Measuring Five Accountable Talk Moves to Improve Instruction at Scale.
CoRR(2023)
摘要
Providing consistent, individualized feedback to teachers on their
instruction can improve student learning outcomes. Such feedback can especially
benefit novice instructors who teach on online platforms and have limited
access to instructional training. To build scalable measures of instruction, we
fine-tune RoBERTa and GPT models to identify five instructional talk moves
inspired by accountable talk theory: adding on, connecting, eliciting, probing
and revoicing students' ideas. We fine-tune these models on a newly annotated
dataset of 2500 instructor utterances derived from transcripts of small group
instruction in an online computer science course, Code in Place. Although we
find that GPT-3 consistently outperforms RoBERTa in terms of precision, its
recall varies significantly. We correlate the instructors' use of each talk
move with indicators of student engagement and satisfaction, including
students' section attendance, section ratings, and assignment completion rates.
We find that using talk moves generally correlates positively with student
outcomes, and connecting student ideas has the largest positive impact. These
results corroborate previous research on the effectiveness of accountable talk
moves and provide exciting avenues for using these models to provide
instructors with useful, scalable feedback.
更多查看译文
AI 理解论文
溯源树
样例
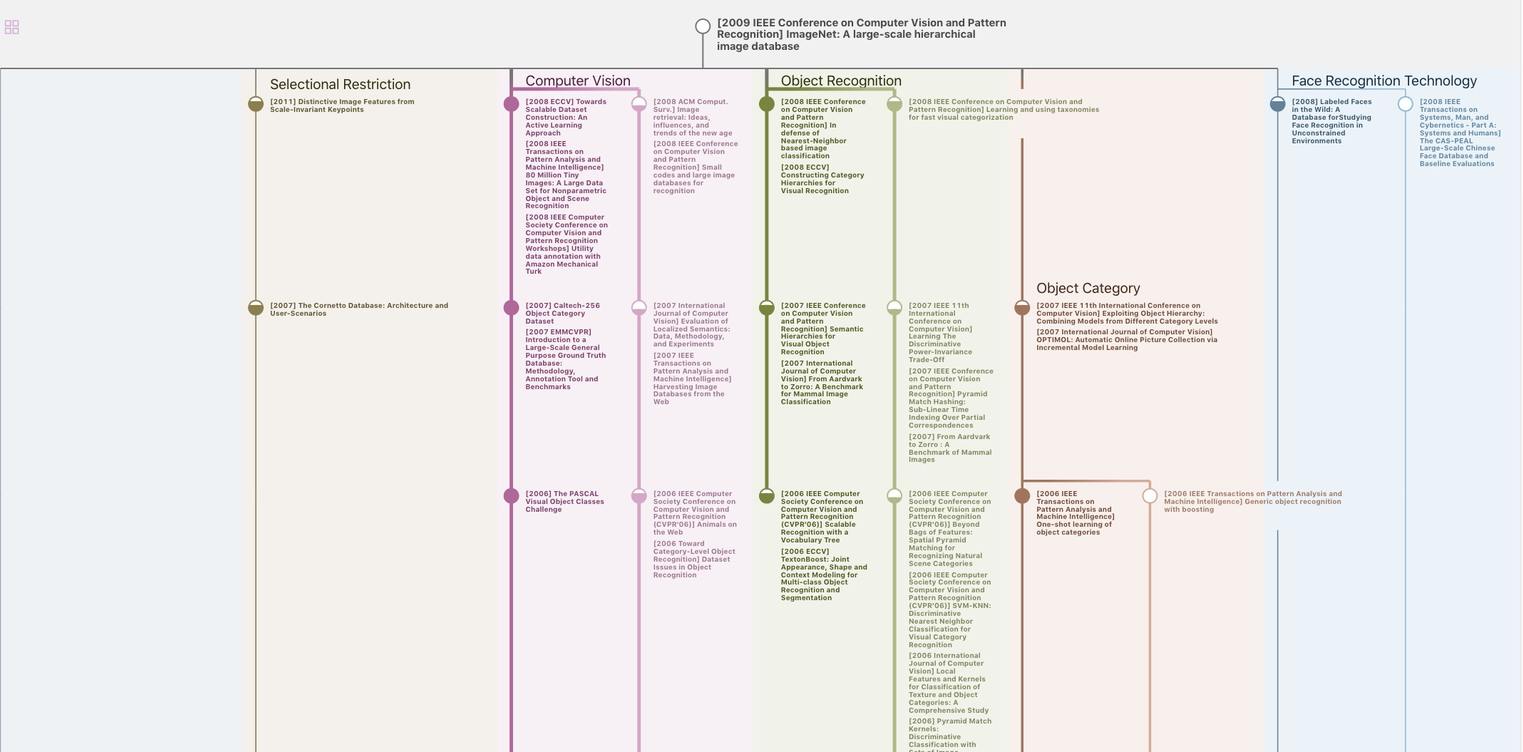
生成溯源树,研究论文发展脉络
Chat Paper
正在生成论文摘要