RattlesnakeJake: A Fast and Accurate Pre-alignment Filter Suitable for Computation-in-Memory.
Embedded Computer Systems: Architectures, Modeling, and Simulation: 23rd International Conference, SAMOS 2023, Samos, Greece, July 2–6, 2023, Proceedings(2023)
摘要
Significant improvements in pre-alignment filter accuracy have shifted the execution bottleneck of short-read sequence alignment to the filtering step for many genomics datasets. Current pre-alignment filters move data from memory to the processing units, and when rejection is determined, this results in wasted energy and time. This paper presents RattlesnakeJake, a hardware/software co-designed accelerator that speeds up and reduces the energy consumption of pre-alignment filtering and hence sequence alignment. RattlesnakeJake achieves this by (1) proposing a lightweight and hardware-friendly filtering algorithm, (2) adopting the Computation-In-Memory paradigm to avoid unnecessary data movement, and (3) exploiting resistive memories (memristors) to perform the low-level operations required by the proposed algorithm. Our preliminary results for RattlesnakeJake show an accuracy at the state-of-the-art (SotA) level and a significant improvement in the execution time of sequence alignment, irrespective of the evaluated dataset. The improvement for filtering varies from dataset to dataset and goes up to ∼ 7 × and ∼ 80 × , compared to SotA accelerators on GPU and CPU, respectively.
更多查看译文
AI 理解论文
溯源树
样例
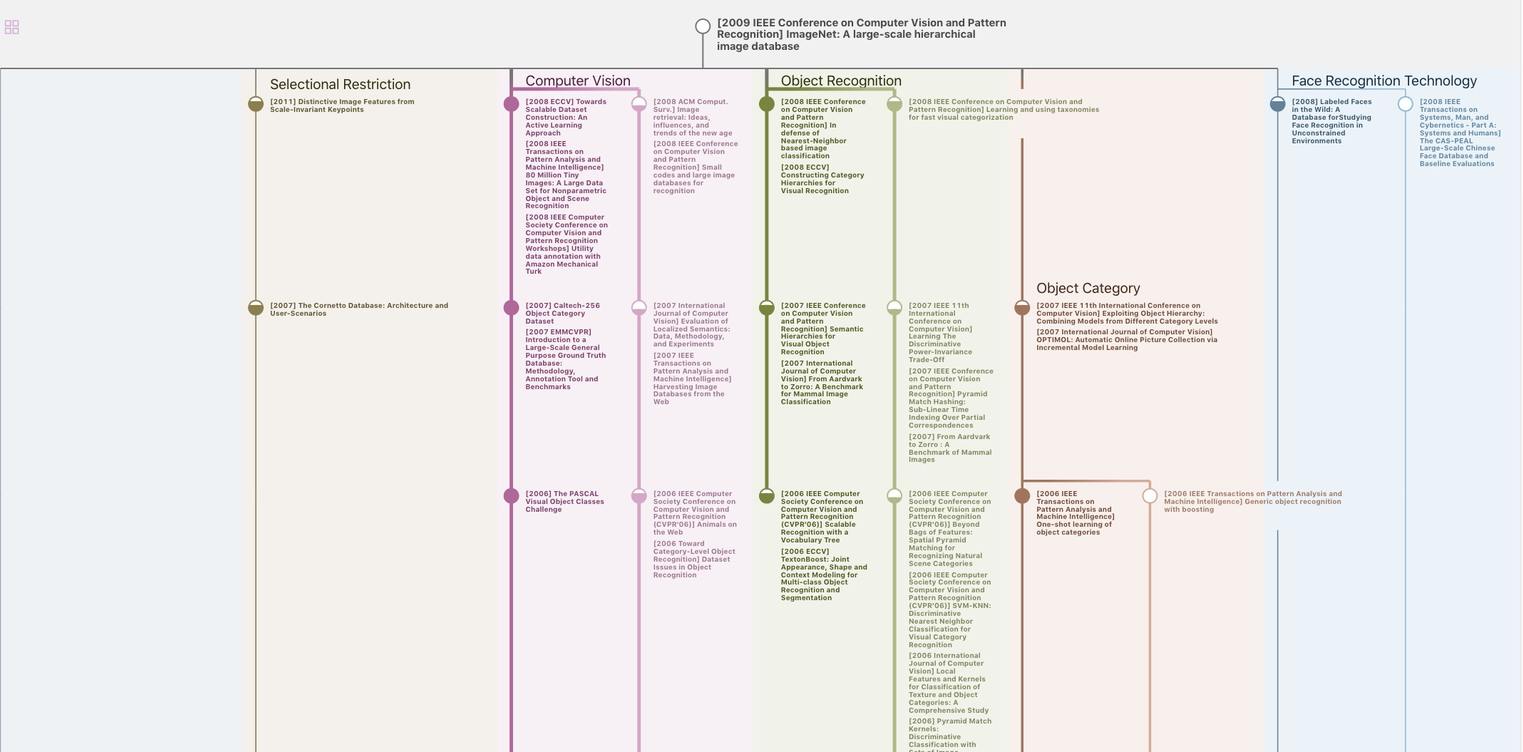
生成溯源树,研究论文发展脉络
Chat Paper
正在生成论文摘要