Positive-Pair Redundancy Reduction Regularisation for Speech-Based Asthma Diagnosis Prediction
ICASSP 2023 - 2023 IEEE International Conference on Acoustics, Speech and Signal Processing (ICASSP)(2023)
摘要
Asthma affects an estimated 334 million people worldwide, causing over 461 000 deaths. Exacerbations or asthma attacks can be predicted with new sensor technologies. We explore how recordings of human voice, and machine learning can provide better diagnostics for pulmonary diseases like asthma, as well as tools for helping patients better manage it. Past studies have focused on data collection processes that either mimic traditional auscultation, or make multi-sensor measurements, where the application of specialised recording hardware is required, possibly by expert personnel. This is costly and places limits on the size of the studies (e.g., number of study participants, and recording devices). In this paper, we consider another avenue, that of modelling self-recorded voice samples made using regular smartphones, along with self-reported clinical diagnosis annotations; specifically of asthma. We propose the usage of self-supervised learning that aims to reduce within-class representation redundancy among heterogeneous samples as an auxiliary task to promote robust, bias-free learning. The application of our method achieves an absolute increase of 1.80% in area under the Precision-Recall curve, compared to not using it, and a total of 3.54% compared to our baseline.
更多查看译文
关键词
Asthma,speech-modelling,self-supervised-learning,redundancy-reduction,dataset-bias-reduction
AI 理解论文
溯源树
样例
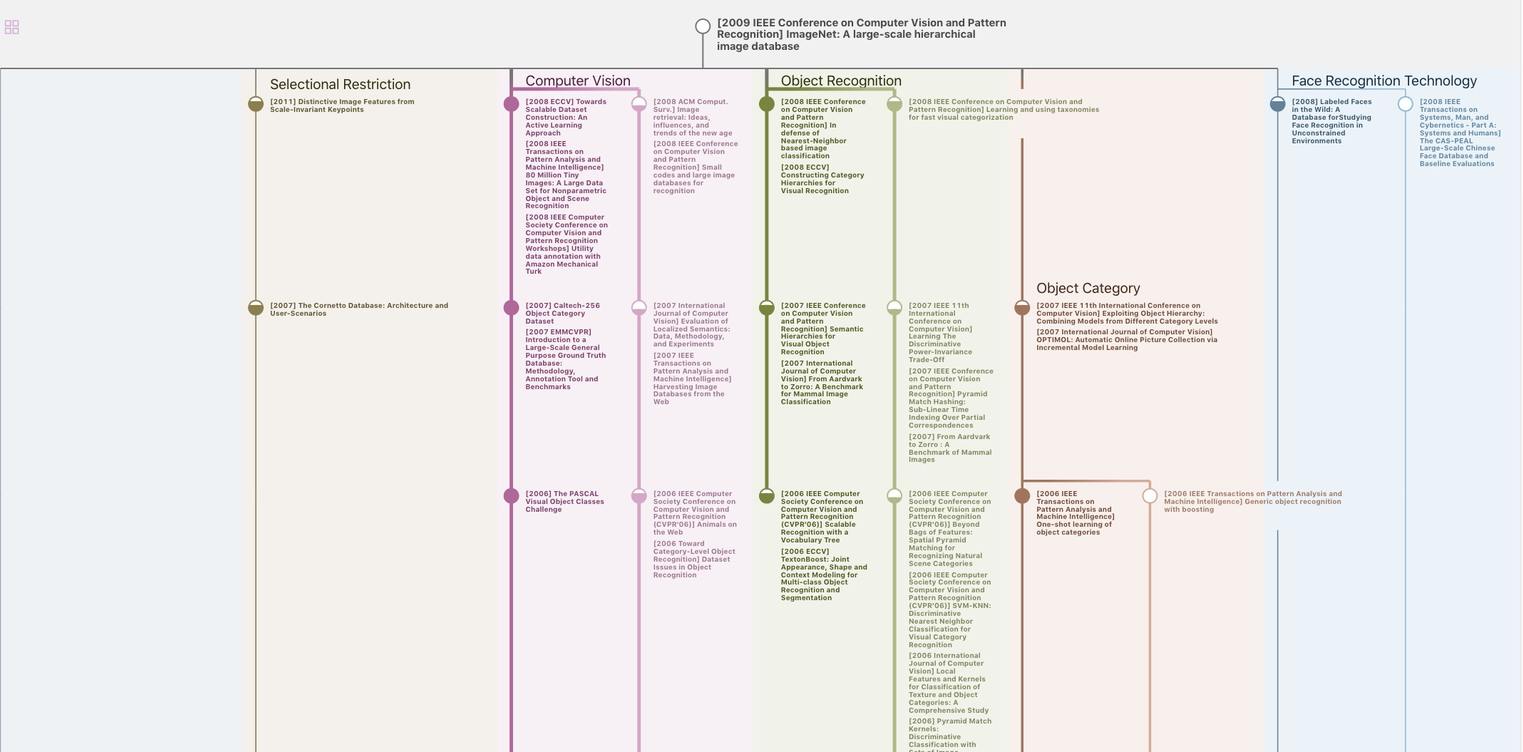
生成溯源树,研究论文发展脉络
Chat Paper
正在生成论文摘要