In Search of the Long-Tail: Systematic Generation of Long-Tail Inferential Knowledge via Logical Rule Guided Search
CoRR(2023)
摘要
State-of-the-art LLMs outperform humans on reasoning tasks such as Natural
Language Inference. Recent works evaluating LLMs note a marked performance drop
on input data from the low-probability distribution, i.e., the longtail.
Therefore, we focus on systematically generating statements involving long-tail
inferential knowledge for more effective evaluation of LLMs in the reasoning
space. We first propose a novel framework Logic-Induced- Knowledge-Search
(LINK) that generates factually correct and long-tail knowledge statements
grounded on symbolic rule templates; LINK effectively generates data in the
longtail distribution that zero-shot prompted LLMs are unable to reach, and
outperforms zero-shot GPT4 on factual correctness by 5
data generated by LINK to construct a dataset Logic-Induced-Long-Tail (LINT)
that can be used to evaluate downstream models on the long-tail distribution;
LINT contains 108K knowledge statements spanning four domains. We use LINT to
test LLMs on an entailment classification task and find that model performances
drop by as high as 5
distribution. Our work shows the utility of evaluating models in the long-tail
distribution, and calls for more research on generating evaluation data in the
long-tail distribution.
更多查看译文
关键词
Information Retrieval,Topic Modeling,Part-of-Speech Tagging,Syntax-based Translation Models,Language Modeling
AI 理解论文
溯源树
样例
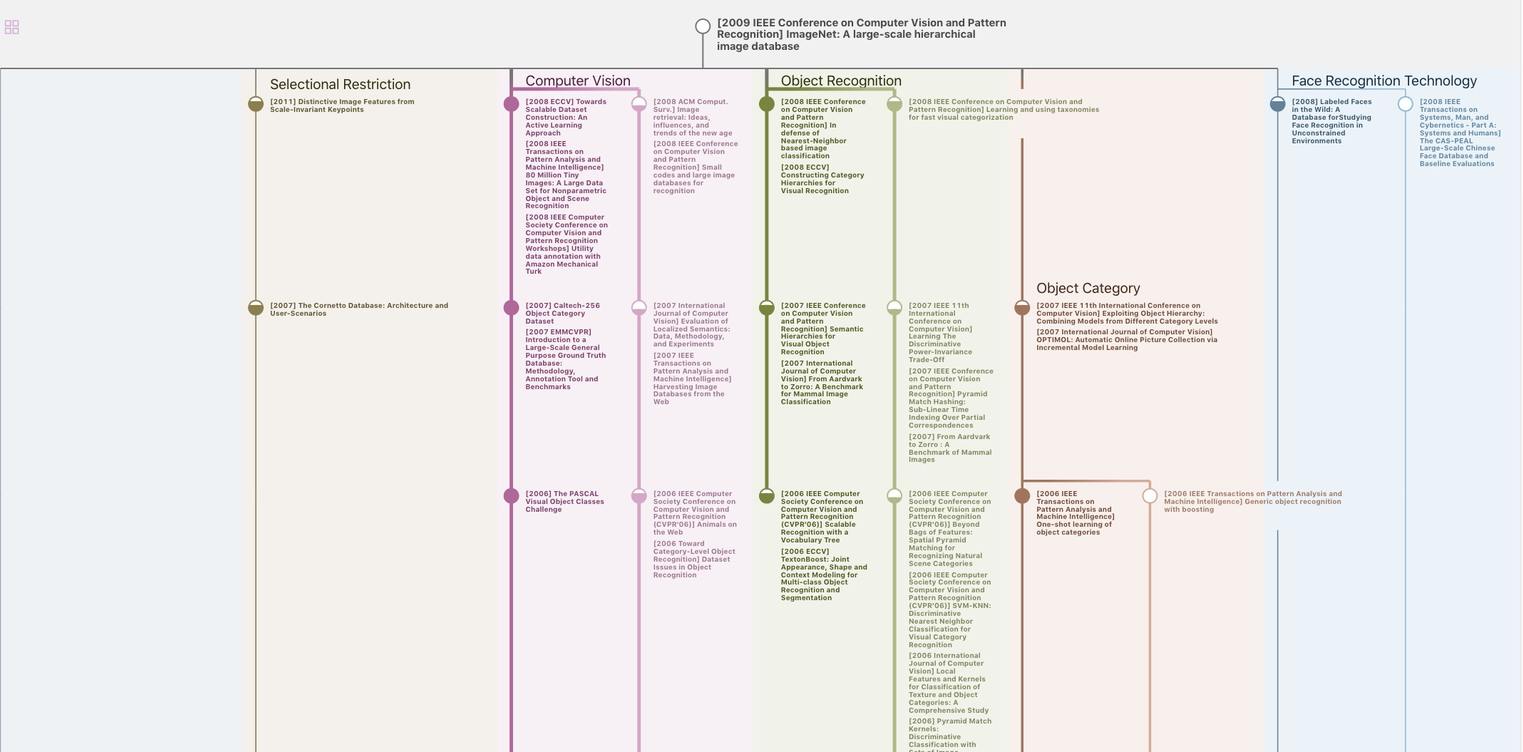
生成溯源树,研究论文发展脉络
Chat Paper
正在生成论文摘要