SADE: A Self-Adaptive Expert for Multi-Dataset Question Answering
ICASSP 2023 - 2023 IEEE International Conference on Acoustics, Speech and Signal Processing (ICASSP)(2023)
摘要
Multi-dataset question answering (QA) aims to combine multiple QA datasets to build models that not only perform well on training distributions, but also transfer and generalize well to new distributions. Some prior work considered building a collection of dataset-specific experts upon a shared Transformer, so as to simultaneously encode both regularities across datasets and specificities of each dataset. This approach, however, has its limitations when generalized to an unseen new distribution, and the number of extra parameters will increase with the number of training datasets. In this paper, we devise Self-ADaptive Expert (SADE), the key idea of which is to train a single expert that can be automatically adapted to each individual instance according to its gradients. This gradient-based, instance-level modulation scheme makes our approach easily adaptable to any instance from unseen new distributions, and keeps the number of extra parameters as a constant. We further design a contrastive learning mechanism to enhance the discriminability of modulation signals across different datasets. Experimental results on twelve QA datasets demonstrate that SADE consistently outperforms previous state-of-the-art in all the three settings including in-domain learning, few-shot transfer learning, and zero-shot generalization.
更多查看译文
关键词
question answering,multi-dataset,transfer learning,generalization
AI 理解论文
溯源树
样例
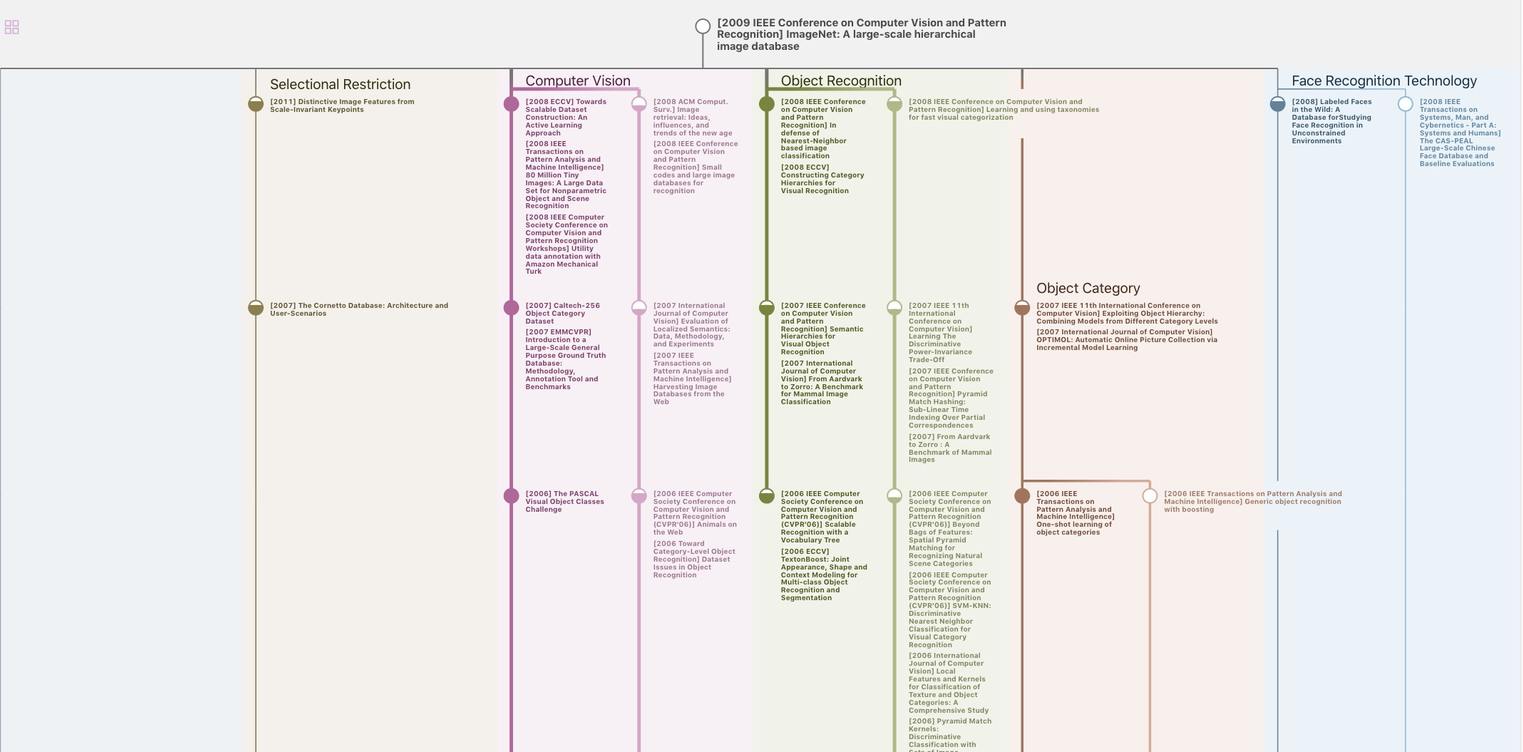
生成溯源树,研究论文发展脉络
Chat Paper
正在生成论文摘要