Two-stage label embedding via neural factorization machine for multi-label classification
AAAI'19/IAAI'19/EAAI'19: Proceedings of the Thirty-Third AAAI Conference on Artificial Intelligence and Thirty-First Innovative Applications of Artificial Intelligence Conference and Ninth AAAI Symposium on Educational Advances in Artificial Intelligence(2019)
摘要
Label embedding has been widely used as a method to exploit label dependency with dimension reduction in multi-label classification tasks. However, existing embedding methods intend to extract label correlations directly, and thus they might be easily trapped by complex label hierarchies. To tackle this issue, we propose a novel Two-Stage Label Embedding (TSLE) paradigm that involves Neural Factorization Machine (NFM) to jointly project features and labels into a latent space. In encoding phase, we introduce a Twin Encoding Network (TEN) that digs out pairwise feature and label interactions in the first stage and then efficiently learn higher-order correlations with deep neural networks (DNNs) in the second stage. After the codewords are obtained, a set of hidden layers is applied to recover the output labels in decoding phase. Moreover, we develop a novel learning model by leveraging a max margin encoding loss and a label-correlation aware decoding loss, and we adopt the mini-batch Adam to optimize our learning model. Lastly, we also provide a kernel insight to better understand our proposed TSLE. Extensive experiments on various real-world datasets demonstrate that our proposed model significantly outperforms other state-of-the-art approaches.
更多查看译文
AI 理解论文
溯源树
样例
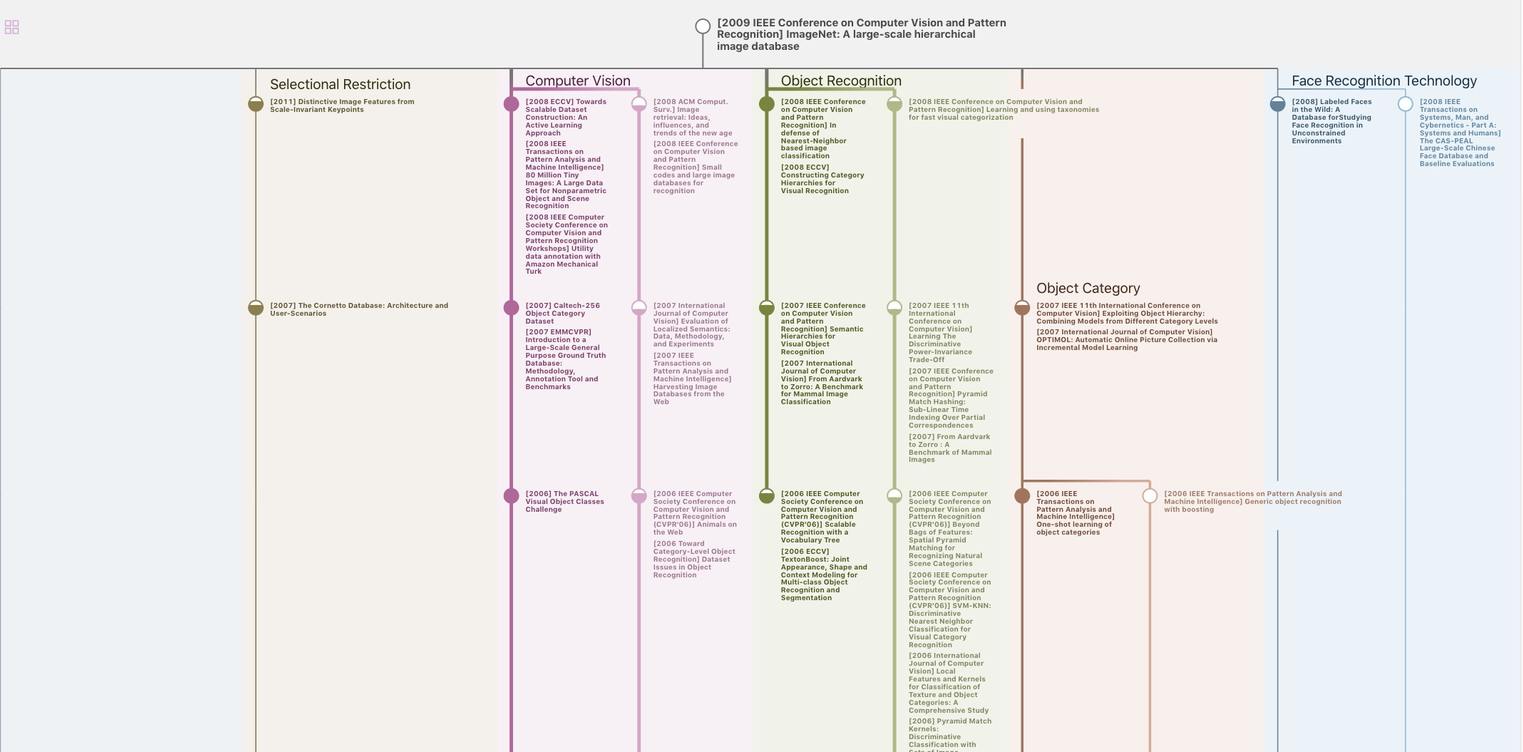
生成溯源树,研究论文发展脉络
Chat Paper
正在生成论文摘要