Mitigating Privacy Leakage in Anomalous Building Data Streams
PROCEEDINGS OF THE 10TH ACM INTERNATIONAL CONFERENCE ON SYSTEMS FOR ENERGY-EFFICIENT BUILDINGS, CITIES, AND TRANSPORTATION, BUILDSYS 2023(2023)
摘要
The presence of anomalies in building datasets represents a unique privacy challenge. There is a risk of successful identification within the complex and voluminous data streams for a building’s occupancy, CO2, temperature, humidity and power consumption. This can leak patterns of usage and other derived information that adversaries can leverage for both cyber and real-world attacks. However, this issue needs to be weighed against the indispensable roles that Building Management Systems (BMS) can play in reducing power consumption and, thus, the emissions for commercial buildings. Our initial work attempts to balance these competing demands, by implementing a decentralized Internet of Things (IoT) architecture against the Data Clearing House (DCH), an established repository housing multitudes of live building data streams. The aim is to detect and smooth the presence of anomalies, which would limit the exposure of sensitive information before it reaches the cloud for further analysis. To this end, we began by analyzing the historical streams for CO2, occupancy and temperature for a selected building within DCH. We then applied a fast and lightweight anomaly detection method using the PyOD python library. A privacy-preserving architecture is then described, where a well-known Differential Privacy (DP) technique was also applied and studied.
更多查看译文
关键词
building management systems,building occupancy,time-series data,anomaly detection,differential privacy
AI 理解论文
溯源树
样例
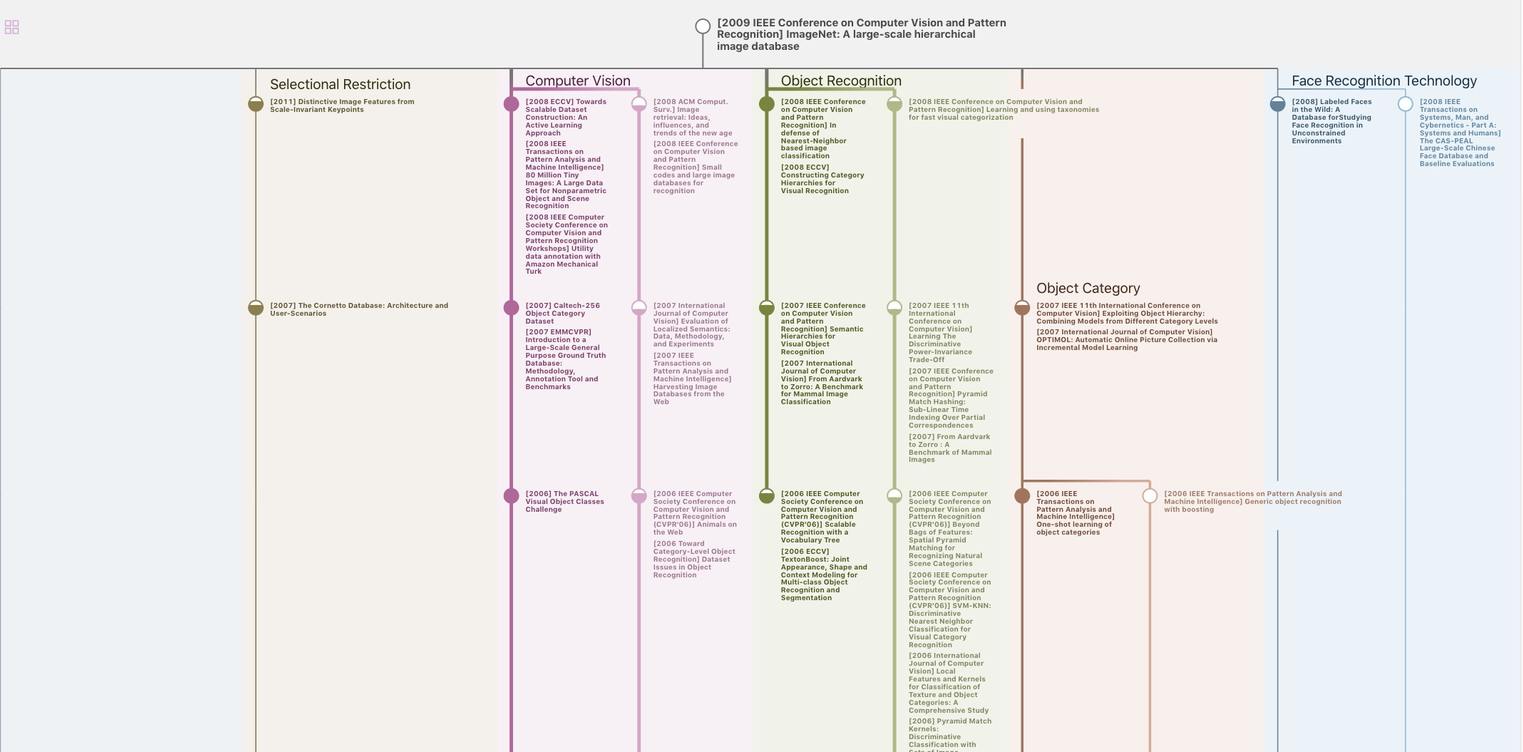
生成溯源树,研究论文发展脉络
Chat Paper
正在生成论文摘要