Influence of the Carrier–Envelope Phase on the Generation of the Multioctave Supercontinuum and Ultrashort Pulses in Antiresonant Hollow Waveguides
JETP Letters Journal of Experimental And Theoretical Physics Letters(2023)
摘要
The influence of the carrier–envelope phase on the spectrum of the supercontinuum and on the characteristics of ultrashort pulses, which are formed by the nonlinear optical transformation of pump pulses in an argon-filled antiresonant hollow waveguide has been demonstrated. The experimental and theoretical analysis has shown that the soliton self-compression of pump radiation with a central wavelength of about 2 μm forms a pulse with a duration of nearly one optical cycle and with a spectrum broadened to the region of 400‒800 nm, where interference with the broadband third harmonic generated by the same pulse is observed. The interference pattern is sensitive to the carrier–envelope phase of the laser pulse. The analysis of the interference pattern provides information on the difference of the spectral phases of the soliton and third harmonic in the spectral range wider than an octave and allows one to control the duration of pulses formed in the process of soliton self-compression.
更多查看译文
关键词
Ultrafast Laser Pulses
AI 理解论文
溯源树
样例
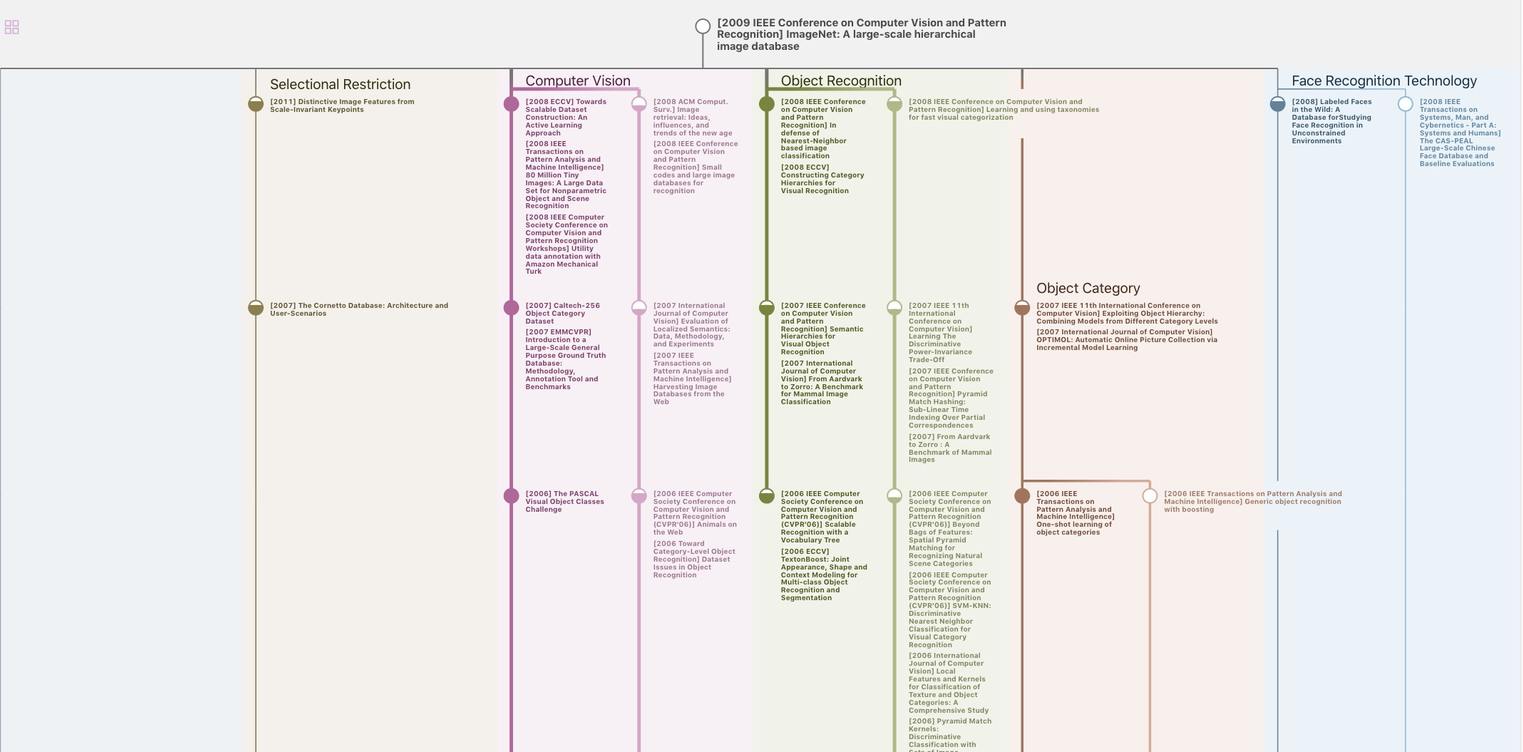
生成溯源树,研究论文发展脉络
Chat Paper
正在生成论文摘要