Unmasking Fraud in Food Delivery Business: Harnessing Amazon Web Services’ Random Cut Forest for Efficient Detection
2023 3rd International Conference on Electrical, Computer, Communications and Mechatronics Engineering (ICECCME)(2023)
摘要
This paper presents the development and evaluation of a fraud detection model for identifying fraudulent cases and anomalous instances in a business system. The authors faced a dilemma between choosing a univariate model (Isolation Forest) or a multivariate model (Random Cut Forest) to identify fraudulent cases and ultimately decided on the multivariate model due to its ability to detect all types of anomalies and its safety for the system. The model was evaluated using a limited number of confirmed fraudulent cases and a cutoff score of 1.4 and was able to identify instances similar to the known cases of fraud. In addition to identifying confirmed cases of fraud, the model also identified anomalies that required further investigation, which was essential in building a more effective supervised model and improving the system’s accuracy in identifying future instances of fraud. The findings from the investigations were also used to refine the engine rules, strengthening the overall security and stability of the system. Although the abstract does not mention the specific context of a food delivery business or Amazon, the paper describes a fraud detection model that could be applied in various business systems. The paper contributes to the current state of knowledge by presenting a reliable and effective fraud detection model that improves the overall security and stability of a business system.
更多查看译文
关键词
fraud detection,Random Cut Forest,cutoff score,Isolation Forest,univariate,multivariate,food delivery,anomalies
AI 理解论文
溯源树
样例
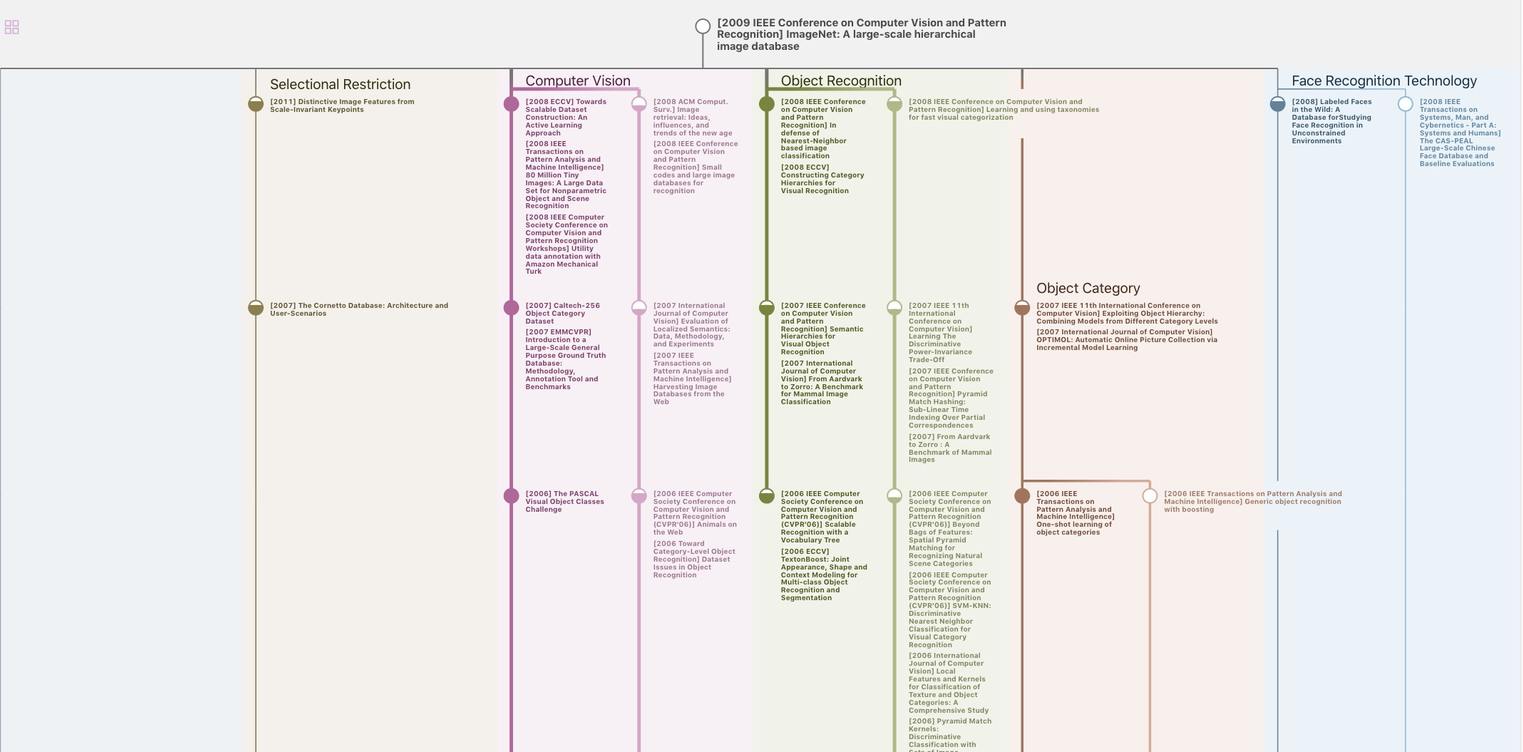
生成溯源树,研究论文发展脉络
Chat Paper
正在生成论文摘要