Mixed Attention Network for Cross-domain Sequential Recommendation
PROCEEDINGS OF THE 17TH ACM INTERNATIONAL CONFERENCE ON WEB SEARCH AND DATA MINING, WSDM 2024(2024)
摘要
In modern recommender systems, sequential recommendation leverages chronological user behaviors to make effective next-item suggestions, which suffers from data sparsity issues, especially for new users. One promising line of work is the cross-domain recommendation, which trains models with data across multiple domains to improve the performance in data-scarce domains. Recent proposed cross-domain sequential recommendation models such as PiNet and DASL have a common drawback relying heavily on overlapped users in different domains, which limits their usage in practical recommender systems. In this paper, we propose a Mixed Attention Network (MAN) with local and global attention modules to extract the domain-specific and cross-domain information. Firstly, we propose a local/global encoding layer to capture the domainspecific/cross-domain sequential pattern. Then we propose a mixed attention layer with item similarity attention, sequence-fusion attention, and group-prototype attention to capture the local/global item similarity, fuse the local/global item sequence, and extract the user groups across different domains, respectively. Finally, we propose a local/global prediction layer to further evolve and combine the domain-specific and cross-domain interests. Experimental results on two real-world datasets (each with two domains) demonstrate the superiority of our proposed model. Further study also illustrates that our proposed method and components are modelagnostic and effective, respectively. The code and data are available at https://github.com/tsinghua- fib- lab/WSDM24-MAN.
更多查看译文
关键词
Cross-domain Sequential Recommendation,Mixed Attention Network,Recommender Systems
AI 理解论文
溯源树
样例
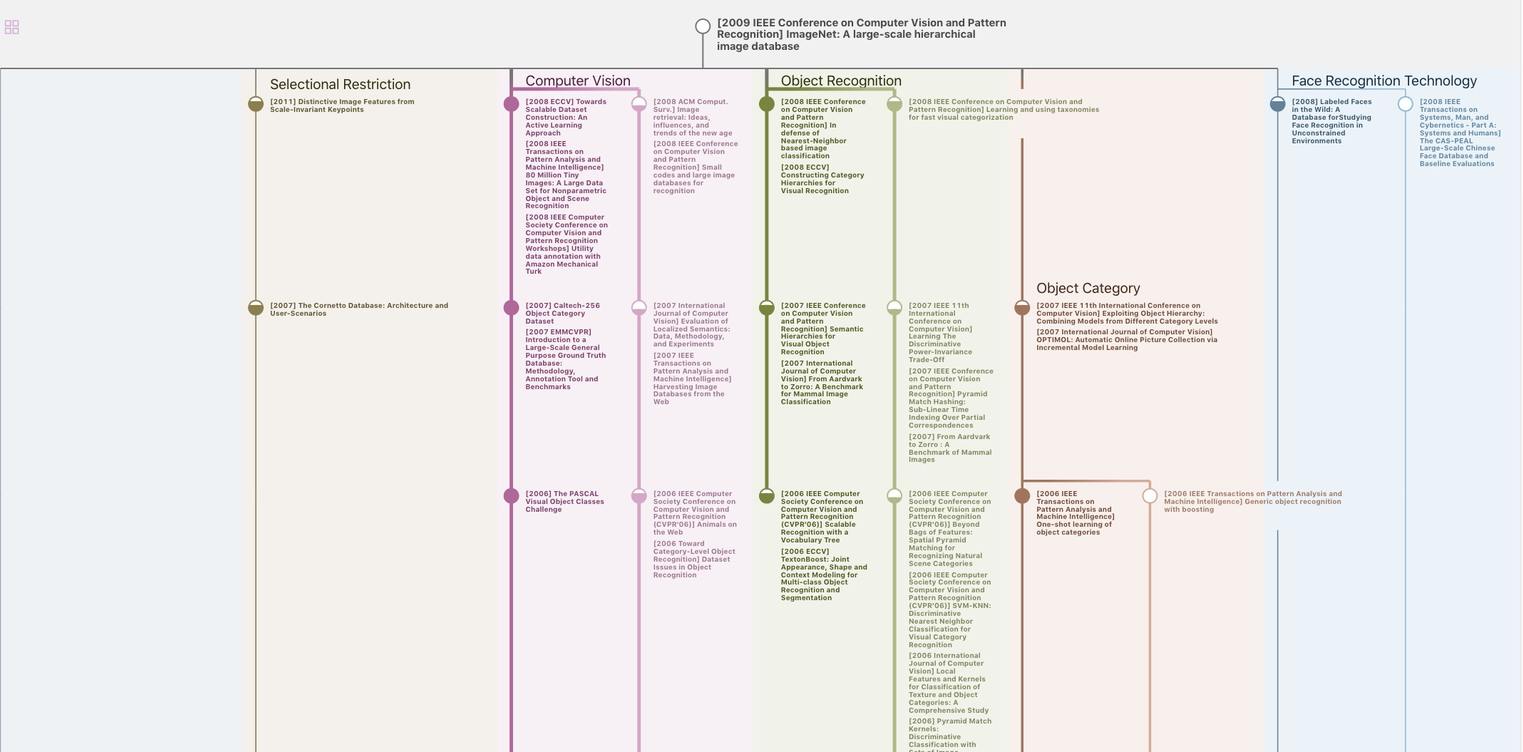
生成溯源树,研究论文发展脉络
Chat Paper
正在生成论文摘要