Comparative Effectiveness of Explainable Machine Learning Approaches for Extrauterine Growth Restriction Classification in Preterm Infants Using Longitudinal Data
FRONTIERS IN MEDICINE(2023)
Kangwon Natl Univ Hosp | Sangmyung Univ | Seoul Natl Univ
Abstract
IntroductionPreterm birth is a leading cause of infant mortality and morbidity. Despite the improvement in the overall mortality in premature infants, the intact survival of these infants remains a significant challenge. Screening the physical growth of infants is fundamental to potentially reducing the escalation of this disorder. Recently, machine learning models have been used to predict the growth restrictions of infants; however, they frequently rely on conventional risk factors and cross-sectional data and do not leverage the longitudinal database associated with medical data from laboratory tests.MethodsThis study aimed to present an automated interpretable ML-based approach for the prediction and classification of short-term growth outcomes in preterm infants. We prepared four datasets based on weight and length including weight baseline, length baseline, weight follow-up, and length follow-up. The CHA Bundang Medical Center Neonatal Intensive Care Unit dataset was classified using two well-known supervised machine learning algorithms, namely support vector machine (SVM) and logistic regression (LR). A five-fold cross-validation, and several performance measures, including accuracy, precision, recall and F1-score were used to compare classifier performances. We further illustrated the models’ trustworthiness using calibration and cumulative curves. The visualized global interpretations using Shapley additive explanation (SHAP) is provided for analyzing variables’ contribution to final prediction.ResultsBased on the experimental results with area under the curve, the discrimination ability of the SVM algorithm was found to better than that of the LR model on three of the four datasets with 81%, 76% and 72% in weight follow-up, length baseline and length follow-up dataset respectively. The LR classifier achieved a better ROC score only on the weight baseline dataset with 83%. The global interpretability results revealed that pregnancy-induced hypertension, gestational age, twin birth, birth weight, antenatal corticosteroid use, premature rupture of membranes, sex, and birth length were consistently ranked as important variables in both the baseline and follow-up datasets.DiscussionThe application of machine learning models to the early detection and automated classification of short-term growth outcomes in preterm infants achieved high accuracy and may provide an efficient framework for clinical decision systems enabling more effective monitoring and facilitating timely intervention.
MoreTranslated text
Key words
preterm birth,extrauterine growth restriction,machine learning,classification,model trustworthy,interpretability
PDF
View via Publisher
AI Read Science
Must-Reading Tree
Example
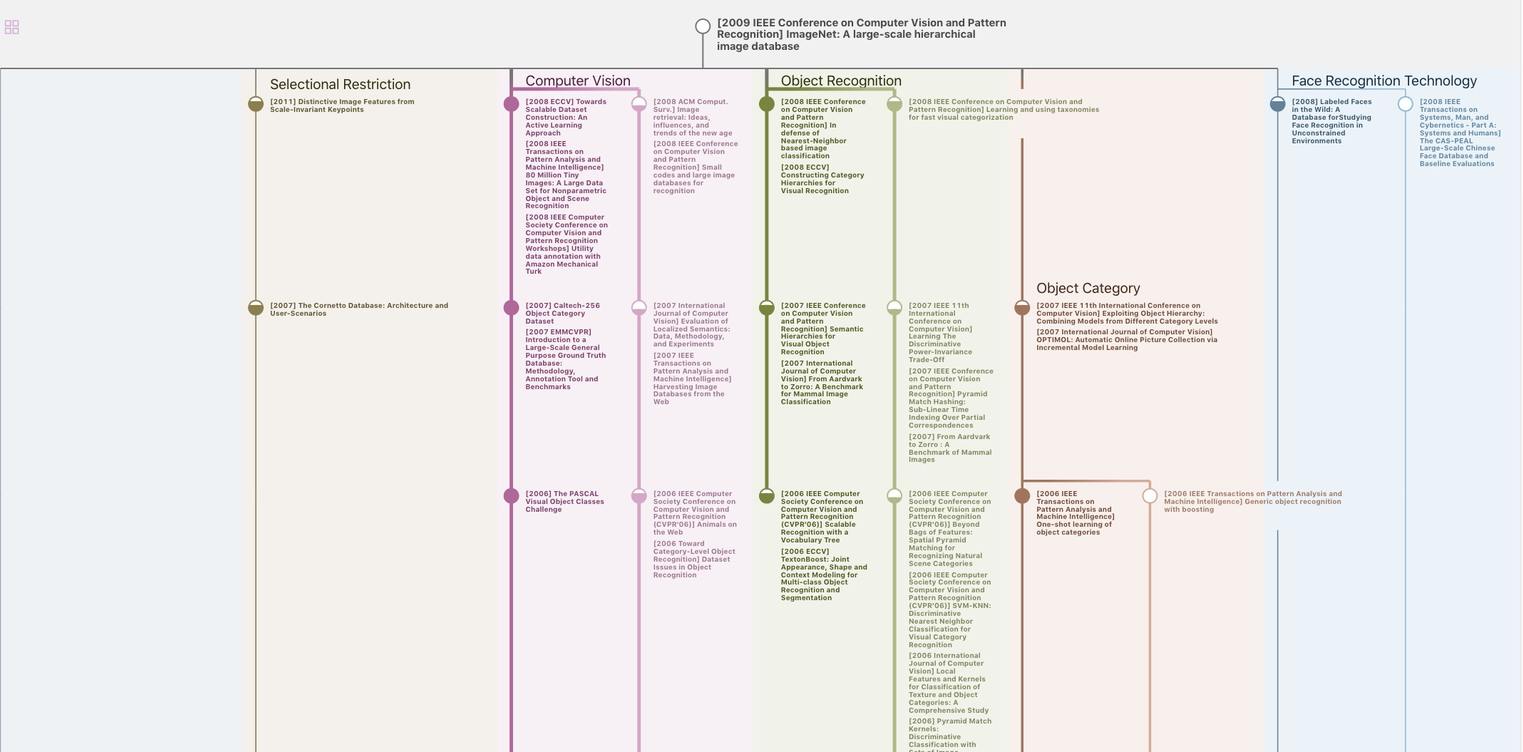
Generate MRT to find the research sequence of this paper
Related Papers
2003
被引用592 | 浏览
2001
被引用200 | 浏览
2013
被引用1926 | 浏览
2020
被引用41 | 浏览
2020
被引用95 | 浏览
2020
被引用21 | 浏览
Application 2D Descriptors and Artificial Neural Networks for Beta-Glucosidase Inhibitors Screening.
2020
被引用7 | 浏览
2021
被引用23 | 浏览
2022
被引用15 | 浏览
2022
被引用8 | 浏览
Data Disclaimer
The page data are from open Internet sources, cooperative publishers and automatic analysis results through AI technology. We do not make any commitments and guarantees for the validity, accuracy, correctness, reliability, completeness and timeliness of the page data. If you have any questions, please contact us by email: report@aminer.cn
Chat Paper
去 AI 文献库 对话