Bearing Fault Diagnosis and Interpretation Based on 2D Images and Convolutional Neural Network
2023 ASIA PACIFIC SIGNAL AND INFORMATION PROCESSING ASSOCIATION ANNUAL SUMMIT AND CONFERENCE, APSIPA ASC(2023)
摘要
In intelligent manufacturing, fault diagnosis of rotating machinery is indeed a critical and complex task. Most of the current research only classifies faults without considering the interpretability of classification. To fill this gap, inspired by the interpretable technology of computer vision, we propose 2DImages-CNN, a method combining 2D images and a convolutional neural network (CNN) for mechanical fault diagnosis and interpretation. First, we transform time series signals into distinct 2D images through two techniques: the Gramian angular field (GAF) and the Wavelet transform (WT). Then, we input 2D images into the ResNet34 model for fault recognition. We conducted experiments using transfer learning on the Case Western Reserve University (CWRU) bearing dataset. The results provide compelling evidence that, when compared to renowned methods, the 2DImages-CNN method achieves superior fault recognition accuracy. Finally, we use gradient-weighted class activation mapping (Grad-CAM) to interpret deep fault features. The results show that, for GAF images, the network shows a strong degree of activation in the area with large signal fluctuations. For WT images, the network shows a strong degree of activation in the area with large amplitude.
更多查看译文
关键词
Convolutional Neural Network (CNN),Fault Diagnosis,Gramian Angular Field (GAF),Wavelet Transform (WT),Interpretation
AI 理解论文
溯源树
样例
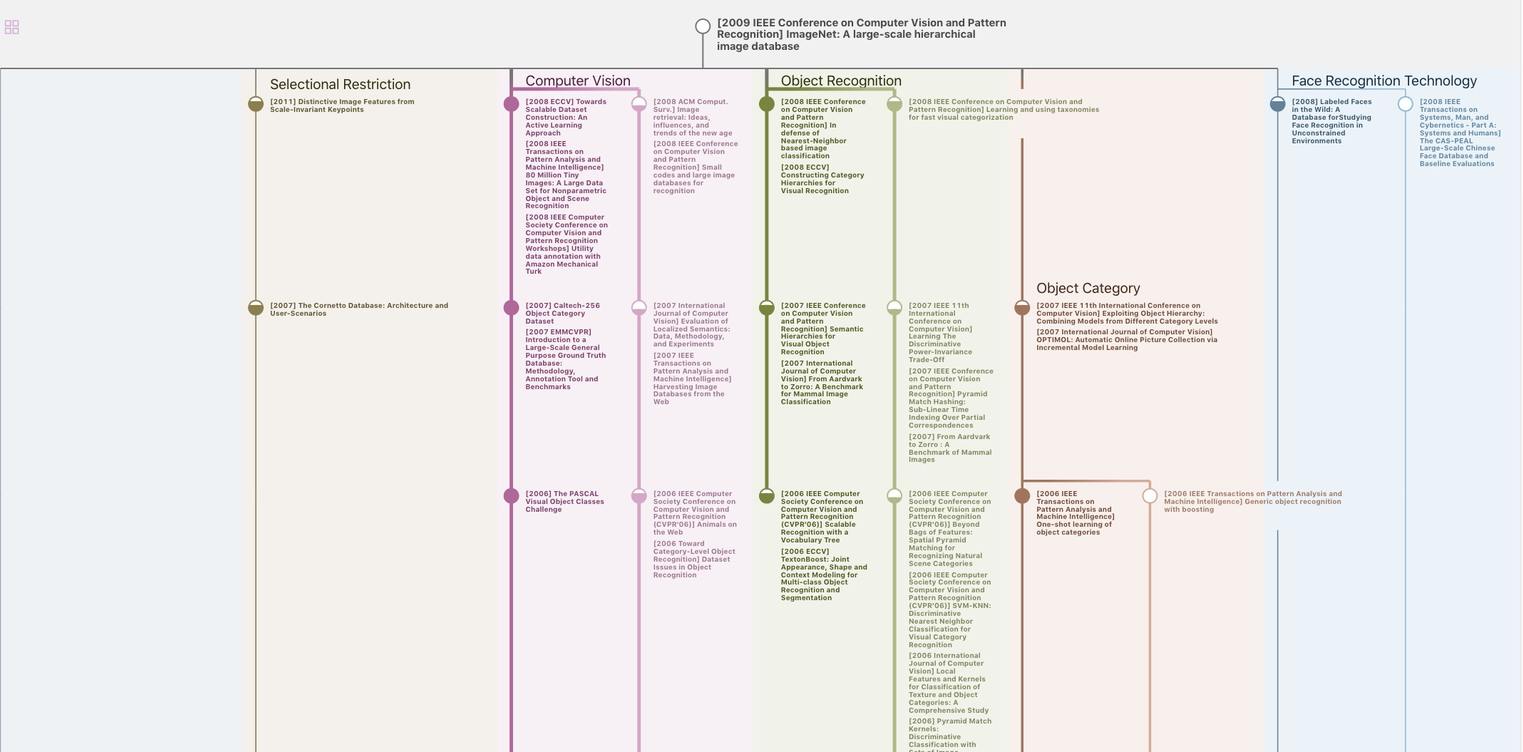
生成溯源树,研究论文发展脉络
Chat Paper
正在生成论文摘要