Corrected Sinus Node Recovery Time As a Screening Test in Patients with Typical Atrial Flutter Post-Ablation to Predict Pacemaker Implantation.
Deleted Journal(2024)
摘要
Objective: The objective of the study was to establish the prognostic value of CSNRT regarding the necessity for pacemaker implantation in patients with atrial flutter (AFL) post-ablation. Methods: This prospective cohort study, conducted at the Na- tional Institute of Cardiology "Ignacio Chavez" in Mexico City, assessed patients who had undergone ablation procedures to correct AFL, posterior to which an autonomic blockade was performed, and CSNRT was measured. Results: The sample for this investigation was 40 patients. These were subdivided into two study groups depending on their requirement of pacemaker implant post-ablation (Pacemaker P, No Pacemaker NP). Sinus node (SN) dysfunction was diagnosed in 13 (32.5%) of the 40 participants, 10 (71.43%) of which required a pacemaker implant, while only 4 participants (28.57%) with normal SN func- tion required pacemakers. Ten out of the 14 patients (71.43%) who required a pacemaker had an elevated CSNRT > 500 ms (p <= 0.01). Post-ablation CSNRT mean was 383.54 ms +/- 67.96 ms in the NP group versus 1972.57 ms +/- 3423.56 ms in the P group. Furthermore, SN pause in the P group had a mean of 1.86 s +/- 0.96 s versus the NP group with 1.196 s +/- 0.52 s. Conclusion: CSNRT has the potential to be a quantitative prognostic tool for the assessment of future pacemaker implants in patients with AFL post-ablation. This could aid in the timely diagnosis of sinus node dysfunction, which could, in the long run, result in the reduction of cardiac functional capacity loss due to cardiac remodeling.
更多查看译文
关键词
CSNRT,Atrial flutter,Pacemaker,Screening,Post-ablation
AI 理解论文
溯源树
样例
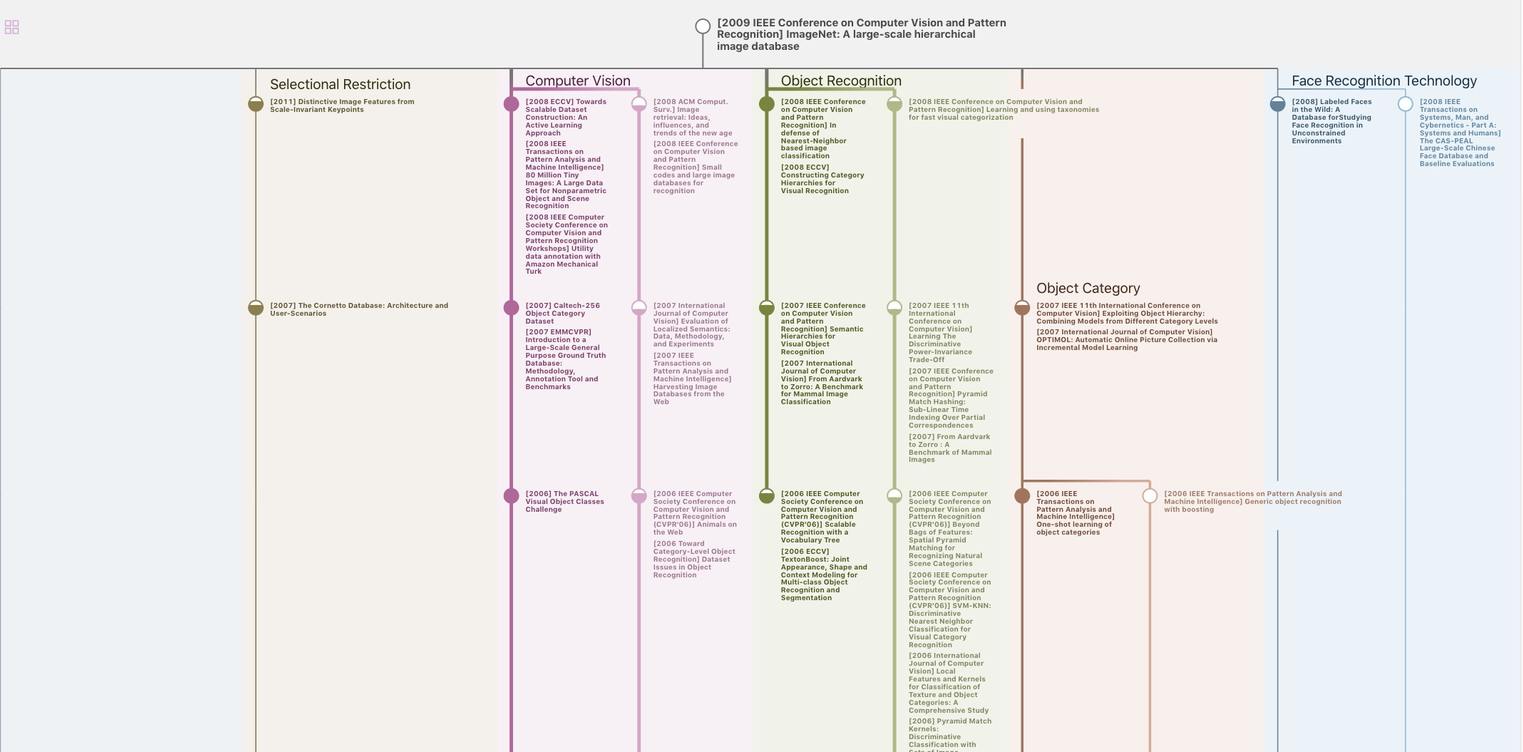
生成溯源树,研究论文发展脉络
Chat Paper
正在生成论文摘要