Convolutional Neural Network for Risk Assessment in Polycrystalline Alloy Structures Via Ultrasonic Testing
FATIGUE & FRACTURE OF ENGINEERING MATERIALS & STRUCTURES(2024)
Abstract
In the current state of the art of process industries/manufacturing technologies, computer-instrumented and computer-controlled autonomous techniques are necessary for damage diagnosis and prognosis in operating machinery. From this perspective, the paper addresses the issue of fatigue damage that is one of the most encountered sources of degradation in polycrystalline-alloy structures of machinery components. In this paper, the convolutional neural networks (CNNs) are applied to synergistic combinations of ultrasonic measurements and images from a confocal microscope (Alicona) to detect and evaluate the risk of fatigue damage. The database of the Alicona has been used to calibrate the ultrasonic database and to provide the ground truth for fatigue damage assessment. The results show that both the ultrasonic data and Alicona images are capable of classifying the fatigue damage into their respective classes with considerably high accuracy. However, the ultrasonic CNN model yields better accuracy than the Alicona CNN model by almost 9%.
MoreTranslated text
Key words
convolutional neural networks,damage classification,damage detection,fatigue damage,ultrasonic
AI Read Science
Must-Reading Tree
Example
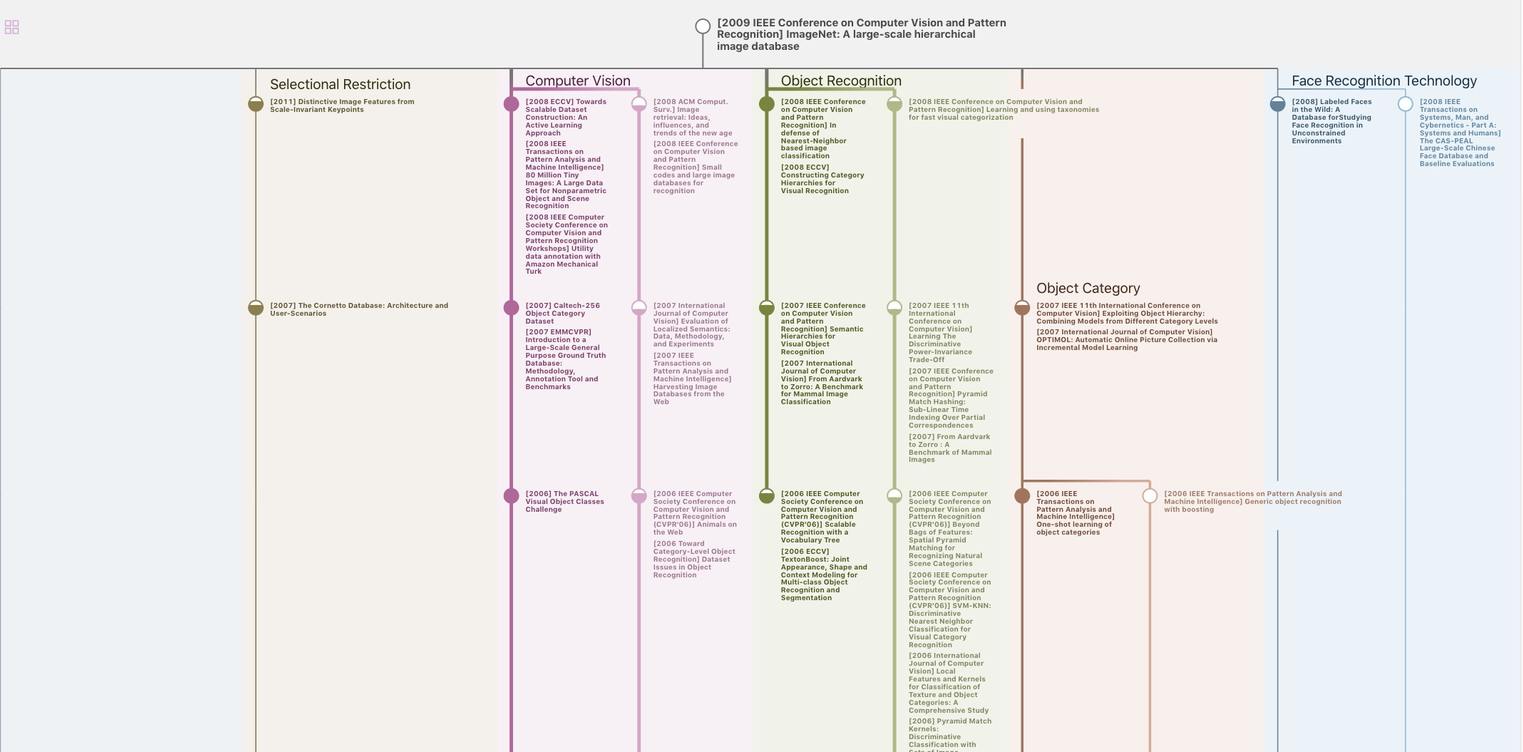
Generate MRT to find the research sequence of this paper
Chat Paper
Summary is being generated by the instructions you defined