A comprehensive review and evaluation of graph neural networks for non-coding RNA and complex disease associations
BRIEFINGS IN BIOINFORMATICS(2023)
摘要
Non-coding RNAs (ncRNAs) play a critical role in the occurrence and development of numerous human diseases. Consequently, studying the associations between ncRNAs and diseases has garnered significant attention from researchers in recent years. Various computational methods have been proposed to explore ncRNA-disease relationships, with Graph Neural Network (GNN) emerging as a state-of-the-art approach for ncRNA-disease association prediction. In this survey, we present a comprehensive review of GNN-based models for ncRNA-disease associations. Firstly, we provide a detailed introduction to ncRNAs and GNNs. Next, we delve into the motivations behind adopting GNNs for predicting ncRNA-disease associations, focusing on data structure, high-order connectivity in graphs and sparse supervision signals. Subsequently, we analyze the challenges associated with using GNNs in predicting ncRNA-disease associations, covering graph construction, feature propagation and aggregation, and model optimization. We then present a detailed summary and performance evaluation of existing GNN-based models in the context of ncRNA-disease associations. Lastly, we explore potential future research directions in this rapidly evolving field. This survey serves as a valuable resource for researchers interested in leveraging GNNs to uncover the complex relationships between ncRNAs and diseases.
更多查看译文
关键词
non-coding RNA,disease associations,computational methods,graph neural network
AI 理解论文
溯源树
样例
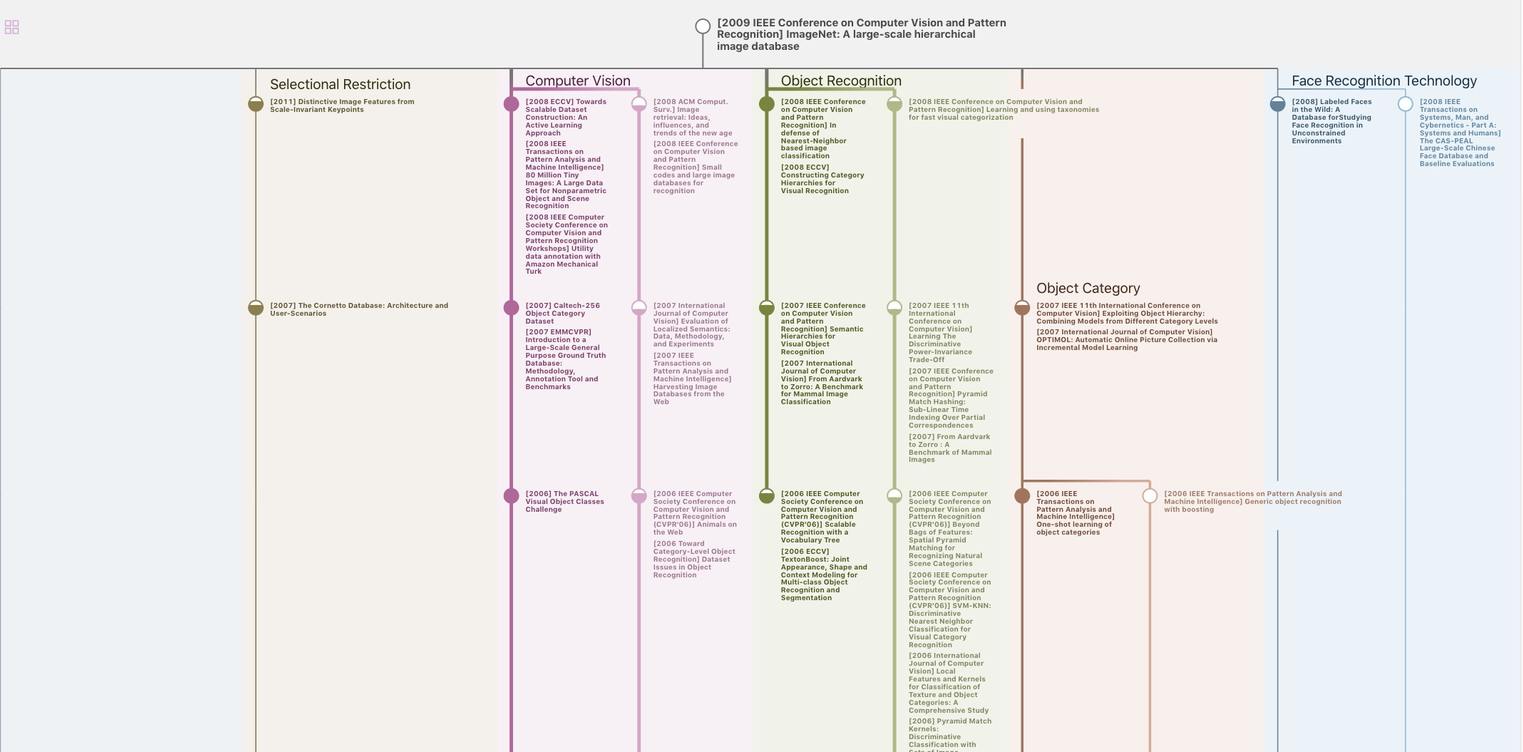
生成溯源树,研究论文发展脉络
Chat Paper
正在生成论文摘要