Objectively Scoring the Human-Likeness of Artificial Driver Models
Applied sciences(2023)
摘要
Several applications of artificially modeled drivers, such as autonomous vehicles (AVs) or surrounding traffic in driving simulations, aim to provide not only functional but also human-like behavior. The development of human-like AVs is expected to improve the interaction between AVs and humans in traffic, whereas, in a driving simulation, the objective is to create realistic replicas of real driving scenarios to investigate various research questions under safe and reproducible conditions. In urban traffic, driving behavior strongly depends on the situational context, which introduces new challenges not only for modeling but also for the evaluation of such models. However, current objective assessment strategies rarely consider situational context and human similarity, whereas subjective approaches are not suitable for iterative development processes. In this paper, we present a first attempt to make the plausibility and human-likeness of vehicles' trajectories objectively measurable. A multidimensional quality function is presented that incorporates various parameters characterizing human-like driving behavior and compares each of those parameters to human driving behavior under similar conditions. Among other things, our validation results show that the presented evaluation methodology is scalable to a wide range of situations has the ability to identify model weaknesses, and is able to reflect the way people distinguish between artificial and human behavior.
更多查看译文
关键词
traffic data,driver models,autonomous driving,driving simualtion,urban,human factors
AI 理解论文
溯源树
样例
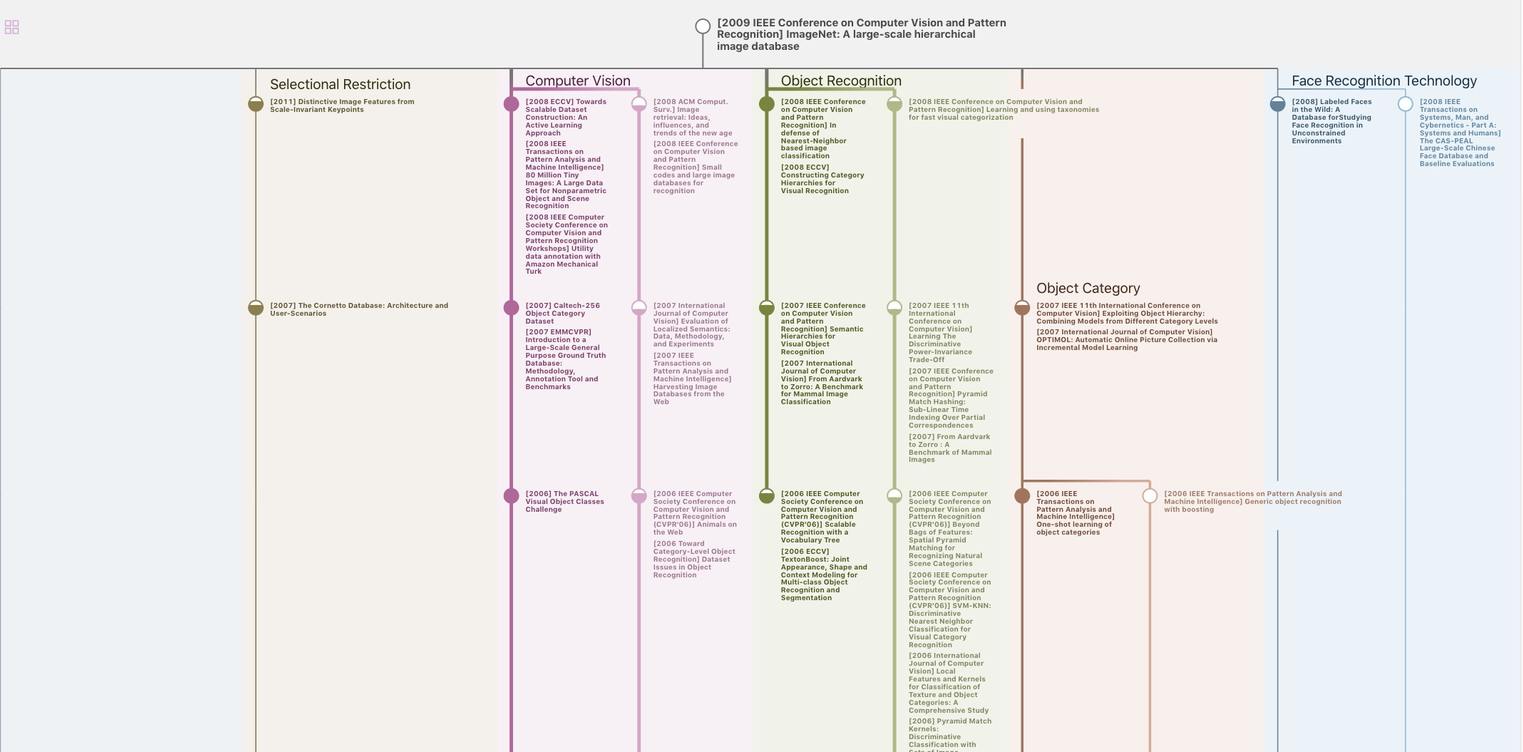
生成溯源树,研究论文发展脉络
Chat Paper
正在生成论文摘要