Health Status Assessment for HDDs Based on Bi-LSTM and N-Dimensional Similarity Metric
DATABASES THEORY AND APPLICATIONS, ADC 2023(2024)
摘要
In order to reduce the economic losses caused by hard disk failures, researchers have proposed various statistical and machine learning methods based on Self-Monitoring Analysis and Reporting Technology (SMART) attributes. Predicting hard drive health using SMART attributes, as proposed by previous methods, is effective for adopting different passive fault tolerance mechanisms in advance. Despite the effectiveness of these methods, there are still significant limitations. Specifically, these methods define health status according to the remaining time before it breaks down. However, they ignore changes in SMART features that reflect deteriorating disk health. In this paper, we propose an N-dimensional similarity metric to evaluate the health of HDDs, which acts on both SMART attributes and time-to-failure of HDDs. In addition, we use hypothesis test to eliminate abnormal data and propose a Bidirectional LSTM (Bi-LSTM) based model with weighted categorical cross-entropy loss. Experiments on the Backblaze and Baidu datasets show that our method obtains reasonably accurate health status assessments and outperforms previous methods. Code is available at https://github.com/su26225/HDD-Health-Status.
更多查看译文
关键词
Hard disk failure,SMART attributes,Health status assessment,Euclidean distance,Bi-LSTM
AI 理解论文
溯源树
样例
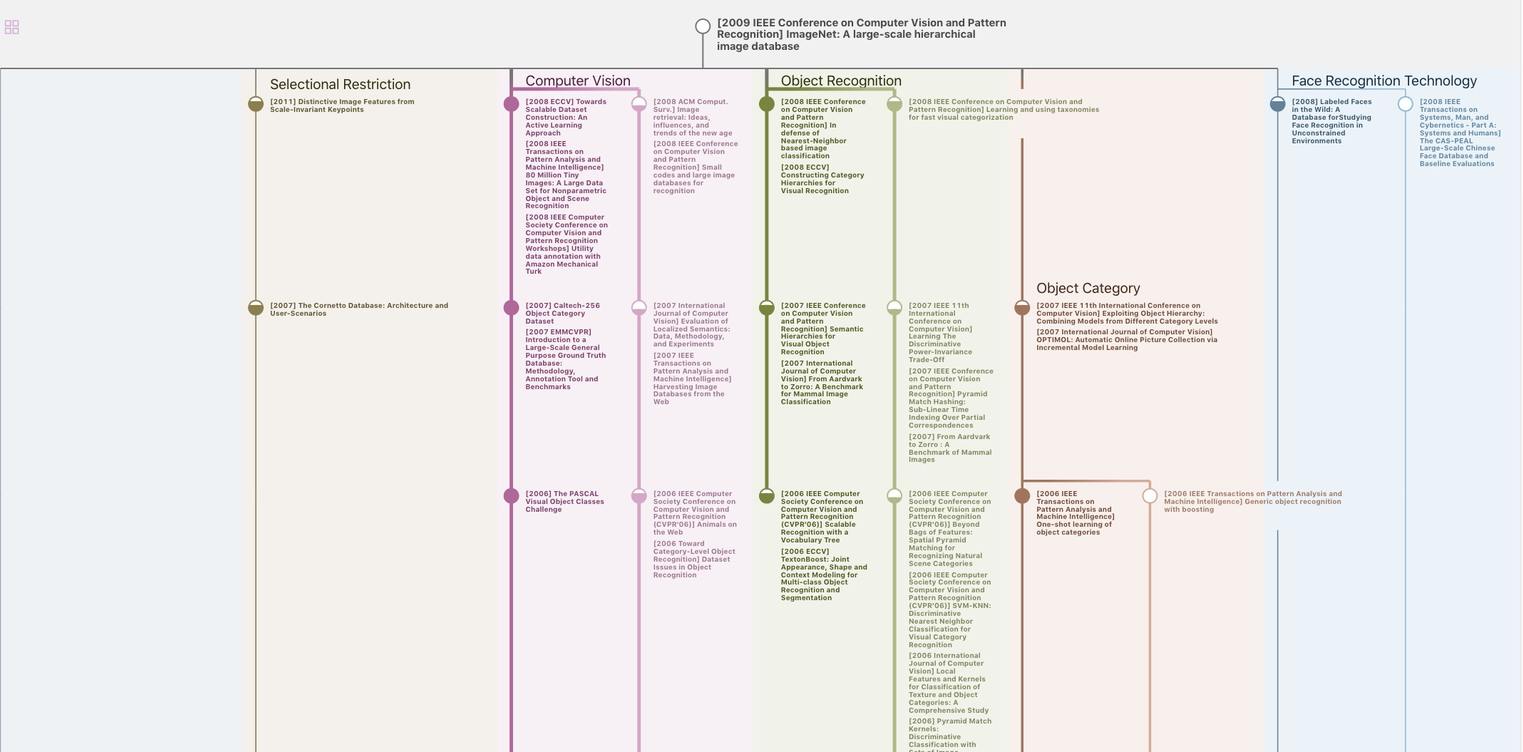
生成溯源树,研究论文发展脉络
Chat Paper
正在生成论文摘要