Evaluating Pretrained models for Deployable Lifelong Learning
2024 IEEE/CVF Winter Conference on Applications of Computer Vision Workshops (WACVW)(2023)
摘要
We create a novel benchmark for evaluating a Deployable Lifelong Learning
system for Visual Reinforcement Learning (RL) that is pretrained on a curated
dataset, and propose a novel Scalable Lifelong Learning system capable of
retaining knowledge from the previously learnt RL tasks. Our benchmark measures
the efficacy of a deployable Lifelong Learning system that is evaluated on
scalability, performance and resource utilization. Our proposed system, once
pretrained on the dataset, can be deployed to perform continual learning on
unseen tasks. Our proposed method consists of a Few Shot Class Incremental
Learning (FSCIL) based task-mapper and an encoder/backbone trained entirely
using the pretrain dataset. The policy parameters corresponding to the
recognized task are then loaded to perform the task. We show that this system
can be scaled to incorporate a large number of tasks due to the small memory
footprint and fewer computational resources. We perform experiments on our DeLL
(Deployment for Lifelong Learning) benchmark on the Atari games to determine
the efficacy of the system.
更多查看译文
关键词
Lifelong Learning,Scalable,Incremental Learning,Pre-training Dataset,Neural Network,Large Datasets,Feed-forward Network,Real-world Systems,Buffer Size,Types Of Games,Support Set,Robot Navigation,Number Of Games,Inductive Bias,Specific Game,Pre-training Phase,Catastrophic Forgetting,Specific Benchmark,Pre-trained Encoder
AI 理解论文
溯源树
样例
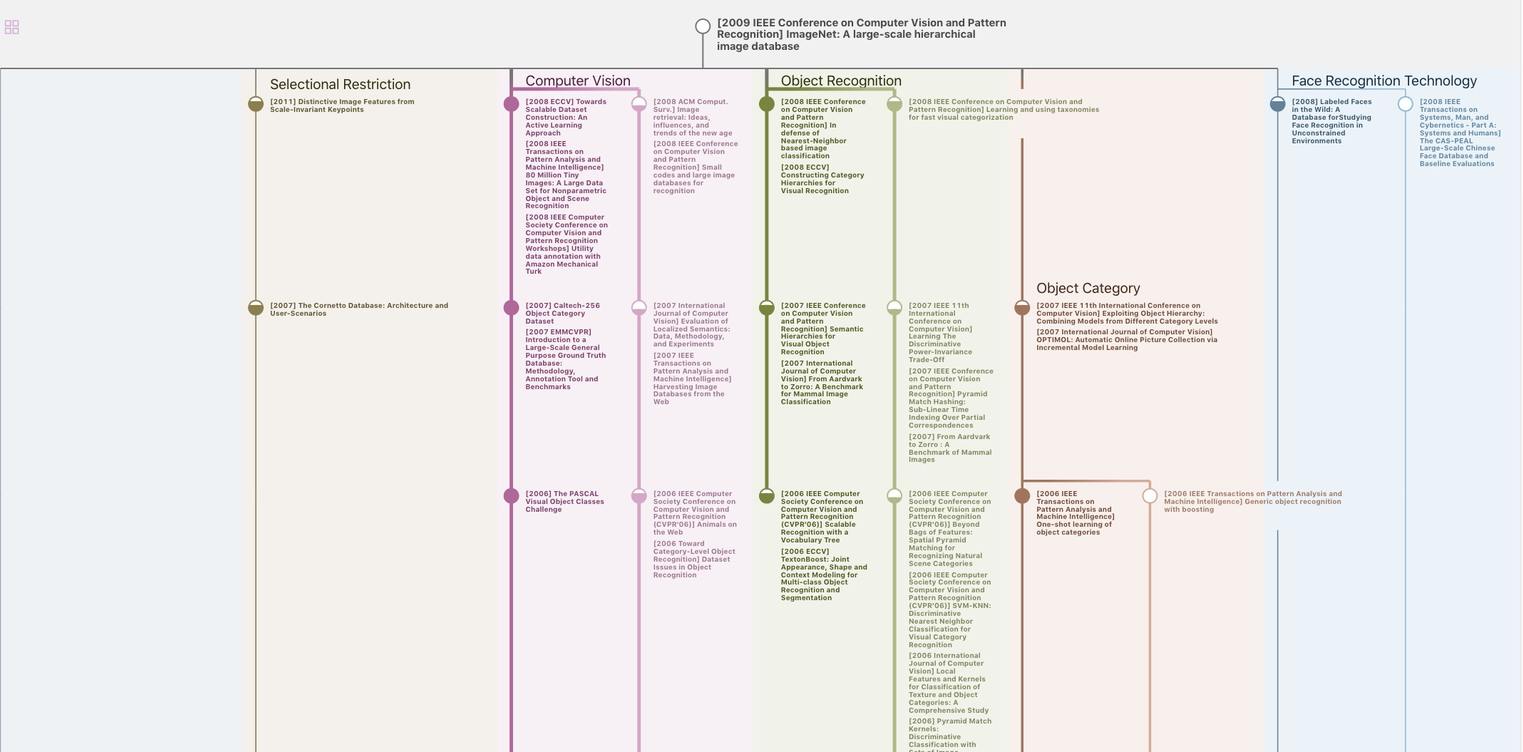
生成溯源树,研究论文发展脉络
Chat Paper
正在生成论文摘要