A Density Evolution Framework for Recovery of Covariance and Causal Graphs from Compressed Measurements
2023 59th Annual Allerton Conference on Communication, Control, and Computing (Allerton)(2023)
摘要
In this paper, we propose a general framework for designing sensing matrix A ∈ ℝ
d×p
, for estimation of sparse covariance matrix from compressed measurements of the form y = Ax + n, where y, n ∈ ℝ
d
, and x ∈ ℝ
p
. By viewing covariance recovery as inference over factor graphs via message passing algorithm, ideas from coding theory, such as Density Evolution (DE), are leveraged to construct a framework for the design of the sensing matrix. The proposed framework can handle both (1) regular sensing, i.e., equal importance is given to all entries of the covariance, and (2) preferential sensing, i.e., higher importance is given to a part of the covariance matrix. Through experiments, we show that the sensing matrix designed via density evolution can match the state-of-the-art for covariance recovery in the regular sensing paradigm and attain improved performance in the preferential sensing regime. Additionally, we study the feasibility of causal graph structure recovery using the estimated covariance matrix obtained from the compressed measurements.
更多查看译文
AI 理解论文
溯源树
样例
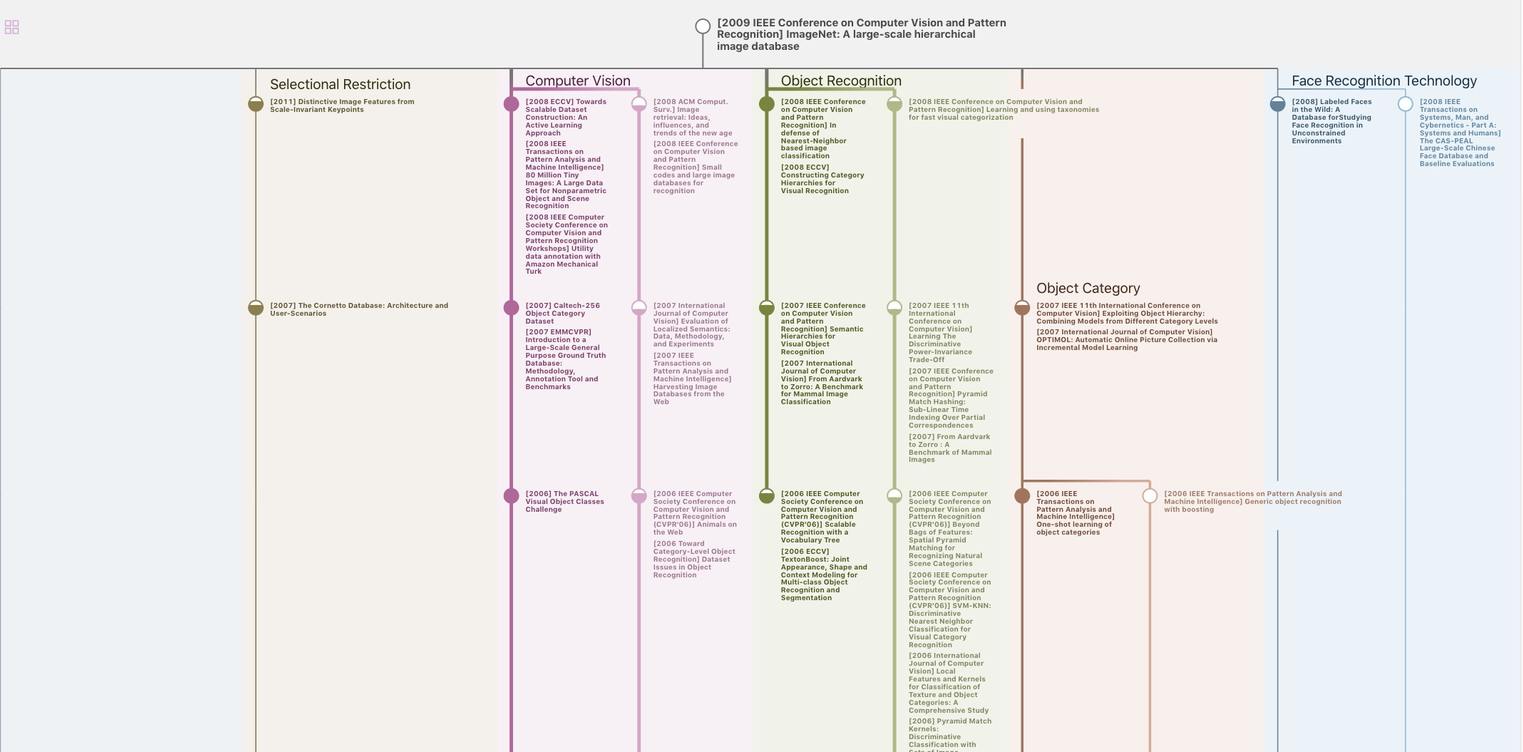
生成溯源树,研究论文发展脉络
Chat Paper
正在生成论文摘要