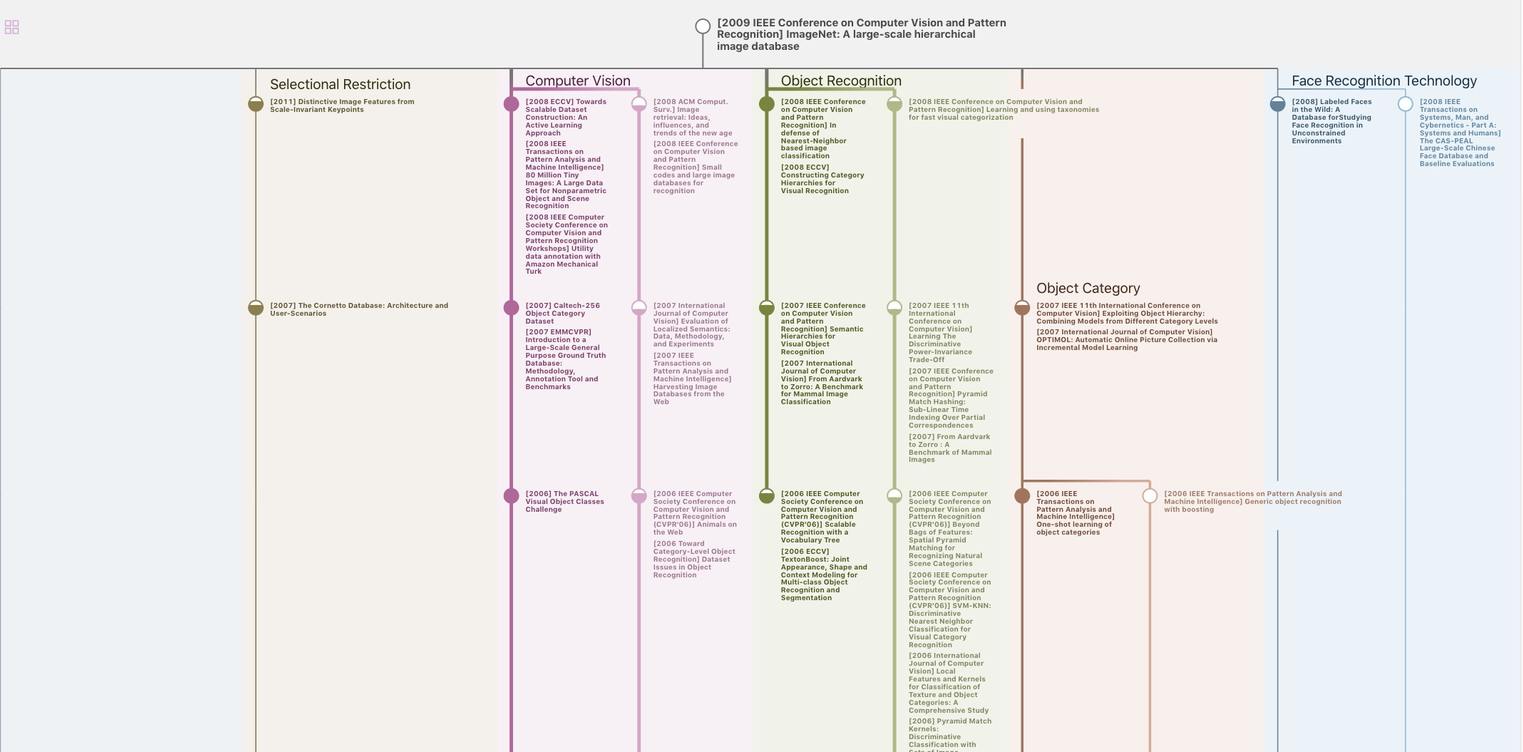
生成溯源树,研究论文发展脉络
Chat Paper
正在生成论文摘要