The Impact of Adversarial Node Placement in Decentralized Federated Learning Networks
ICC 2024 - IEEE International Conference on Communications(2024)
摘要
As Federated Learning (FL) grows in popularity, new decentralized frameworksare becoming widespread. These frameworks leverage the benefits ofdecentralized environments to enable fast and energy-efficient inter-devicecommunication. However, this growing popularity also intensifies the need forrobust security measures. While existing research has explored various aspectsof FL security, the role of adversarial node placement in decentralizednetworks remains largely unexplored. This paper addresses this gap by analyzingthe performance of decentralized FL for various adversarial placementstrategies when adversaries can jointly coordinate their placement within anetwork. We establish two baseline strategies for placing adversarial node:random placement and network centrality-based placement. Building on thisfoundation, we propose a novel attack algorithm that prioritizes adversarialspread over adversarial centrality by maximizing the average network distancebetween adversaries. We show that the new attack algorithm significantlyimpacts key performance metrics such as testing accuracy, outperforming thebaseline frameworks by between 9findings provide valuable insights into the vulnerabilities of decentralized FLsystems, setting the stage for future research aimed at developing more secureand robust decentralized FL frameworks.
更多查看译文
关键词
Federated Learning,Node Placement,Impact Of Placement,Adversarial Nodes,Test Accuracy,Placement Strategy,Random Placement,Key Performance Metrics,Federated Learning Framework,Data Distribution,Convolutional Neural Network,Onset Time,Network Topology,Network Size,Graph Structure,Performance Gap,Local Dataset,Adversarial Attacks,Eigenvector Centrality,Breadth-first Search,Attack Strategy,Attack Performance,Federated Learning Model,Aggregation Procedure,Random Attack,Disparities In Performance,Baseline Algorithms,Degree Of Randomness
AI 理解论文
溯源树
样例
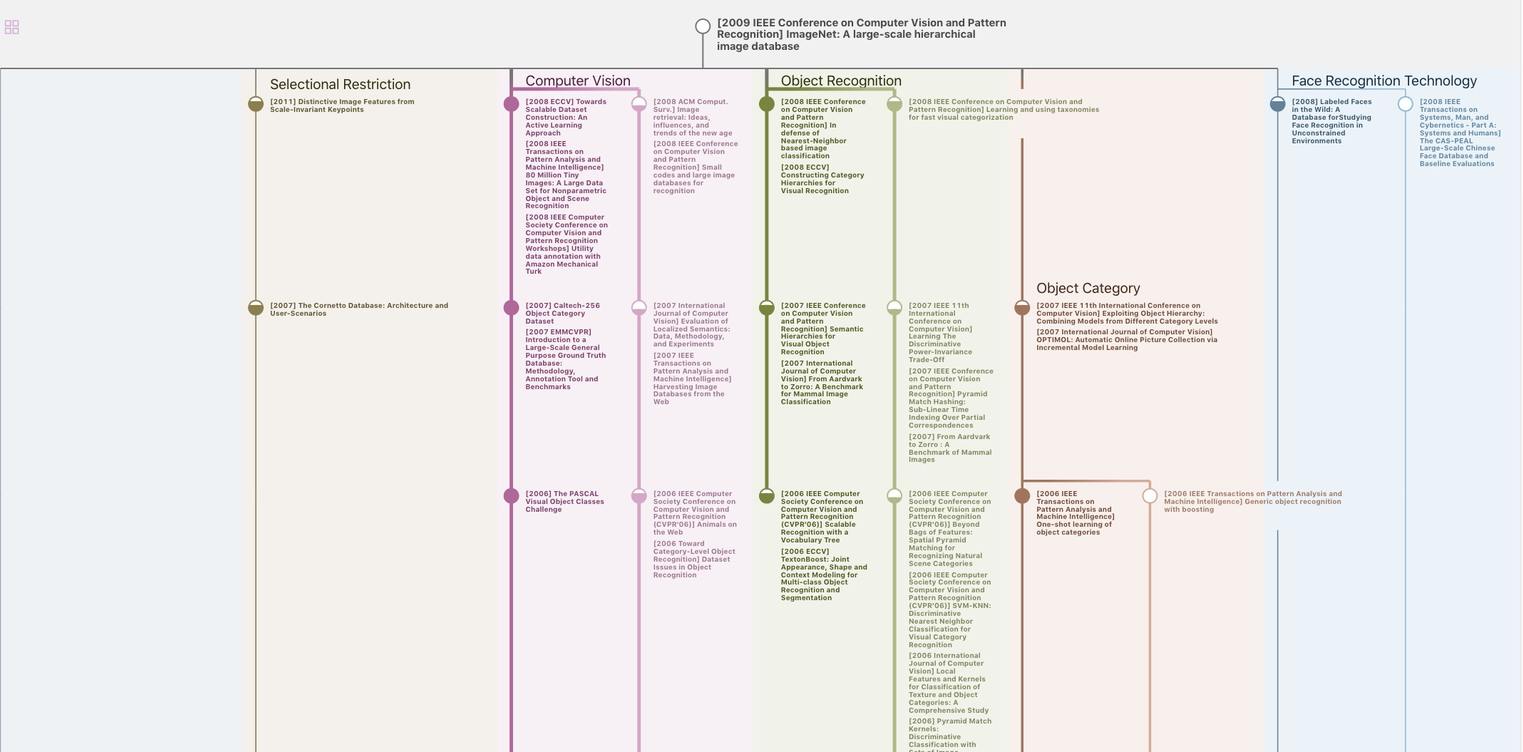
生成溯源树,研究论文发展脉络
Chat Paper
正在生成论文摘要