Pre-training LLMs using human-like development data corpus.
CoRR(2023)
摘要
Pre-trained Large Language Models (LLMs) have shown success in a diverse set
of language inference and understanding tasks. The pre-training stage of LLMs
looks at a large corpus of raw textual data. The BabyLM shared task compares
LLM pre-training to human language acquisition, where the number of tokens seen
by 13-year-old kids is magnitudes smaller than the number of tokens seen by
LLMs. In this work, we pre-train and evaluate LLMs on their ability to learn
contextual word representations using roughly the same number of tokens as seen
by children. We provide a strong set of baselines; with different
architectures, evaluation of changes in performance across epochs, and reported
pre-training metrics for the strict small and strict tracks of the task. We
also try to loosely replicate the RoBERTa baseline given by the task organizers
to observe the training robustness to hyperparameter selection and
replicability. We provide the submission details to the strict and strict-small
tracks in this report.
更多查看译文
关键词
corpus,llms,development,data,pre-training,human-like
AI 理解论文
溯源树
样例
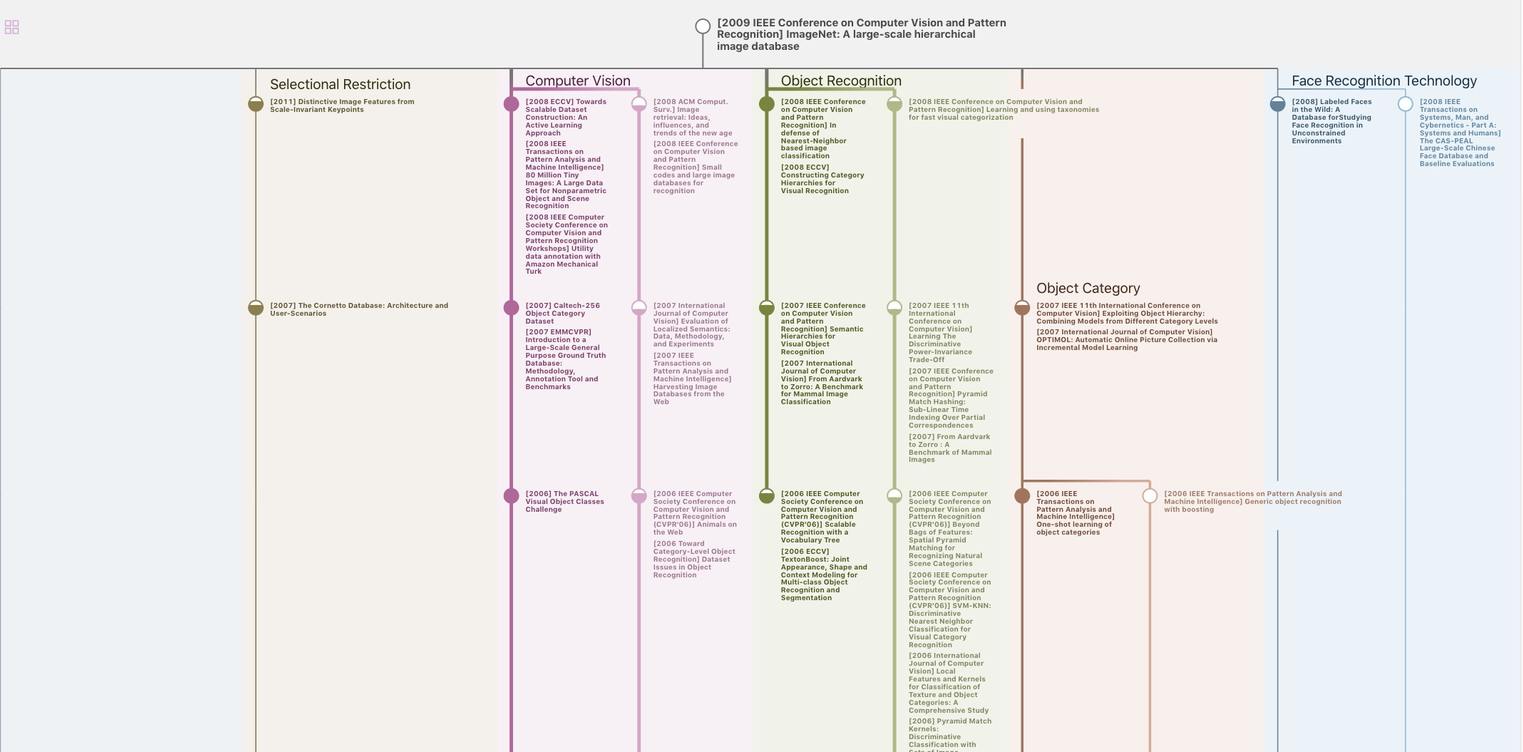
生成溯源树,研究论文发展脉络
Chat Paper
正在生成论文摘要