Exploring Universal Singing Speech Language Identification Using Self-Supervised Learning Based Front-End Features
ICASSP 2023 - 2023 IEEE International Conference on Acoustics, Speech and Signal Processing (ICASSP)(2023)
摘要
Despite the great performance of language identification (LID), there is a lack of large-scale singing LID databases to support the research of singing language identification (SLID). This paper proposed a over 3200 hours dataset used for singing language identification, called Slingua. As the baseline, we explore two self-supervised learning (SSL) models, WavLM and Wav2vec2, as the feature extractors for both SLID and universal singing speech language identification (ULID), compared with the traditional handcraft feature. Moreover, by training with speech language corpus, we compare the performance difference of the universal singing speech language identification. The final results show that the SSL-based features exhibit more robust generalization, especially for low-resource and open-set scenarios. The database can be downloaded following this repository: https://github.com/Doctor-Do/Slingua.
更多查看译文
关键词
Singing Language Identification (SLID),Universal singing speech Language Identification (ULID),Music Database
AI 理解论文
溯源树
样例
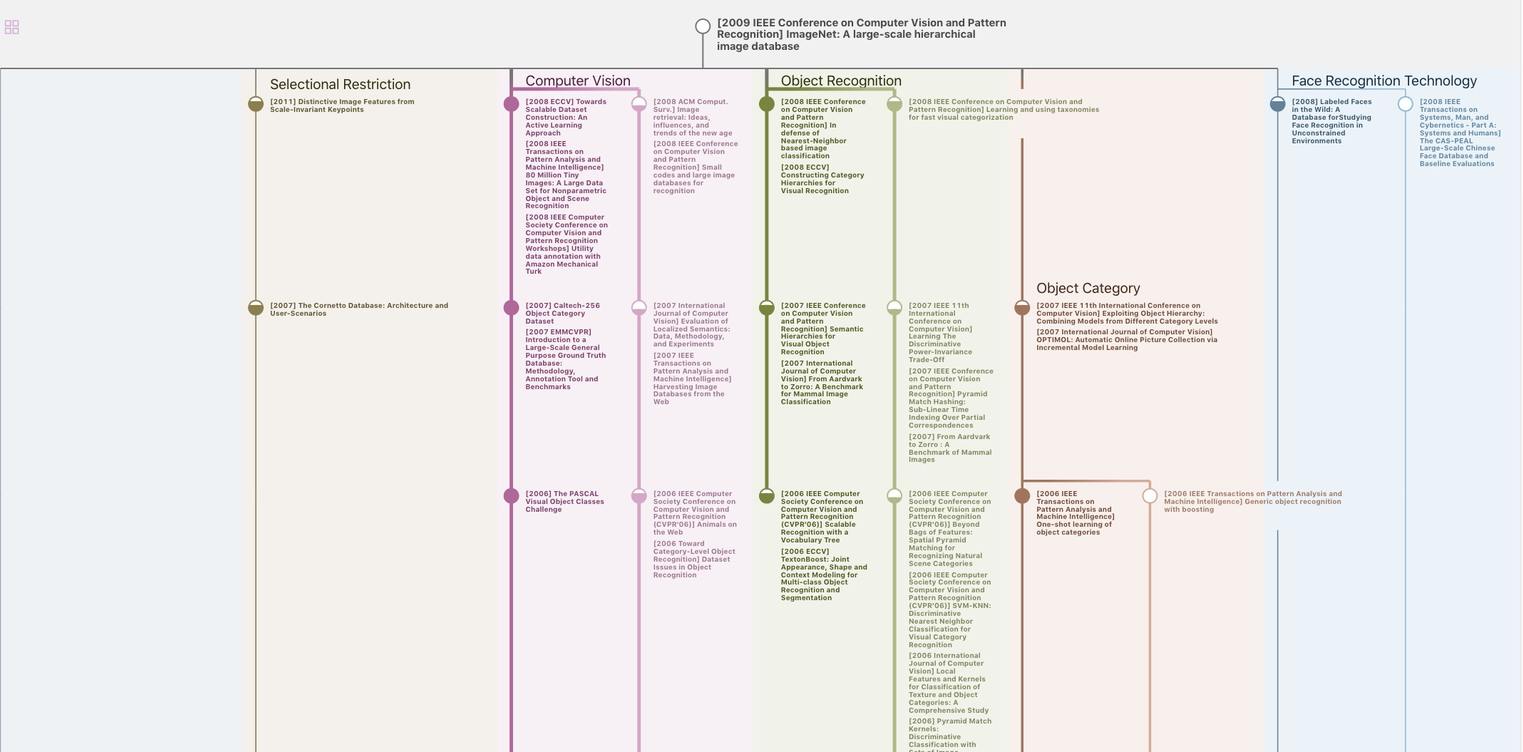
生成溯源树,研究论文发展脉络
Chat Paper
正在生成论文摘要