The PetShop Dataset - Finding Causes of Performance Issues Across Microservices.
CAUSAL LEARNING AND REASONING, VOL 236(2024)
摘要
Identifying root causes for unexpected or undesirable behavior in complexsystems is a prevalent challenge. This issue becomes especially crucial inmodern cloud applications that employ numerous microservices. Although themachine learning and systems research communities have proposed varioustechniques to tackle this problem, there is currently a lack of standardizeddatasets for quantitative benchmarking. Consequently, research groups arecompelled to create their own datasets for experimentation. This paperintroduces a dataset specifically designed for evaluating root cause analysesin microservice-based applications. The dataset encompasses latency, requests,and availability metrics emitted in 5-minute intervals from a distributedapplication. In addition to normal operation metrics, the dataset includes 68injected performance issues, which increase latency and reduce availabilitythroughout the system. We showcase how this dataset can be used to evaluate theaccuracy of a variety of methods spanning different causal and non-causalcharacterisations of the root cause analysis problem. We hope the new dataset,available at https://github.com/amazon-science/petshop-root-cause-analysis/enables further development of techniques in this important area.
更多查看译文
AI 理解论文
溯源树
样例
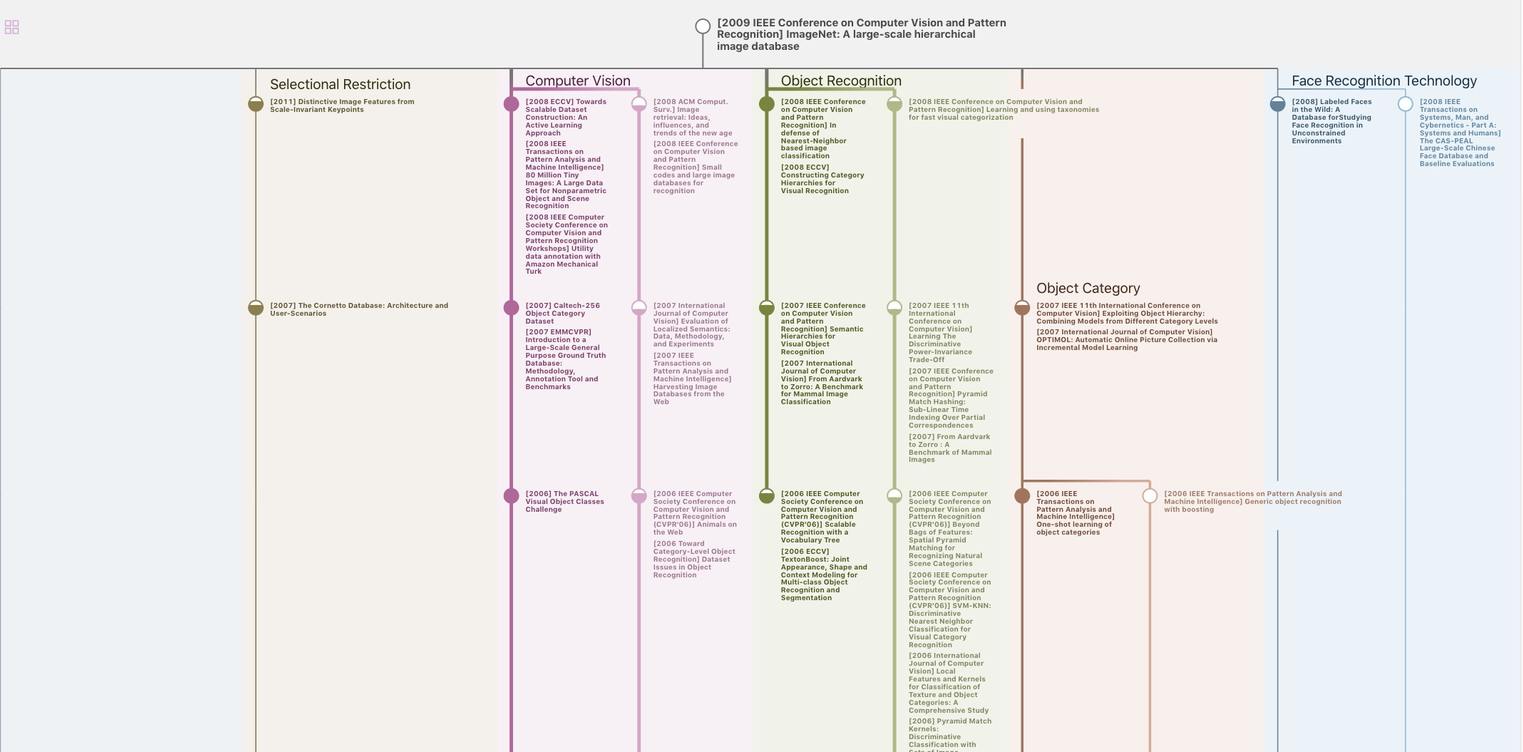
生成溯源树,研究论文发展脉络
Chat Paper
正在生成论文摘要