Assurance-driven Design of Machine Learning-based Functionality in an Aviation Systems Context
2023 IEEE/AIAA 42nd Digital Avionics Systems Conference (DASC)(2023)
摘要
We present an approach to the co-development of an aeronautical function integrating Machine Learning (ML) and its associated preliminary safety assurance case. Using an Autonomous Visual Landing (AVL) application as a running example, in which a Deep Neural Network (DNN) supports navigation state estimation, first we describe how we identify the contributions of ML-based functionality to system hazards. We then give the safety and ML requirements necessary for hazard mitigation, together with a candidate safety architecture aimed at mitigating the ML-induced functional insufficiencies. Thereafter, we present a structured argument embodying the rationale that provides confidence that the ML-based function will be safe for use. Collectively, these artifacts represent the core elements of a multi-viewpoint safety assurance case. We discuss how that concept is compatible with prevailing aerospace recommended practices for safety assessment, its utility to align design-time assurance considerations with operational safety needs, and how it can communicate the evidence necessary for safety assurance in an integrated way.
更多查看译文
关键词
Assurance Cases,Autonomy,Design Assurance,Machine Learning,Safety Cases,Visual Landing
AI 理解论文
溯源树
样例
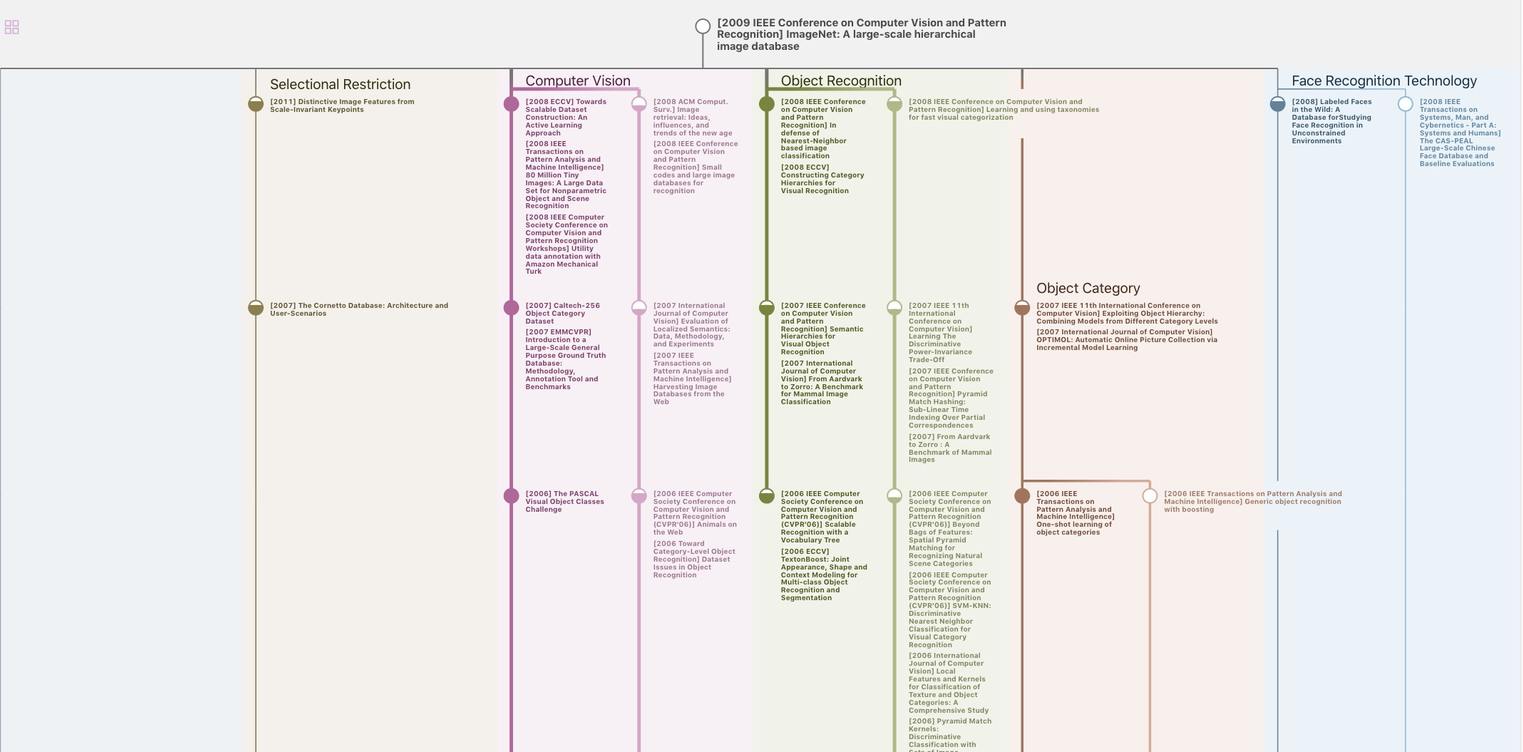
生成溯源树,研究论文发展脉络
Chat Paper
正在生成论文摘要