DCA-DAFFNet: an End-to-End Network with Deformable Fusion Attention and Deep Adaptive Feature Fusion for Laryngeal Tumor Grading from Histopathology Images
IEEE transactions on instrumentation and measurement(2023)
摘要
Laryngeal tumor grading is a challenging task for computer-aided clinical diagnosis (CACD), mainly because the nuclei in histopathological images have large differences in shape and distribution, and the complex spatial relationship between nuclei. However, the existing convolutional neural networks (CNNs)-based tumor grading models cannot adaptively represent the nuclei with variable morphology and lack of effective modeling for the semantic information of nuclear spatial location. Therefore, we propose an end-to-end network (DCA-DAFFNet) with deformable convolution guided attention block (DCAB) and deep adaptive feature fusion (DAFF) to improve the grading ability. Specifically, a novel DCAB is designed to represent variable nuclei adaptively with more flexible receptive fields of deformable convolution. Besides, to solve the problem of poor semantic information modeling ability of nuclear spatial location, the vision transformer (ViT) block is incorporated into the attention-based CNN models to construct DCA-DAFFNet with powerful global information representation capability. Furthermore, a DAFF method containing multikernel max mean discrepancy (MK-MMD) and adaptive fusion block (AFB) is proposed to solve the problem of negative fusion in DCA-DAFFNet. Extensive experiment results demonstrate the effectiveness of DCA-DAFFNet, and its average grading accuracy is increased to 90.78% ( $\uparrow 11.80$ %). By comparing visualization results, our proposed DCA-DAFFNet can pay more attention to the heterogeneous nuclei (polymorphic-nucleus, mega-nucleus, and so on) concerned by pathologists, which shows that our method is more interpretable and human-computer interactive.
更多查看译文
关键词
Attention mechanism,deformable convolution,laryngeal cancer,model fusion,tumor grading
AI 理解论文
溯源树
样例
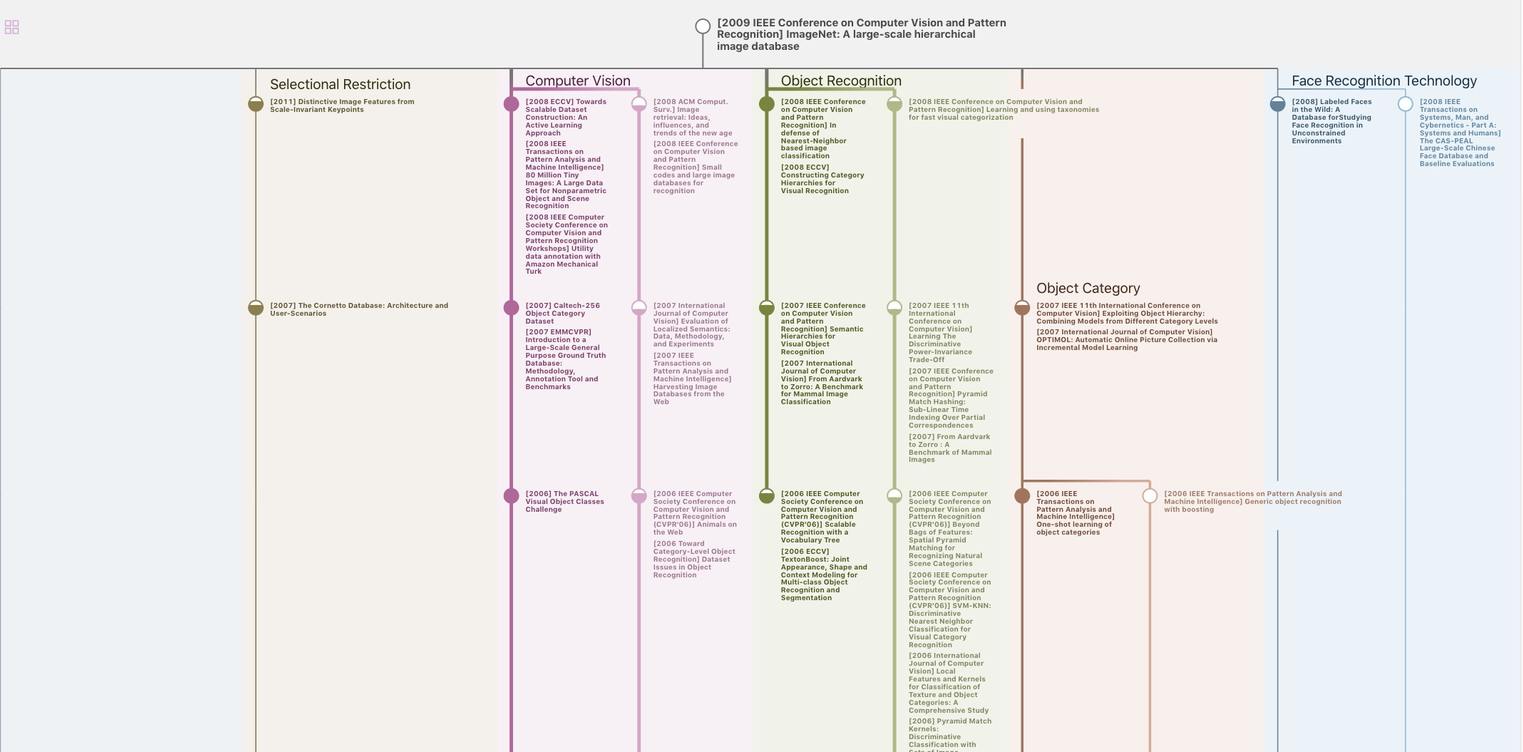
生成溯源树,研究论文发展脉络
Chat Paper
正在生成论文摘要