A Simple Interpretable Transformer for Fine-Grained Image Classification and Analysis
arxiv(2023)
摘要
We present a novel usage of Transformers to make image classification
interpretable. Unlike mainstream classifiers that wait until the last fully
connected layer to incorporate class information to make predictions, we
investigate a proactive approach, asking each class to search for itself in an
image. We realize this idea via a Transformer encoder-decoder inspired by
DEtection TRansformer (DETR). We learn "class-specific" queries (one for each
class) as input to the decoder, enabling each class to localize its patterns in
an image via cross-attention. We name our approach INterpretable TRansformer
(INTR), which is fairly easy to implement and exhibits several compelling
properties. We show that INTR intrinsically encourages each class to attend
distinctively; the cross-attention weights thus provide a faithful
interpretation of the prediction. Interestingly, via "multi-head"
cross-attention, INTR could identify different "attributes" of a class, making
it particularly suitable for fine-grained classification and analysis, which we
demonstrate on eight datasets. Our code and pre-trained models are publicly
accessible at the Imageomics Institute GitHub site:
https://github.com/Imageomics/INTR.
更多查看译文
AI 理解论文
溯源树
样例
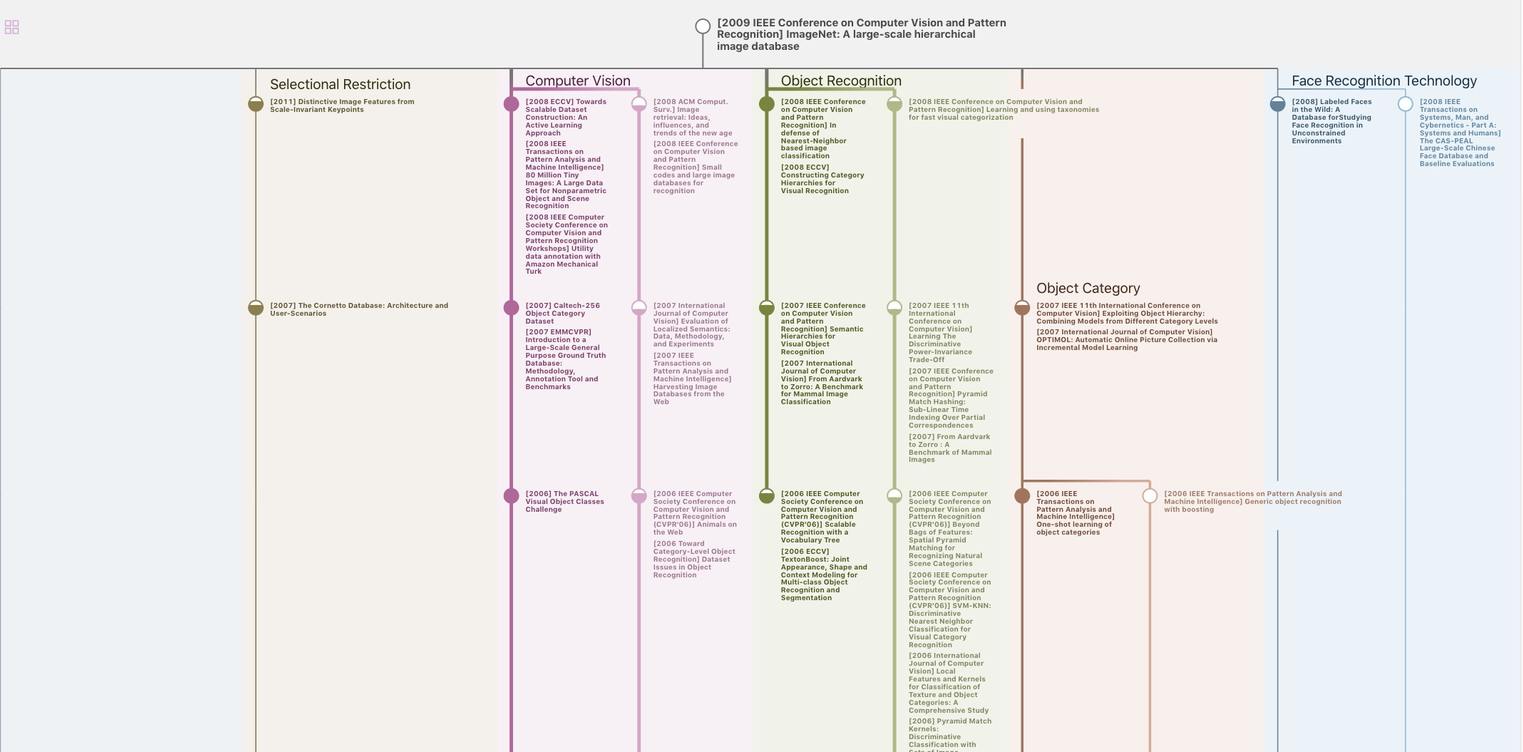
生成溯源树,研究论文发展脉络
Chat Paper
正在生成论文摘要