Assessing the Robustness of Deep Learning-Assisted Pathological Image Analysis Under Practical Variables of Imaging System
ICASSP 2023 - 2023 IEEE International Conference on Acoustics, Speech and Signal Processing (ICASSP)(2023)
摘要
With the advancement of deep learning, computer-assisted clinical diagnosis, such as liquid-based cervical cytology, has attracted more attention. However, the fragile robustness of deep learning models has a non-negligible impact on their classification accuracy and reliability. To be more specific, various scanner parameters will be used depending on the pathologist’s preferences during the clinical diagnosis process (e.g., field source brightness, contrast, saturation, etc.), and this variation will lead to the unstable performance of the model. In this paper, we construct an evaluation pathway to assess the stability and consistency of deep learning models under various customized scanner parameters. Specifically, a multi-scanned dataset consists of 4200 whole slide images (WSIs) is generated by scanning 200 stained slices using various scanner parameters. Moreover, we conducted a large number of experiments to investigate the robustness of numerous models, including convolution-based and transformer-based models concerning various scanner parameter settings. Furthermore, we introduce several indicators to analyze the prediction accuracy, consistency and robustness of the model on the constructed dataset. The experimental results indicate that the deep learning models are sensitive to luminance-related scanner parameters. In addition, transformer-based models have better robustness than traditional convolutional neural networks. Our code has been made available
1
.
更多查看译文
关键词
Deep learning,Cervical cytology images,Robustness,Scanner parameters
AI 理解论文
溯源树
样例
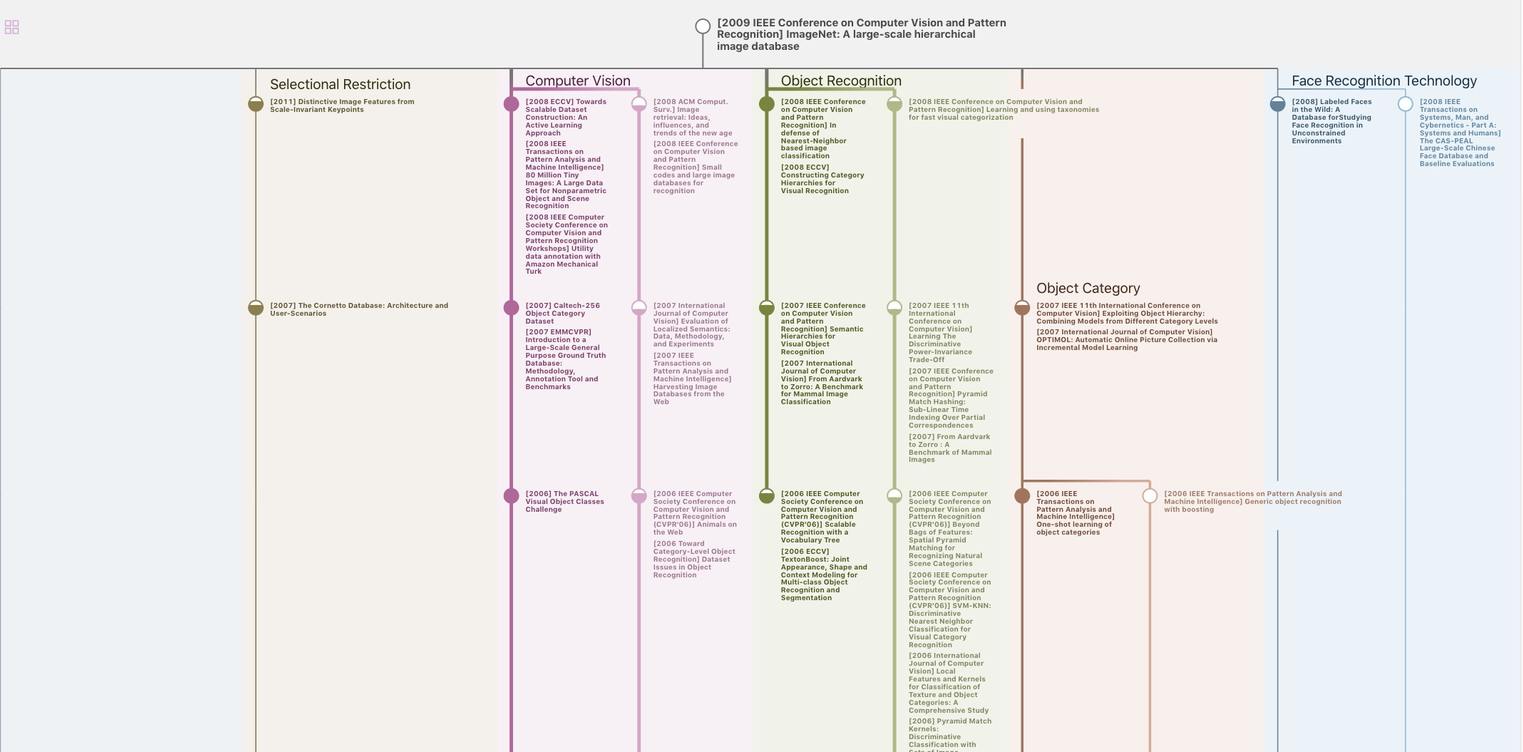
生成溯源树,研究论文发展脉络
Chat Paper
正在生成论文摘要