Developing an Automatic Training Technique Based on Integration of Radar and Optical Remotely Sensed Images for Building Extraction
Earth science informatics(2023)
摘要
Identifying and extracting urban features such as buildings and producing accurate information about the location of features from remotely sensed images is important in updating maps, spatial databases, urban planning, and meeting the needs of urban services. Supervised methods of producing training data were widely applied in deriving accurate information from such images. However, those approaches require human intervention and are therefore time-consuming, and associated with classification accuracy limitations. This study presents a novel approach for extracting buildings from remotely sensed images by automating training data selection steps for supervised classification. To prepare training samples intelligently and automatically, a knowledge-based integration of radar and optical images was used. First, a general height constraint was applied to a digital surface model (DSM) obtained from radar images to divide the image pixels into two parts. Then, by applying a local height filter in each part, pixels representing buildings were extracted. Considering that the buildings are not the only features with significant heights, another constraint defined as coherence constraint was considered. Then, histogram of the optical image pixels corresponding to the filtered radar DSM pixels extracted from the previous steps was used to select sample training pixels. By considering pixels within the maximum range of the histogram, the final building samples for Support Vector Machine (SVM) classification were derived and applied for building extraction. The output showed that it sufficiently and reliably improved the existing supervised classification limitation to extract building. The performance of the proposed method was evaluated over different image datasets regardless of input image type and region. It achieved an overall average accuracy of 92% and a kappa coefficient of 0.83.
更多查看译文
关键词
Automatic Feature Extraction,Training Data,Remote Sensing,Building Extraction,Radar Images,Optical Images
AI 理解论文
溯源树
样例
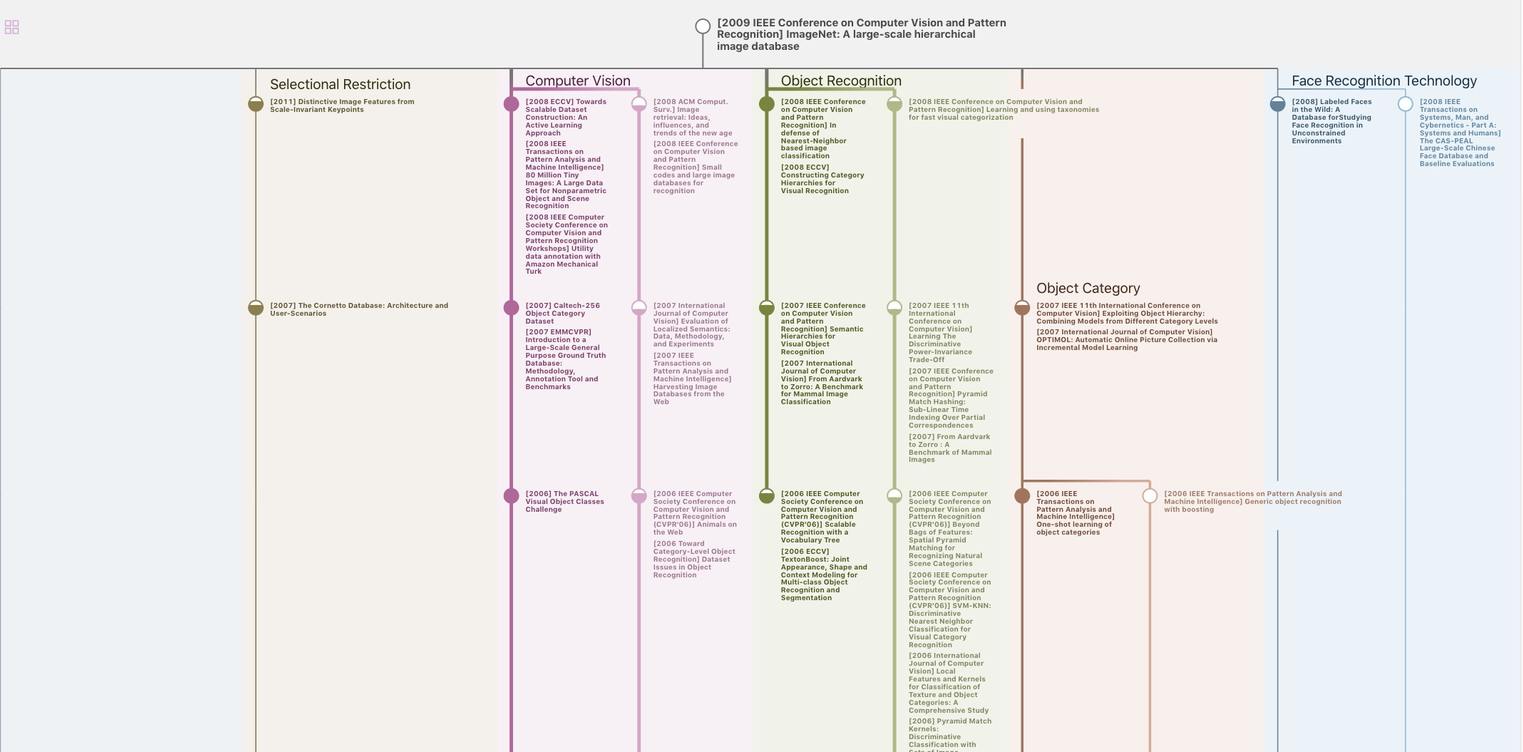
生成溯源树,研究论文发展脉络
Chat Paper
正在生成论文摘要