A Dual-Path Supplemental Information Learning Architecture for Breast Cancer Ki-67 Status Prediction in T2w MRI
2023 IEEE INTERNATIONAL CONFERENCE ON MULTIMEDIA AND EXPO, ICME(2023)
摘要
In this paper, we propose a Dual-path Supplemental Information Learning Architecture (DSILA) for predicting breast cancer Ki-67 status based on T2-weighted (T2w) magnetic resonance imaging (MRI). DSILA consists of two components: 1) a transfer network with multi-scale feature selection strategy to obtain generic multi-scale features most relative to target, 2) a supplemental learning network with a large receptive field and channel-level attention to mine scenario-related semantic information. A regulation item - Aspect Overlap Loss (AOL), is further added to force the supplemental learning network to pay more attention to the regions overlooked by the transfer network. The experimental results tested on the collected T2w MRI breast cancer Ki-67 dataset show that DSILA outperforms state-of-the-art techniques among all adopted evaluation metrics, even achieving 0.85 in Area under the Receiver Operating Characteristic Curve (AUC).
更多查看译文
关键词
Healthcare, breast cancer, Ki-67 status, dual-path architecture, supplemental information, aspect overlap loss
AI 理解论文
溯源树
样例
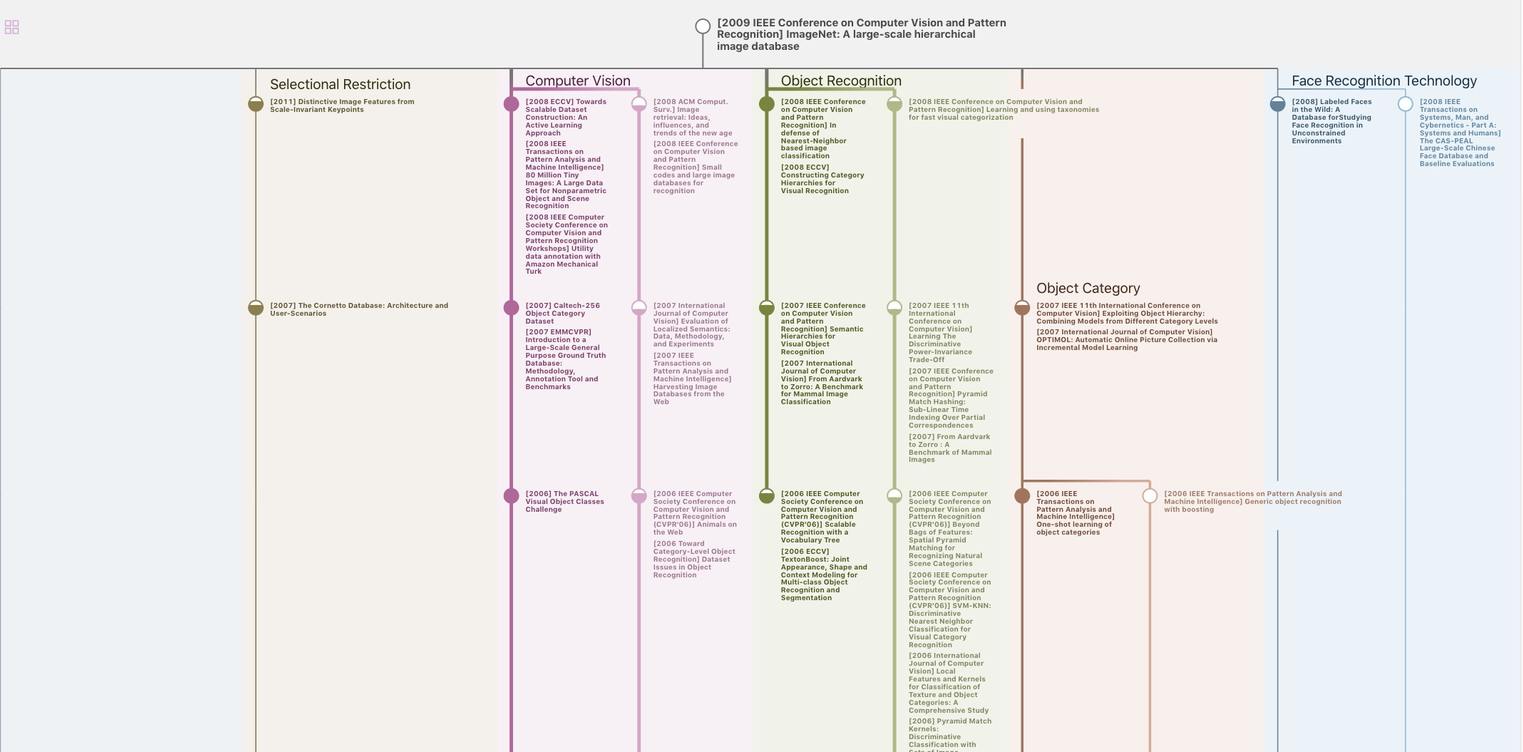
生成溯源树,研究论文发展脉络
Chat Paper
正在生成论文摘要