SAT-verifiable LTL Satisfiability Checking via Graph Representation Learning
2023 38TH IEEE/ACM INTERNATIONAL CONFERENCE ON AUTOMATED SOFTWARE ENGINEERING, ASE(2023)
摘要
With the superior learning ability of neural networks, it is promising to obtain highly confident results for linear temporal logic (LTL) satisfiability checking in polynomial time. However, existing neural approaches are limited in inductive ability and in supporting with an arbitrary number of atomic propositions. Besides, there is no mechanism to verify the results for satisfiability checking. In this paper, we propose an approach to checking the satisfiability of an LTL formula and meanwhile generating a satisfiable trace if the LTL formula is satisfiable, where the satisfiable trace verifies the satisfiability result. The core contribution is a new graph representation for LTL formulae - one-step unfolded graph (OSUG) to incorporate the syntax and semantic features of LTL. Preliminary results show that our approach is superior to the state-of-the-art neural approaches on synthetic datasets and confirms the effectiveness of OSUG.
更多查看译文
关键词
linear temporal logic,satisfiability checking,graph representation learning
AI 理解论文
溯源树
样例
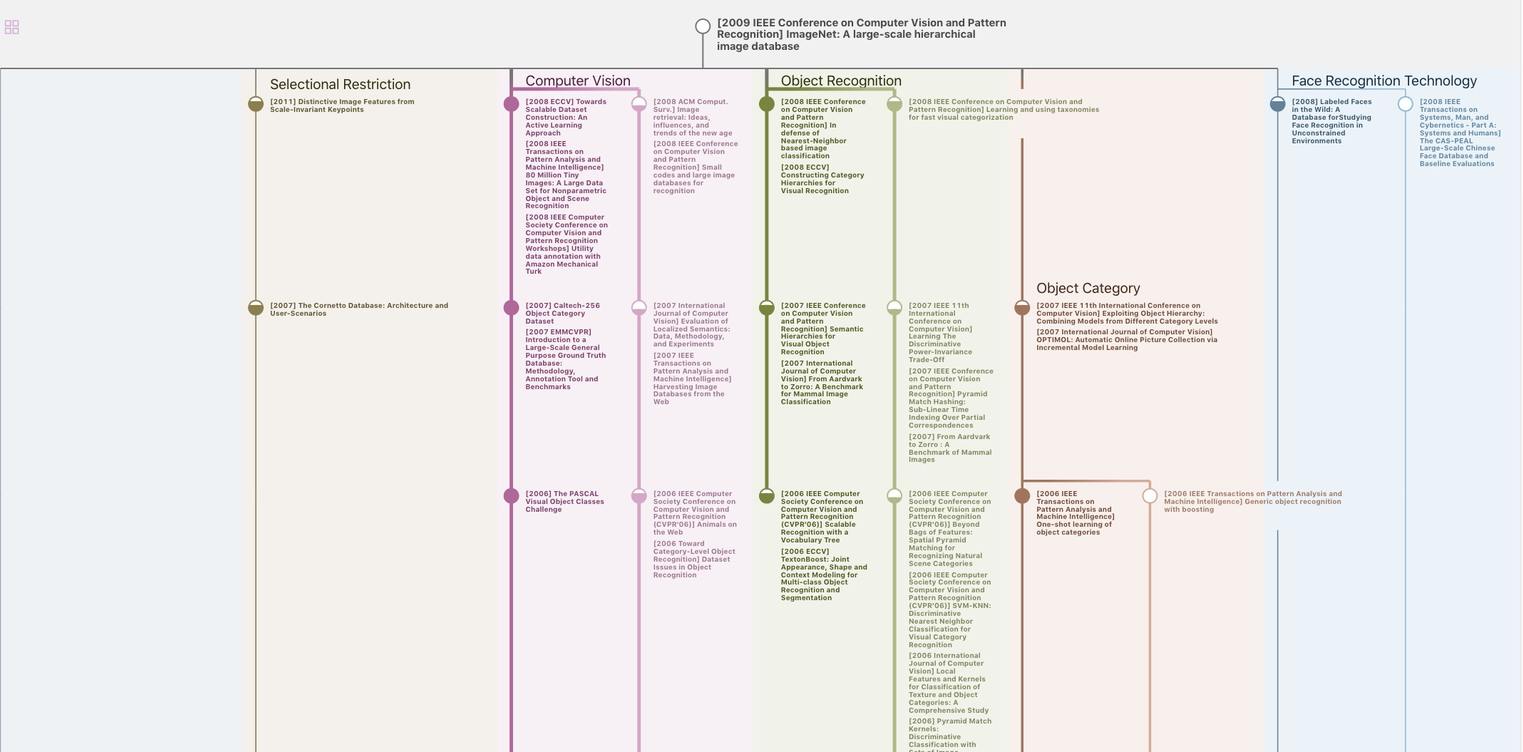
生成溯源树,研究论文发展脉络
Chat Paper
正在生成论文摘要