DSC-GraspNet: A Lightweight Convolutional Neural Network for Robotic Grasp Detection
2023 9th International Conference on Virtual Reality (ICVR)(2023)
Abstract
Grasp detection is an essential task for robots to achieve autonomous operation, it can also make virtual reality-based teleoperation more intelligent and reliable. Existing learning-based grasp detection methods usually fail to strike a balance between high accuracy and low time consumption. Also, the large number of model parameters tends to make these methods expensive to deploy. To solve this problem, a lightweight generative grasp detection network DSC-GraspNet is proposed. Firstly, Depth-separable convolutional blocks with Coordinate Attention (CA) are stacked to obtain a lightweight backbone network for feature extraction. Then multi-level features extracted by the backbone network are fused by the Cross Stage Partial (CSP) block in the up-sampling network. Finally, pixel-level grasp candidates are generated by grasp generating heads. Experimental results shows that an accuracy of 98.3% under image-wise splitting and 97.7% under object-wise splitting can be achieved on the Cornell public dataset. Meanwhile, an accuracy of 94.7% is achieved on the Jacquard dataset using the depth map as inputs. Our method also achieve a grasp success rate of 86.4% in the simulated grasp test. In addition, our network is able to inference an RGB-D image within 14ms, and can be applied to closed-loop grasping scenarios.
MoreTranslated text
Key words
Robot,grasp detection,convolutional neural network,depth-separable convolution
AI Read Science
Must-Reading Tree
Example
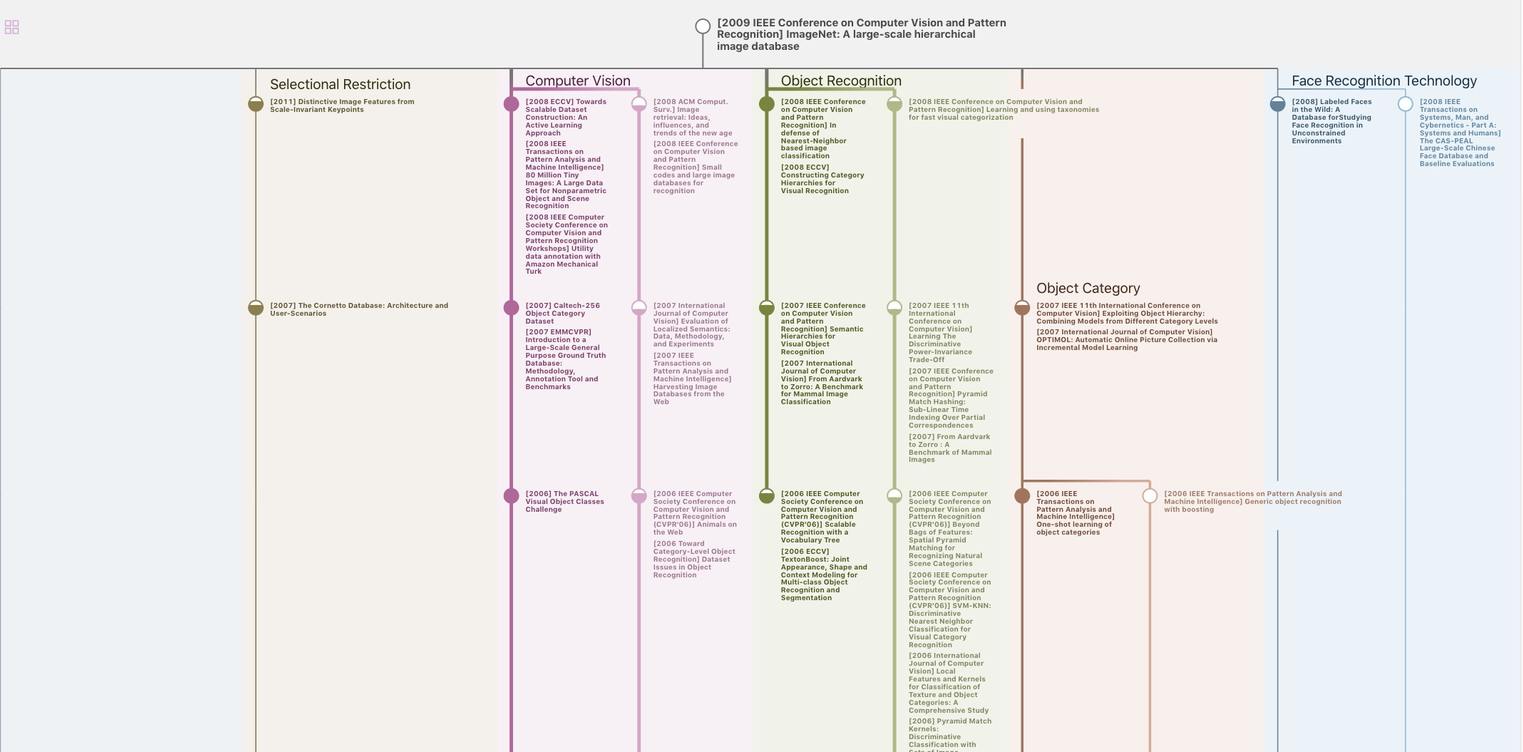
Generate MRT to find the research sequence of this paper
Chat Paper
Summary is being generated by the instructions you defined