FeFET Reliability Modeling for In-Memory Computing: Challenges, Perspective, and Emerging Trends
IEEE TRANSACTIONS ON ELECTRON DEVICES(2024)
摘要
Ferroelectric FET (FeFET) is a singularly attractive emerging technology with a rich feature set. Boasting high versatility, it has already been implemented in a host of applications, like dense memory arrays and in-memory computing (IMC). Nevertheless, being an emerging technology, FeFETs are faced with reliability concerns that warrant analysis and mitigation. As a first step, modeling the FeFET variability can help to ascertain its present applicability. For instance, binary neural networks (BNNs) are more error resilient than classical deep-neural networks implementations and thus, can utilize this emerging yet unreliable hardware. However, conventional machine learning (ML) still relies on large datasets for training that need to be processed many times by the algorithm, exposing the memory wall of the von Neumann architecture. This necessitates the pursuit of new computing paradigms, like hyperdimensional computing (HDC). HDC is an emerging brain-inspired ML algorithm that can learn from very little data, using pattern recognition and randomness as its core working principles. Thus, it is inherently robust against noise and errors in the employed computing hardware. Hence, the robust HDC and the efficient yet unreliable FeFET are fiercely symbiotic. In this work, we will cover the challenges of the emerging FeFET technology and how to model them on a device level. We will then make use of these models and inject them into applications to study their error resiliency. For this, we will use the aforementioned applications of BNNs and HDC, and showcase their response to the unreliable hardware.
更多查看译文
关键词
Binary neural network (BNN),ferroelectric (FE),ferroelectric FET (FeFET),hyperdimensional computing (HDC),multidomain,reliability,technology CAD (TCAD),variation
AI 理解论文
溯源树
样例
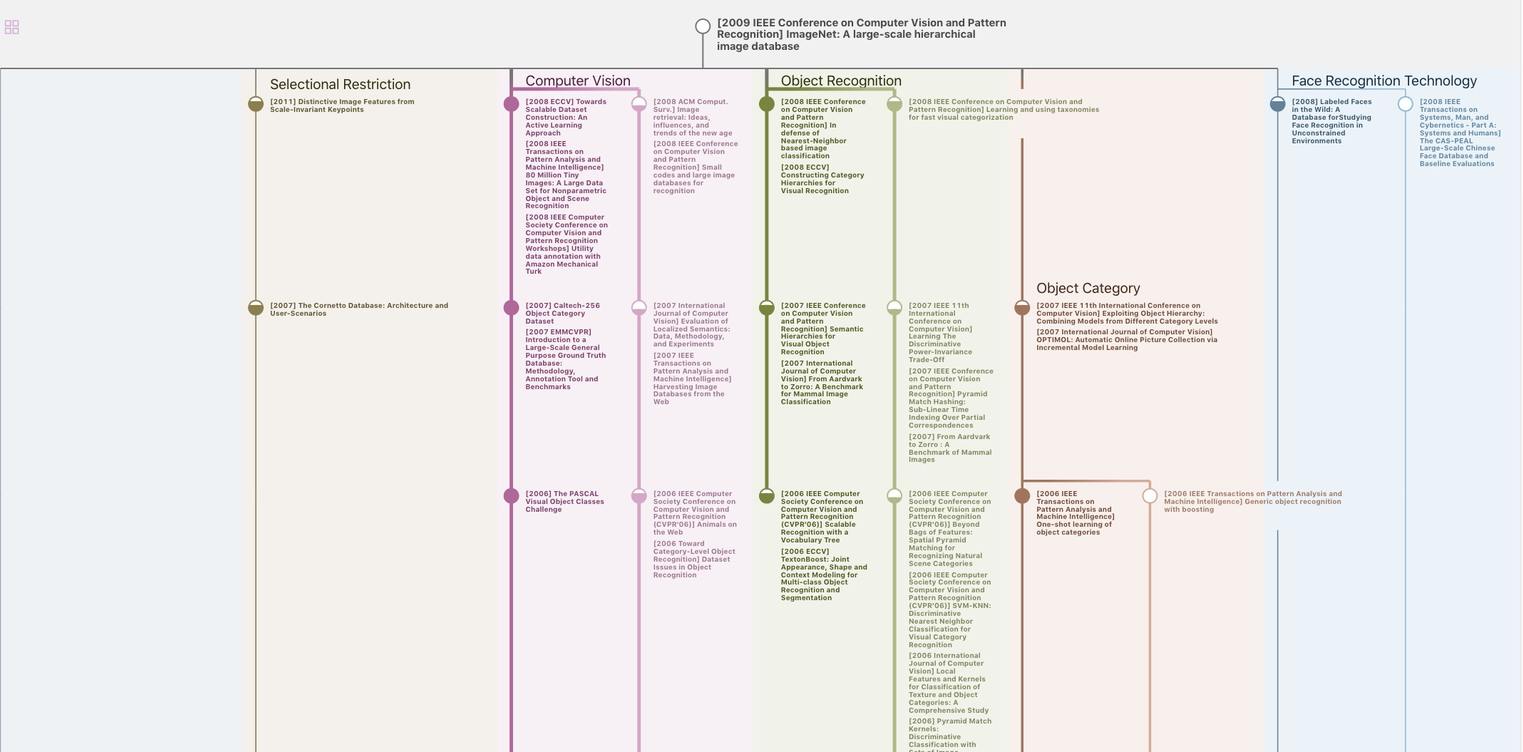
生成溯源树,研究论文发展脉络
Chat Paper
正在生成论文摘要