On How to Unravel Bone Microscale Phenomena: A Mask-Guided Attention SR-microCT Image Classification Approach
2023 IEEE EMBS INTERNATIONAL CONFERENCE ON BIOMEDICAL AND HEALTH INFORMATICS, BHI(2023)
摘要
The global increase in elderly individuals has led to a rise in fragility fractures and chronic aging-related diseases, including osteoporosis. In this context, Deep Learning (DL) offers the potential to analyze bone images to aid researchers and clinicians in studying its health starting from the microscale. Previous studies demonstrate the effectiveness of DL in segmenting lacunae and classifying bone tissue micro-states from Synchrotron-Radiation micro-Computed Tomography (SR-microCT) images. However, the generalizability of these models, the laborious work in labeling tiny structures in high-dimensional images, and the low inter-class variance in SR-microCT images remain a concern. To fill this void, this paper proposes a Mask-Guided Attention (MGA) approach that combines semi-supervised learning lacunae segmentation and attention methods for healthy and osteoporotic SR-microCT image classification. In particular, semi-supervised learning aims at reducing the number of labeled images required during segmentation. At the same time, the MGA approach exploits the pseudo-labels predicted to focus the network's attention on the informative lacunar structures. Our strategy allows achieving up to 5.64% and 12.17% accuracy improvements over de-facto lacunae image segmentation and image classification methods, as well as more interpretable results. Clinical relevance-The proposed MGA approach could enhance the understanding of bone microscale phenomena by exploiting SR-microCT images, supporting the study and the diagnosis of osteoporosis in individuals.
更多查看译文
AI 理解论文
溯源树
样例
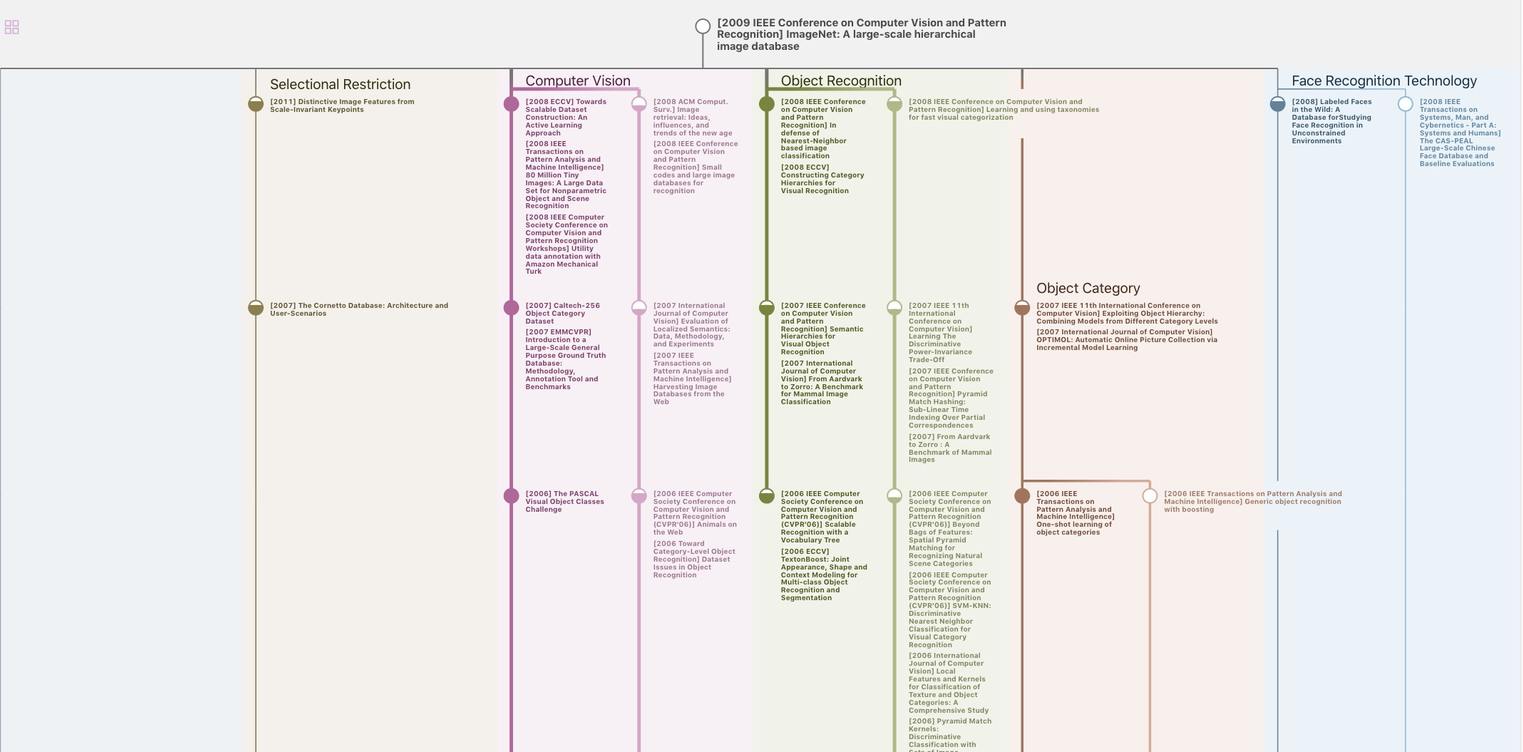
生成溯源树,研究论文发展脉络
Chat Paper
正在生成论文摘要