Comparative Study of Different Optical Character Recognition Models on Handwritten and Printed Medical Reports
2023 International Conference on Innovative Data Communication Technologies and Application (ICIDCA)(2023)
摘要
Text may be extracted from an image using a method called optical character recognition (OCR). An OCR is mostly used to translate existing paper documents or image data into editable documents. To create an OCR, a large variety of algorithms are needed, and it essentially functions in two stages, such as character and word detection. To retain a document's structure while using a more advanced technique, an OCR additionally focuses. The advancement in OCR has been very promising lately. Different tools have arrived in the market ranging from open-source tools PyTesseract to big established companies releasing their own OCR tools like Amazon's AWS Textract and Google's Google Vision. The accuracy of modern OCR techniques like AWS Textract, Google Vision, and updated versions of Pytesseract haven't been analyzed for different datasets. For complex datasets like a CBC report, there are various parameters and fields in a tabular and unstructured fashion. Every algorithm has a different approach to extracting the text from the image, so it becomes difficult to determine how accurate these models are on such datasets. Based on 2 different datasets, one handwritten and the other printed CBC images, this study performed analysis using the above 3 models by building an end-to-end pipeline to retrieve the necessary fields, thus making it feasible to access the accuracy of these models. Upon testing these models' accuracy, the study concluded that the AWS Textract model was the highest-performing model for both datasets, achieving an accuracy of 95% for the printed dataset and 89.42% for the handwritten IAM dataset after preprocessing.
更多查看译文
关键词
Optical Character Recognition,PyTesseract,Amazon Web Services Textract,Google Vision,IAM,Complete Blood Cell Report
AI 理解论文
溯源树
样例
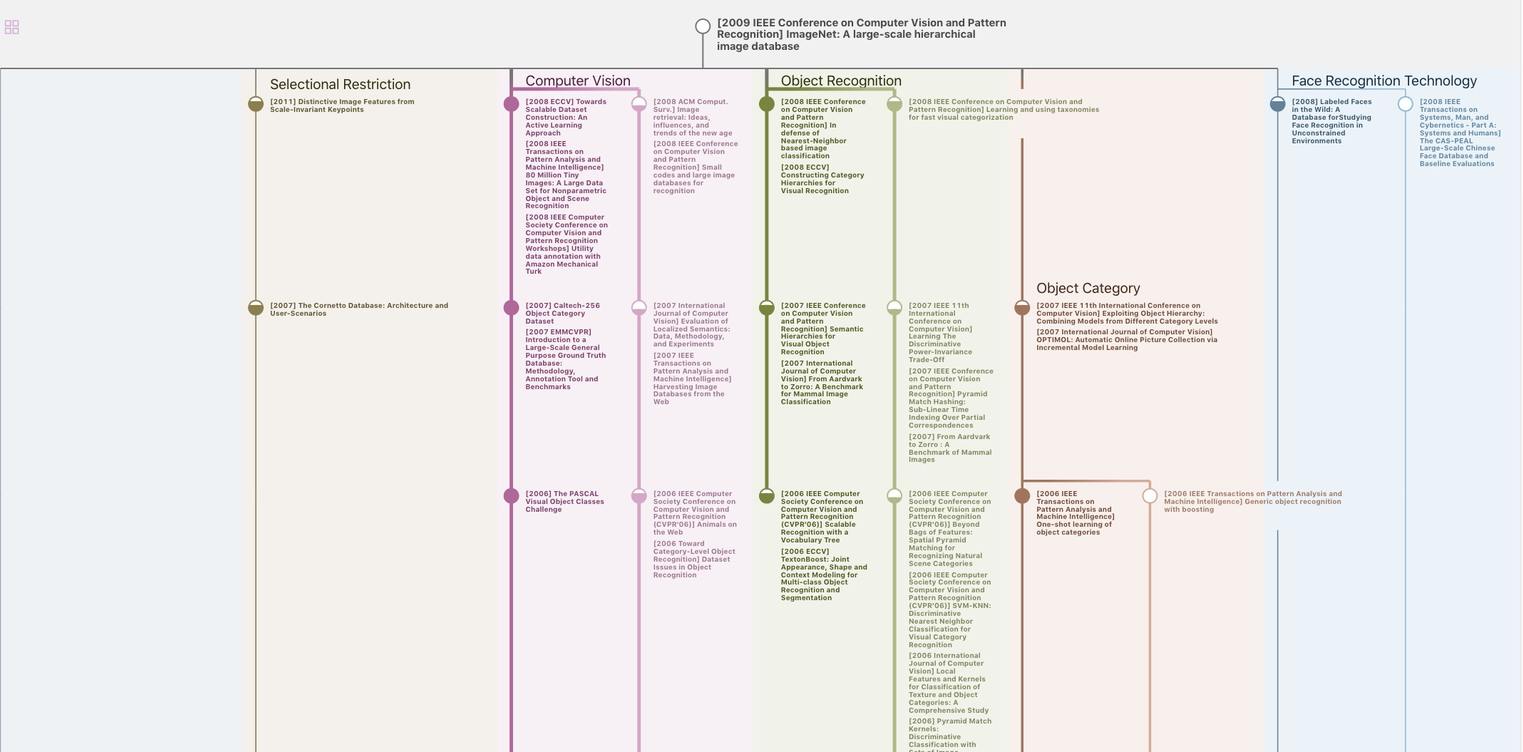
生成溯源树,研究论文发展脉络
Chat Paper
正在生成论文摘要