Smoke Detection in Complex Scenery Using Improved YOLOV5
2023 8th International Conference on Intelligent Computing and Signal Processing (ICSP)(2023)
摘要
Considering smoke detection in a complex scene, high smoke object error rate and low detection efficiency, an improved YOLOV5 for smoke detection is proposed in this paper. To improve the effectiveness of smoke detection algorithm for smoke targets in complex scenes. First, a new lightweight convolution technique GSConv is introduced to replace the Conv layer in the neck layer for feature extraction, and the original C3 module is replaced by Cross Stage Partial Network (GSCSP) module VoVGSCSP. This improvement reduces the computation complexity of the algorithm while maintaining the detection accuracy. Second, CIoU is replaced by SIoU as the regression function of the prediction box to improve the prediction accuracy of the prediction box and reduce the rate of missed detection of the target. Finally, to improve the performance of the deep network, Dynamic ReLU is introduced as an activation function (dynamically adjusting the ReLU parameters depending on the input data). Experiments on the SM-dataset (smoke detection dataset) show that the improved model improves the accuracy and precision of target smoke detection compared to the YOLOV5 model. Its precision is increased by 1.8%, mAP@0.5 is increased by 1.0%, the complexity of the model is reduced by 23.8%. The improved algorithm proposed in this paper can effectively extract smoke features and more suitable for smoke detection tasks in complex scenes.
更多查看译文
关键词
Computer vision,Smoke detection,YOLOV5
AI 理解论文
溯源树
样例
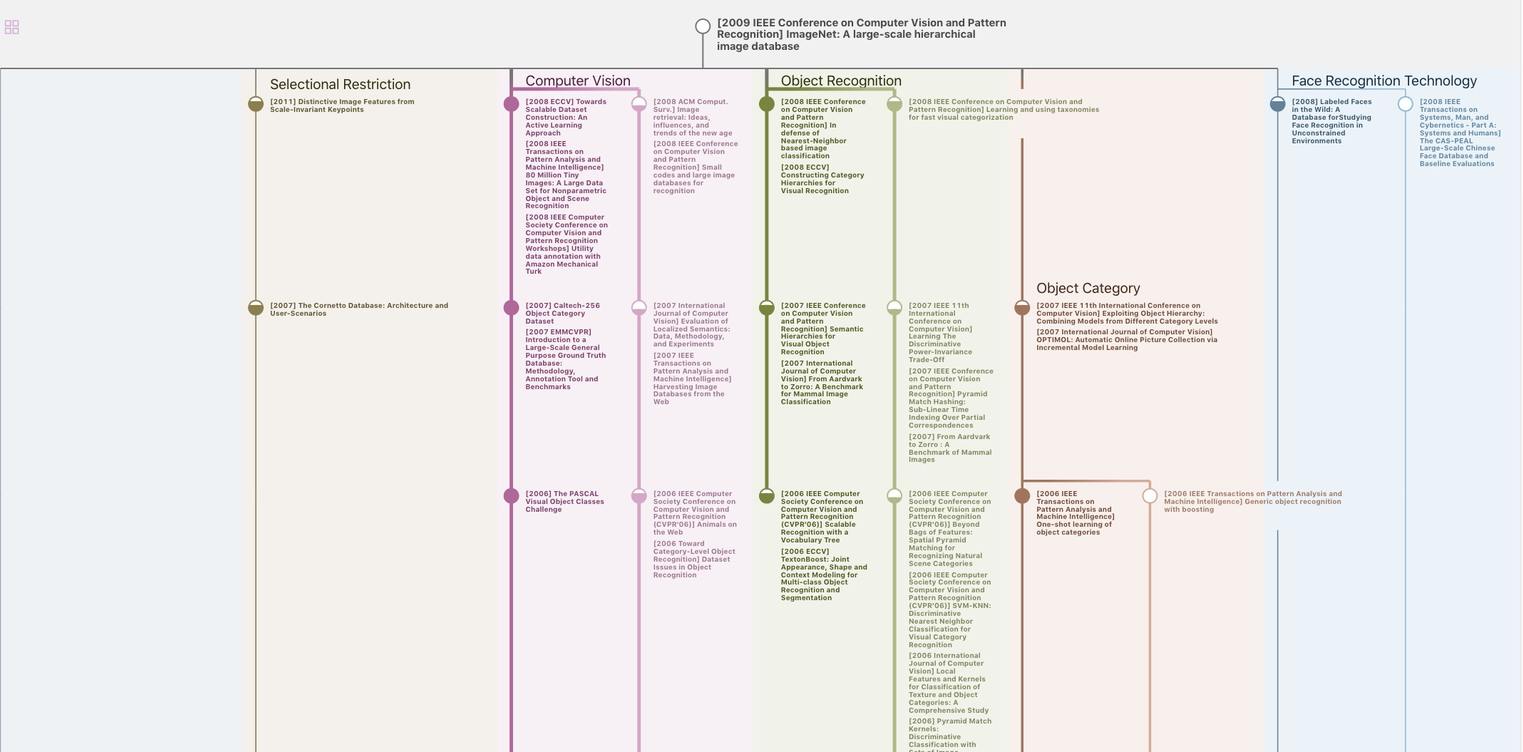
生成溯源树,研究论文发展脉络
Chat Paper
正在生成论文摘要